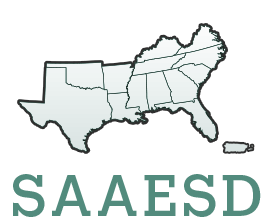
S1090: AI in Agroecosystems: Big Data and Smart Technology-Driven Sustainable Production
(Multistate Research Project)
Status: Active
Date of Annual Report: 08/31/2022
Report Information
Period the Report Covers: 10/01/2021 - 08/05/2022
Participants
Confirmed in-person attendees:1. Yiannis Ampatzidis (Univ. of Florida)
2. Tom Burks (Univ. of Florida)
3. Dana Choi (Univ. of Florida)
4. Thanos Gentimis (Louisiana State Univ.)
5. Zhen Jia (Univ. of Florida)
6. Edward Kick (North Carolina State Univ.)
7. Juan Landivar (Texas A&M Univ.)
8. Daniel Lee (Univ. of Florida)
9. Amando Lopes de Brito Filho (Louisiana State U.)
10. Yuzhen Lu (Mississippi State Univ.)
11. Henry Medeiros (Univ. of Florida)
12. Brenda Ortiz (Auburn Univ.)
13. Luciano Shiratsuchi (Louisiana State Univ.)
14. Alex Thomasson (Mississippi State Univ.)
15. Gary Thompson (University of Arkansas)
16. Jeffrey Vitale (Oklahoma State Univ.)
Confirmed online attendees:
1. Tom Burks (Univ. of Florida, on Friday)
2. Matt Donovan (AgIntel)
3. Hao Gan (Univ. of Tennessee)
4. Steve Thomson (USDA, NIFA)
5. Paul Weckler (Oklahoma State Univ.)
Brief Summary of Minutes
Aug. 4: Field trip
7:45 – 8:00 am Met at the Hilton hotel parking lot and departed at 8 am for field trip.
8:00 – 8:30 am Traveled to PSREU (Citra, FL) (https://plantscienceunit.ifas.ufl.edu/)
8:30 – 9:00 am Dr. Jim Boyer gave a tour of the PSREU facilities including a detailed explanation of the extensive field trials conducted on-site. Our group engaged in an active discussion with Dr. Boyer regarding various agronomic issues and constraints encountered during field trials.
9:00 – 9:50 am Dr. Congliang Zhou provided a precision ag demonstration of a robot programmed to analyze plant wetness using on-board sensors as well to detect soil mites.
9:50 – 10:00 am Break was provided by PSREU at their main deadquarters.
10:00 – 10:30 am Mr. Whitehurst gave a PowerPoint presentation of his 4,000 acres farm/plantation. This included a video of how Mr. Whitehurst uses aerial drones in his ranching operations to herd cattle remotely. He was assisted by Yilin Zhuang and Stacy Strickland. Mr. Whitehrus also explained how data collected from drones is used to mange his extensive plantation.
10:30 – 11:00 am Traveled to the Whitehurst Cattle Farm in Williston, FL.
11:00 – 11:45 am Mr. Whitehurst gave brief farm tour and a presentation of the various drones he owns and operates. This was followed by an in-field demonstration of Mr. Whitehurst herding his cattle using a drone.
11:45 – 12:15 pm Participants returned to Dept. of ABE in Gainesville, FL.
12:15 – 1:00 pm Lunch.
1:00 – 2:00 pm Meeting with Dr. David Reed of the AI2 Center. Dr. Reed dicussed the new AI initiiatives including the hiring of over 100 new faculty dedicated to AI focused positions. Other issues discussed included the SEC Consortium.
2:00 – 3:00 pm Meeting with Dr. Amber Ross, AI ethics expert. Dr. Ross generated a spritied discussion on the ethics of AI use in agriculture and in general throughout society.
3:00 – 3:30 pm Break and travel to HiPerGator supercomputer facilities on the UF campus.
3:30 – 5:00 pm HiPerGator tour was provided by Dr. Erik Deumens. Participants were allowed access into the Hypergator's complex of servers Dr. Deumens explained hoe Hypergator utilizes the CPU computing power of video cards to process computing tasks. participants also viwed the immense cooling faciliies required by HiPerGator.
5:00 - 7:00 pm Dinner and networking at Mildred’s Restaruant. Meal was sponsored by Auburn University.
Aug. 5: Meeting
7:30 – 7:45 am Meet at the Hilton hotel parking lot
7:45 – 8:00 am Drive to ABE Department on the UF campus.
8:00 – 8:10 am Introduction of the participants including Zoom participants.
8:10 – 8:20 am Dr. Gary Thompson, Executive Director, SAAESD, University of Arkansas. Dr. Thompson provided an overview of multi state Hatch projects incuding how to develop and submit annual reports. Dr. Thompson has provided hisPowerPoint.
8:20 – 8:35 am Dr. Damian Adams, S1090 Administrative Advisor, Associate Dean for Research, UF. Dr. Adams streesed the importance of strengthenin AI in the southern region, which lags behind Corn Belt and Western region.
8:35 – 9:10 am Dr. Steve Thomson, USDA-NIFA (video for funding programs, Q&A via zoom). Dr. Thompsen provided a thorough review of cvarious funding oipportuntiies availabel to AI reserach, including fundamental science based research as well as development and implementation.
9:10 – 9:30 am Dr. Shai Sela, Chief Scientist, Agmatix, Ramat Gan, Israel,gave a presentation of his company's AI technology applications.
9:30 – 9:40 am Coffee break.
9:40 – 11:30 am In the first part of this session, participants were grouped into three teams based on area of expertise to encourage team building and future collaboration. In a follow-up session, groups reconvended to discuss plans for the second project year. Consensus was reached to plan for developing a research proposal to be submitted through an agency such as NSF, USDA, etc. A committee was selected to develop a white paper to begin the proposal writing.
11:30 – 1:00 pm This session was a "working lunch" session to take care of several business items such as electiosn, locations of future meetings, annul reporting etc. The following outcomes were achieved:
Business meeting outcomes:
- Elected Yuzhen Lu as our new secretary
- LSU was selected as the 2023 meeting location sometine in May 2023.
- Jeff Vitale was selected to submit the annual report.
1:00 pm Meeting was adjourned by President Daniel Lee circa 1 pm.
Accomplishments
<h1><strong>Activities (2021-2022)</strong></h1><br /> <p> </p><br /> <h2 class="x_MsoNormal"><em>Project Level Activities</em></h2><br /> <p>Members of S1090 project from Auburn University led by Dr. Brenda Ortiz organized a conference targeting undegraduate and young professionals. A total of 250 participants attened in-person and anothe r150 online. the conference was well received and plans are going forward to hold a similar conference next year. Conference details available online: </p><br /> <p class="x_MsoNormal">Website: <a title="Original URL: https://aaes.auburn.edu/ai-driven-innovations-in-agriculture/. Click or tap if you trust this link." href="https://nam04.safelinks.protection.outlook.com/?url=https%3A%2F%2Faaes.auburn.edu%2Fai-driven-innovations-in-agriculture%2F&data=05%7C01%7Cjeffrey.vitale%40okstate.edu%7C83af757bee5a44e01b1208da911e60a1%7C2a69c91de8494e34a230cdf8b27e1964%7C0%7C0%7C637981856910954682%7CUnknown%7CTWFpbGZsb3d8eyJWIjoiMC4wLjAwMDAiLCJQIjoiV2luMzIiLCJBTiI6Ik1haWwiLCJXVCI6Mn0%3D%7C3000%7C%7C%7C&sdata=G6rexetJ8dAa5fFi5oUF%2BjznH1IK0n6oXxhss4vK0H8%3D&reserved=0" target="_blank" rel="noopener noreferrer" data-auth="Verified" data-linkindex="0">https://aaes.auburn.edu/ai-driven-innovations-in-agriculture/</a></p><br /> <p class="x_MsoNormal">Website with conference posters: <a title="Original URL: https://auburncatalog.instructure.com/courses/1860/pages/conference-posters. Click or tap if you trust this link." href="https://nam04.safelinks.protection.outlook.com/?url=https%3A%2F%2Fauburncatalog.instructure.com%2Fcourses%2F1860%2Fpages%2Fconference-posters&data=05%7C01%7Cjeffrey.vitale%40okstate.edu%7C83af757bee5a44e01b1208da911e60a1%7C2a69c91de8494e34a230cdf8b27e1964%7C0%7C0%7C637981856910954682%7CUnknown%7CTWFpbGZsb3d8eyJWIjoiMC4wLjAwMDAiLCJQIjoiV2luMzIiLCJBTiI6Ik1haWwiLCJXVCI6Mn0%3D%7C3000%7C%7C%7C&sdata=hziKrx1lRLlVjn9K4f0r4x7TyFNoOl36PP9uCUoUAKc%3D&reserved=0" target="_blank" rel="noopener noreferrer" data-auth="Verified" data-linkindex="1">https://auburncatalog.instructure.com/courses/1860/pages/conference-posters</a></p><br /> <p class="x_MsoNormal"> </p><br /> <h2 class="x_MsoNormal"><em>State Level Activities</em></h2><br /> <p class="x_MsoNormal"><span style="text-decoration: underline;">Alabama: Auburn University<br /></span></p><br /> <ol><br /> <li>AI-driven high-throughput phenotyping of agronomic and physiological traits in peanut (Yin Bao)</li><br /> </ol><br /> <p>Weekly UAV-based VNIR hyperspectral imagery data were collected for a F<sub>1</sub> peanut population for identifying drought tolerant lines under rainout shelters at the USDA-ARS National Peanut Research Laboratory (NPRL) in Dawson, GA, during pod filling stage in 2021. The project is in collaboration with a peanut breeder (Dr. Charles Chen) and a plant physiologist (Dr. Alvaro Sanz-Saez) from Auburn University and a research chemist (Dr. Phat Dang) from NPRL. Machine and deep learning models were developed to predict three agronomic traits (i.e., pod yield, biomass, and pod count) and two physiological traits (i.e., photosynthesis and stomatal conductance) with reasonable accuracies (R<sup>2 </sup>values around 0.55). A manuscript has been submitted to <em>Remote Sensing</em>.</p><br /> <ol start="2"><br /> <li>AI-based remote sensing of water quality and HABs for inland water bodies in Southeast (Yin Bao)</li><br /> </ol><br /> <p>A dataset including in-situ chlorophyll a and/or microcystin concentration measurements and Sentinel 2 multispectral satellite imagery has been curated for Lake Okeechobee (FL), Lake Thonotosassa (FL), and Lake Seminole (GA) from 2016 to 2021. LSTM models have been trained and tested to forecast chlorophyll a and/or microcystin concentrations in one month ahead using time-series satellite spectral response. Preliminary results are promising but need further improvement. Continued investigation is needed to see if including other features such as weather parameters can improve prediction accuracy.</p><br /> <p>The developed machine and deep learning models for peanut agronomic and physiological traits prediction will enable screening of a large population for drought tolerance by reducing the labor requirement with traditional phenotyping methods, thus accelerating releasing of climate-smart peanut lines for the Southeast.</p><br /> <p> </p><br /> <p class="x_MsoNormal">Peanut Maturity Assessment: Remote sensing and Artificial Intelligence (Brenda Ortiz)</p><br /> <p class="x_MsoNormal">Our research team has begun our work on a project purposed to use remote sensing and AI technology to assist peanut producers in the Southern region in developing improved methods to determine peanut maturity. We are seeking non-desctrucitve methods to assess maturity in cost effective ways to improve peanut harvest and subsequent farm income. </p><br /> <h4> </h4><br /> <p><span style="text-decoration: underline;">Florida: University of Florida</span></p><br /> <p>Uncertainty-aware Robotic Perception Models for Agricultural Production Systems (Dr. Henry Medeiros)</p><br /> <p>Our team developed a self-supervised machine learning model to detect flowers in images of trees acquired in an orchard. Our algorithm makes it possible to detect individual flowers in real-world conditions without the need for specialized data acquisition systems or training data. An evaluation on publicly available benchmark datasets containing images of multiple flower species collected under various environmental conditions demonstrates that our method substantially outperforms existing techniques, despite the fact that it does not need to be trained using images of the target flower species. A manuscript describing our research findings has been submitted to IEEE Robotics and Automation Letters and is currently undergoing its second round of revisions.</p><br /> <p>Expected Impact(s): The self-supervised machine learning model described above enables the development of systems to detect flowers, fruit, buds, and other relevant plant parts in the field without the need to collect and annotate hundreds to thousands of images reflecting all the potential data acquisition scenarios that may impact algorithmic performance, such as illumination variation and image resolution. Data collection and annotation is currently one of the main factors hindering the application of modern artificial intelligence techniques to agricultural problems. Hence, we expect our model to serve as a foundational architecture for the development of future agricultural robotic perception systems.</p><br /> <p> </p><br /> <p>Deep Learning Algorithms (Dr. Dana Choi)</p><br /> <p>A deep learning based algorithm was developed to segment green fruits and fruit stems, then the orientation of the fruits were identified to provide guidance for the robotic green fruit system to remove fruits. A path planning algorithm was also developed with a six-degree-freedom robotic arm to engage targeted green fruits. A series of early apple buds images were acquired with two image acquisition systems, and a YOLOv4 model was developed to detect the buds in the tree canopies.</p><br /> <p>Expected impact(s): Machine vision systems are being utilized extensively in agriculture applications. Daytime imaging in outdoor field conditions presents challenges such as variable lighting and color inconsistencies due to sunlight. Motion blur can occur due to vehicle movement and vibrations from ground terrain. A camera system with active lighting can be a solution to overcome these challenges. The developed on-tree apple fruit sizing system with high-resolution stereo cameras and artificial lighting increased performance of fruit sizing compared to manual inspection. Apple fruit size plays an integral role in orchard management decision-making, particularly during chemical thinning, fruit quality assessment, and yield prediction. UAV-based systems for thermal and RGB imaging with machine vision algorithms demonstrated the feasibility of the orchard heating requirement determination methodology, which has the potential to be a critical component of an autonomous, precise frost management system in future studies.</p><br /> <p> </p><br /> <p>Fruit Based AI Technology (Dr. Daniel Lee)</p><br /> <p>Our team accomplished the following over the past reporting year:</p><br /> <ul style="list-style-type: circle;"><br /> <li>Strawberry plant wetness detection system has been developed using color imaging and deep learning for strawberry production. Based on the 2021-22 results, a portable wetness sensor will be designed for use in commercial strawberry fields.</li><br /> <li>Smartphone-based tool was developed to detect and count two-spotted spider mites (TSSM) on strawberry plants. Various deep learning methods were used to detect TSSM, eggs, and predatory mites. A portable six-camera sensor device was developed and is currently being tested for detecting TSSM in strawberry and almond leaves.</li><br /> <li>Strawberry bruise and size detection systems for postharvest fruit quality evaluation were developed utilizing machine vision and deep learning. These systems can be used in strawberry packinghouses.</li><br /> </ul><br /> <p>Expected impact(s): The plant wetness detection system could enhance the performance of the disease prediction models for strawberry growers in Florida and other parts of the US. The TSSM detection device and tool will increase the efficiency of pest management and thereby increase strawberry yield and profit. The device could be used for other row crops affected by TSSM. The strawberry bruise and size detection system could improve the quality of strawberries.</p><br /> <p> </p><br /> <p>Computer Algorithms for Machine Vision Applications in Agriculture (Yiannis Ampatzidis)<strong><br /></strong></p><br /> <p>Over the reporting year our team accompllshed the following: </p><br /> <ul style="list-style-type: circle;"><br /> <li>Strawberry bruise detection system for postharvest fruit quality evaluation was developed utilizing machine vision and deep learning.</li><br /> <li>Disease detection and monitoring system was developed for downy mildew in watermelons utilizing UAV-based hyperspectral imaging and machine learning. This technique was able to classify several severity stages of the disease.</li><br /> <li>Yield and related traits prediction system was developed for wheat under heat-related stress environments. This high-throughput system utilizes UAV-based hyperspectral imaging and machine learning. A yield prediction system was developed for citrus too utilizing UAV-based multispectral imaging and AI.</li><br /> <li>System was developed to determine leaf stomatal properties in citrus trees utilizing machine vision and artificial intelligence.</li><br /> <li>Machine vision based system was developed to measure pecan nut growth utilizing deep learning for a better understanding of the fruit growth curve.</li><br /> </ul><br /> <p> </p><br /> <p><span style="text-decoration: underline;">Kentucky (University of Kentucky)</span></p><br /> <p>Non-Destructive Testing of Fruit in Fod Processing and Manufacturing (<strong>Akinbode A. Adedeji)</strong></p><br /> <p>Over the reporting year the following was accompished:</p><br /> <ul style="list-style-type: circle;"><br /> <li>Fundamental understanding of the state-of-the-art in area that relates to the application of nondestructive (NDT) approach (Intelligent sensors) to food (meat, surfaces, and apples) quality evaluation by writing review papers on the subject and published them in high impact journals.</li><br /> <li>Advanced the understanding of application of two sensing methods for qualitative and qualitative assessment of apples and millet cultivars.</li><br /> <li>Developed hyperspectral imaging (HSI) and vibro-acoustic methods for nondestructive testing of apple for codling moth pest. The classification results were well above 90% in both cases for test-set results.</li><br /> </ul><br /> <p> </p><br /> <p>Expected impact(s): The timely publication of review papers provides a succinct summary of current state of knowledge in these areas that is a resource for many of our colleagues. One of the papers has seen double digit citation in less than a year. Also, some of the results from our work on nondestructive method developments will form the foundation for the application of sensing methods in artificial systems (robotics) development for implementation in the apple and meat processing industries.</p><br /> <p> </p><br /> <p>Machine Learning Applications in Grape Production (<strong>Carlos M. Rodriguez Lopez)</strong></p><br /> <p>Over the reporting year the following was accompished:</p><br /> <ul style="list-style-type: circle;"><br /> <li><strong>Predicting continent and country of origin of vineyard soil samples:</strong> We tested the efficiency of 9 different Machine Learning models (i.e., Random Forest, AdaBoost, Bernouli Naïve Bayes, Gradient Boosting Machine, Gausian Naïve Bayes, k-NN(k=5), k-NN(k=10), SVM, and Neural Network) to predict the origin of soil samples using freely available next generation sequencing 16S data from 233 vineyards planted in 5 countries (Australia (n=32), Spain (n=86), Denmark (n=15), Germany (n=10), and USA (n=63)), distributed within 3 different continents (Australia (n=32), Europe (n=138), and North America (n=63)). The accuracy of the tested models to predict the country of origin varied between 63% and 92% obtained by the Bernouli Naïve Bayes and the Neural Network models respectively. As expected, continent prediction was slightly higher and varied between 69% and 94% obtained by the k-NN(k=10) and the Neural Network models respectively.</li><br /> </ul><br /> <ul style="list-style-type: circle;"><br /> <li><strong>Planted genotype prediction using microbiome data from vineyard soil samples:</strong> The same ML models enumerated above were used to predict the planted grapevine genotype (cultivar) using freely available next generation sequencing 16S data from 177 soil samples from vineyards planted with 7 different cultivars in 9 different countries (Cabernet Sauvignon,(n=65; planted in Australia, Spain, South Africa, and USA), Tempranillo (n=60; planted in Spain), Syrah/Shiraz (n=12; planted in Australia, Italy, Spain, and South Africa), Chardonnay (n=12; planted in Argentina and USA), Pinot Noir (n=10; planted in Croacia and USA), Riesling (n=10; planted in Germany) and Solaris (n=10; planted in Denmark). The accuracy of the tested models to predict the country of origin varied between 63% and 81% obtained by the the Bernouli Naïve Bayes and the Neural Network models respectively. All models however showed high levels of variability in their prediction accuracy. We hypothesize that this is due to data imbalance due to the disparity on the number of data sets between cultivars. To test this hypothesis, we will use synthetic and real datasets generated in house.</li><br /> </ul><br /> <p> </p><br /> <p>Expected impact(s): The quality of grapes used for wine production has been traditionally associated to the concept of Terroir. This concept captures the interaction between the cultivated grapevine variety and the complete natural environment in which a particular wine is produced, including the soil, topography, climate, and the viticultural and oenological practices used to manage the vineyard and during wine production respectively. Recent studies (e.g. Zhou et al. 2021) show that the composition, diversity and function of soil bacterial communities play important roles in determining wine quality which can indirectly affect its economic value. Two of the main drivers of soil bacterial community composition in vineyards are the environmental conditions (Zhou et al. 2021), and the planted grapevine cultivar, suggesting that terroir is not a unidirectional vector, but a feed-back loop between the original soil microbial communities, the vineyard environment, and the planted cultivar. Understanding how the environment and the plant genotype interact to alter the soil microbial communities, is therefore of paramount importance for the elucidation of the elusive concept of terroir.</p><br /> <p> </p><br /> <p><span style="text-decoration: underline;">Mississippi: Mississippi State U.</span></p><br /> <p>AI Appications in Cotton and Fruit Crops (Alex Thomasson)</p><br /> <p>Over the reporting year our team accompllshed the following: </p><br /> <ul style="list-style-type: circle;"><br /> <li>Generation of big data sets:<br /> <ul><br /> <li>Collected and processed hundreds of soil samples from benchmark soil series in Mississippi in summer 2022. These samples are being scanned to collect spectra in order to create a dataset that will be used to develop AI-based soil carbon estimations. (Dr. Nuwan Wijewardane)</li><br /> <li class="x_xxmsolistparagraph">Collected and processed hundreds of images of weeds that are common competitors in cotton crops. These images are being used to develop AI models that can enable real-time detection of weeds for spot spraying in cotton crops. (Dr. Yuzhen Lu)</li><br /> </ul><br /> </li><br /> <li>Development of AI-based models for natural resources applications:<br /> <ul><br /> <li class="x_xxmsolistparagraph">Used AI on multisource data to forecast groundwater levels in the Mississippi River Valley Alluvial Aquifer. (Drs. Joel Paz and Mary Love Tagert)</li><br /> </ul><br /> </li><br /> </ul><br /> <ul style="list-style-type: circle;"><br /> <li>Development of AI for image-based detection and classification in the following applications:<br /> <ul><br /> <li class="x_xxmsolistparagraph">Cranberry fruit at different maturity levels for soft robotic harvesting. (Dr. Xin Zhang)</li><br /> <li class="x_xxmsolistparagraph">Treatment of herbicide-resistant weeds in real time, directing an automated tillage implement to reduce herbicide usage and prevent unnecessary soil moisture loss that occur with whole-field tillage. (Dr. Wes Lowe)</li><br /> <li class="x_xxmsolistparagraph">Separate plastic contaminants from cotton fiber. (Filip To)</li><br /> <li class="x_xxmsolistparagraph">Locate cotton bolls on plants for robotic harvesting. (Dr. <span class="markrfaqfn884" data-markjs="true" data-ogac="" data-ogab="" data-ogsc="" data-ogsb="">Alex</span> Thomasson)</li><br /> <li class="x_xxmsolistparagraph">Predict the yield of cotton plants from early-season multisource data including drones. (Dr. <span class="markrfaqfn884" data-markjs="true" data-ogac="" data-ogab="" data-ogsc="" data-ogsb="">Alex</span> Thomasson)</li><br /> </ul><br /> </li><br /> <ul type="circle"><br /> <li class="x_xxmsolistparagraph">Platic rubbish in cotton fields before harvest, with data from drones. (Dr. <span class="markrfaqfn884" data-markjs="true" data-ogac="" data-ogab="" data-ogsc="" data-ogsb="">Alex</span> Thomasson)</li><br /> <li class="x_xxmsolistparagraph">Volunteer cotton plants in corn and sorghum fields. (Dr. <span class="markrfaqfn884" data-markjs="true" data-ogac="" data-ogab="" data-ogsc="" data-ogsb="">Alex</span> Thomasson)</li><br /> </ul><br /> </ul><br /> <p>Expected impact(s): Our research is expected to generate impacts from three major areas in the development and application of AI in agriculture:</p><br /> <ol><br /> <li>Generation of big data sets and the enhanced informed decision making capacity that will be unfold.</li><br /> <li>Modeling for natural resource applications to provide stakeholders with more refined, accurate, and wider scoped information from whcich policy and business decisions can be undertaken.</li><br /> <li>The marked advancements in detection and classification of images expanded to new applications will continue to be adopted by producers as a practical tool for real-time automated decision-making and applications. For example, we have shown that AI can be used for real-time detection of (a) contaminants in cotton fiber, (b) cotton bolls for robotic harvesting, (c) plastic rubbish in cotton fields that can contaminate cotton fiber, and (d) cotton plants that have germinated from seed left in fields at harvest during the prior season, which can serve as a host for pernicious insects. Such improved detection will generate greater productivity and enhanced profits for producers in the Southern region. </li><br /> </ol><br /> <p> </p><br /> <p><span style="text-decoration: underline;">North Carolina: North Carolina State (Edward Kick) </span></p><br /> <p>Our research accompished the foillowing over the past reporting year:</p><br /> <ul style="list-style-type: circle;"><br /> <li>Collected and merged large data sets from published sources such as World Bank, United Nations, Fa. Circa 50 variables were coded using R and Python into an Excel file. 3. Data set was cleaned using R and SPSS, as missing data were searched for missing data that were located, coded, and cleaned using R.</li><br /> <li>Descriptive data analysis was undertaken to identify miscodes, means, standard deviations, etc. Data transformations were applied as necessary in log transformations for skewed data. Replacement data as needed were located, coded, and cleaned in a new access data file.</li><br /> <li>A total of 120 hours of regression analyses were undertaken under the program “structural equation modeling” (SEM) using AMOS. SEM permits tests of hypothesized linkages among all variables, thereby showing the strength of a series of direct and indirect effects. This helps the researchers avoid multicollinearity, which otherwise would compromise the estimations and essentially provide inaccurate inferences. Preliminary results indicate that industrial agriculture results in unsatisfactory consequences for food production. The blind faith that accompanies its usage is seriously questioned for the 134 nations examined. 10. Results and conclusions are published in an agricultural journal. The next set of even more sophisticated results is under examination and very likely to be published in the Swiss journal, Sustainability. These findings are corroborated by Carolan (2016: 112-115).</li><br /> <li>Preliminary literature review of artificial intelligence and agriculture literature undertaken for one section of our recently supported Multistate request.</li><br /> </ul><br /> <p>Expected impact(s): There are said to be four or five nations in the world that use the model of agricultural production that guided much of the Green Revolution (GR). GR is lauded for substantially increasing production of essential agricultural products such as wheat, which helped millions of starving persons in the middle 1900s. However, meta-analyses clearly show the many negative impacts of agricultural production on communities. Our intensive investigation of 134 countries further shows that industrial agriculture has not improved undernourishment in the modern world, and in fact, it has contributed substantially to the degradation of our global environment through production and release. Eco-agricultural farming promises to be a superior alternative, particularly when it is carefully coupled with artificial intelligence. We plan to investigate the attitudes of farming communities, those with a substantial component of farming as we once knew it, to ascertain their views of both Eco-agriculture as explained by us, and artificial intelligence. We have already gathered the base data on communities in every corner of the United States. We have begun to analyze the BIG DATA, which will guide us in the selection of communities for examination.</p><br /> <p> </p><br /> <p><span style="text-decoration: underline;">Oklahoma: Oklahoma State (Brian Arnall, Tyson Ochsner, Jason Warren, and Jeff Vitale)</span></p><br /> <p>Research over the past reporting year achived the following:</p><br /> <ul style="list-style-type: circle;"><br /> <li>Developed and impmentted an AI algorthm on a data set of winter wheat from nitrogen studies which have been continued for 15 years. Data includes yield, protein, NDVI, soil characteristics, and mesonet wheat and soil data. Results generate yield and various plant growth characteristics. </li><br /> <li>Developed a machine learning model to predict the movement of sugar cane aphid on oklahoma sorghum fields.</li><br /> <li>Developed and applied neural network models for nondestructive estimation of aboveground biomass in rangelands and for high resolution mapping of soil moisture across heterogenous land covers.</li><br /> <li>Aerial sensor data collected on wheat field trials at the Perkins Experiment Station </li><br /> </ul><br /> <p> </p><br /> <p> </p><br /> <p> </p><br /> <p><span style="text-decoration: underline;">South Carolina: Clemson University</span></p><br /> <p>Our research over the reporting year completed two successive years of hyperspectral data collection of peach leaves at one of Clemson Research Centers – Piedmont Research and Education Center. The data collection started in 2020 and continued until late 2021. Before the pandemic, our team and collaborators from CSIC, Spain collected hyperspectral images at the same peach orchards to determine the effects of Silicon applications on a peach tree and water stress. Young, full-sized leaves with petioles attached were picked for the trees with high and medium K concentrations (45 leaves), while 50 were selected for the peach trees with low K concentrations due to their size. The center research staff working on the same plot designated the plots for high, medium, and low K concentration. The leaves were collected from the midpoint near the base of each tree. The mature leaf samples were then grouped into low, mid, and high K. A snapshot hyperspectral camera was used to scan each leaf of each group before sending it to the Agricultural Services Laboratory for a plant tissue analysis. The spectral data were preprocessed using a calibration panel. Four pretreatment methods (Multiplicative Scatter Effect, Savitzky-Golay first derivative, Savitzky-Golay second derivative and Standard Normal Variate) were applied to the calibrated raw data and partial least square (PLS) was used to develop a model for each pretreatment.</p><br /> <p>Expected impact(s): The impact of the K prediction project on the peach tree is twofold, 1 -helps determine the spectral signature where K can be predicted, and 2—use of pretreatment methods significantly improves the development of PLS models. The results of this work open the possibility of developing a more miniature detector of K, which only uses the essential bands. It will also facilitate the development of sensors specific to K detection which will be cheaper for farmers to use.</p><br /> <p> </p><br /> <p><span style="text-decoration: underline;">Tennessee: University of Tennessee</span></p><br /> <p>The work conducted by Tennessee focused on the development of AI technology for the improvement of livestock and poultry health and welfare. Our research team developed and evaluated ancamera system in the lab and in the broiler farm for automated gait score assessment. The outcome of this project is an automated tool that helps broiler farmers to identify lameness in broilers early. It provides timely information for broiler farmers to improve their farm management practices for better animal welfare and higher production.multiple research and commercial broiler farms in the U.S.</p><br /> <p>Expected impact(s): In this project, a computer vision system was developed to provide an automated assessment of broiler gait scores in commercial farms. The system was low cost, required minimum maintenance, and was designed to be used for most commercial broiler farms. The potential impact of the research is to provide farmers with an automated tool for accurate and timely broiler welfare evaluation. It will lead to improvements in farm management, thus, improvements in animal welfare and health. It will eventually help improve animal productions and will also bring in economic benefits to US agriculture and food systems.</p><br /> <p> </p><br /> <p> </p><br /> <p><span style="text-decoration: underline;">Texas: Texas A&M (Juan Landivar)</span></p><br /> <p>1. Crop Phenotyping: Cotton and Wheat</p><br /> <p>Cotton: We developed an Unmanned Aircraft System (UAS) based High Throughput Phenotyping (HTP) pipeline that can measure temporal growth and spatial development parameters for cotton. The system includes a big data management system (CottonHub) which facilitates UAS data management (search, upload, and download), generates geospatial data products for visualization purposes, extracts plant growth features, and communicates with users and cooperators. The system includes tools to perform growth analysis of the experimental units or genotypes and extract approximately twelve growth parameters depicting the characteristics and performance of the genotypes in field conditions.</p><br /> <p>Wheat: We are participating in a wheat CAP grant as part of a team of 19 wheat programs in the USA. Our work involves developing a standardized UAS data collection protocol for high-quality data collection, training the WheatCAP team members in UAS data collection, management/processing of the UAS data collected by the national wheat breeding programs, providing visualization tools to the WheatCAP team members, and extracting plant growth features to select elite germplasm.</p><br /> <p>Expected impact(s):</p><br /> <p>The phenotyping systems described above are used by cotton and wheat breeders to manage, display, and analyze phenotypic features of experimental genotypes. The system is included in the NSF grant led by Drs. Hombing Zhang, Wayne Smith, and Steve Hague (Texas A&M University, cotton Breeders), and other breeders from across the Cotton Belt. The cotton UASHub has the potential to save cotton breeders as much as 60% of the cost of collecting phenotype and yield data from field plots. Similarly, the WheatCAPHub (https://wheatcap.uashubs.com) is part of a USDA-NIFA grant (funded, $15M), led by Dr. Amir Ibrahim (Texas A&M Wheat Breeder). Our contribution has the potential of similar cost reductions to the wheat breeder, in addition to facilitating communication among scientists from across the country.</p><br /> <p> </p><br /> <p>2. Testbed Data Management and Uses</p><br /> <p>We completed four years (2019 to 2022) of UAS data collection in a large (approximately 100 acres) commercial cotton field located in Driscoll, Texas as a testbed. RGB and multispectral data were collected on a weekly basis from UAS. The collected data were processed to generate fine spatial and high temporal resolution orthomosaic and Digital Surface Models (DSM). The testbed fields were divided into grids of 10 x 10 m (100 m2) which resulted in a total of approximately 4,000 grids within the testbed. Plant features extracted from each grid include: Canopy Cover (%), Plant Height (m), Canopy Volume (m3), and Vegetative Indexes (Excess Greenness Index and NDVI). Seedcotton yield was obtained from the yield monitoring system of the cotton harvester. The data was used to develop Digital Twin models for in-season crop management and to obtain an early-season estimation of cotton yield for marketing purposes.</p><br /> <p>Expected impact(s): The digital twin system developed from the testbed data described above was used to estimate crop termination time and defoliant rates during the 2021 and 2022 seasons. Canopy Cover data was used to create management zones for the precision application of defoliants. The system accurately estimated the time of defoliation as early as one month before the event. The digital twin model estimated cottonseed yield within 5% of the actual yield approximately a month before harvest. It is expected that the prescription crop management package along with in-season yield forecasting capabilities developed from the testbed data would optimize production cost, yield, and fiber quality. Having earlier and more accurate forecasts of pre-harvest yield would be extremely useful in facilitating forward selling from growers to merchant buyers. Thus, a reduction in production cost along with enhanced marketing strategies could enhance the profitability of cotton production by approximately 10%.</p><br /> <p>3. Extension & Outreach</p><br /> <p>Cotton cultivar tests are crucial educational activities of research and extension agronomists, but their establishment, maintenance, and data collection are time-consuming and costly. Although the information generated is valuable, the field plots are seldom visited by producers. This project proposes to bring the field plots to producers via a web-based platform designed to analyze and visualize the growth characteristics of cultivars and to make comparisons between entries. A cotton cultivar data management web-based Hub (CultivarHub) was developed to facilitate the communication between extension personnel (crop specialists and county agents) with producers.</p><br /> <p>Expected impact(s):</p><br /> <p>The impact of the CultivarHub is twofold; (1) help cotton specialist manage, analyze, and summarize data from cultivar, agrochemicals, or irrigation evaluation trials, and (2) facilitate the communication and educational outreach of extension specialist, county agents, or IPM agents with producers. It is expected that this web-based platform can increase the outreach and technical communication efficiency of extension personnel by 60%.</p><br /> <p> Extension presentation(s):</p><br /> <p>Landivar J, Mahendra Bhandari, Josh McGinty, Murilo Maeda, Jose Landivar, Hend Alkittawi, Anjin Chang, Daniel Gonzales. 2022. UAS-Based Platform for Evaluating the Performance of Cotton Cultivars for Research and Outreach. 2022 Beltwide Cotton Conferences, San Antonio, Texas. January 7, 2022.</p><br /> <p> </p><br /> <p> </p>Publications
<p>Alabama: Aubrun University </p><br /> <p>Citation of the conference proceedings paper: Oliveira, M.F., F.M. Carneiro, M. Thurmond, M.D. Del val, L.P. Oliveira, B. Ortiz, A. Sanz-saez, D. Tedesco. 2022. Predicting Below and Above Ground Peanut Biomass and Maturity Using Multi-target Regression. In Proceedings of the 2022 International Conference of Precision Agriculture. June 26-29, 2022 Minneapolis.</p><br /> <p> </p><br /> <p>Florida: University of Florida</p><br /> <p>Yuan, W., Choi, D., Bolkas, D., Heinemann, P.H. and He, L., 2022. Sensitivity examination of YOLOv4 regarding test image distortion and training dataset attribute for apple flower bud classification. International Journal of Remote Sensing, 43(8), pp.3106-3130.</p><br /> <p>Yuan, W., Choi, D., & Bolkas, D. (2022). GNSS-IMU-assisted colored ICP for UAV-LiDAR point cloud registration of peach trees. Computers and Electronics in Agriculture, 197, 106966.</p><br /> <p>Zahid, A., Mahmud, M.S., He, L., Schupp, J., Choi, D. and Heinemann, P., 2022. An Apple Tree Branch Pruning Analysis. HortTechnology, 32(2), pp.90-98.</p><br /> <p>Zhang, H., He, L., Di Gioia, F., Choi, D., Elia, A. and Heinemann, P., 2022. LoRaWAN based internet of things (IoT) system for precision irrigation in plasticulture fresh-market tomato. Smart Agricultural Technology, 2, p.100053.</p><br /> <p>Mahmud, M.S., Zahid, A., He, L., Choi, D., Krawczyk, G. and Zhu, H., 2021. LiDAR-sensed tree canopy correction in uneven terrain conditions using a sensor fusion approach for precision sprayers. Computers and Electronics in Agriculture, 191, p.106565.</p><br /> <p>Patel, A., W. S. Lee, N. A. Peres, and C. W. Fraisse. 2021. Strawberry plant wetness detection using computer vision and deep learning. Smart Agricultural Technology 1, 2021, 100013, ISSN 2772-3755, https://doi.org/10.1016/j.atech.2021.100013</p><br /> <p>Yun, C., H.-J. Kim, C.-W. Jeon, M. Gang, W. S. Lee, and J. G. Han. 2021. Stereovision-based ridge-furrow detection and tracking for auto-guided cultivator. Computers and Electronics in Agriculture 191, 2021, 106490, ISSN 0168-1699, https://doi.org/10.1016/j.compag.2021.106490.</p><br /> <p>Puranik, P., W. S. Lee, N. Peres, F. Wu, A. Abd-Elrahman, and S. Agehara. 2021. Strawberry flower and fruit detection using deep learning for developing yield prediction models. In the Proceedings of the 13th European Conference on Precision Agriculture (ECPA), July 19-22, 2021, Budapest, Hungary.</p><br /> <p>Zhou, X., W. S. Lee, Y. Ampatzidis, Y. Chen, N. Peres, and C. Fraisse. 2021. Strawberry maturity classification from UAV and near-ground imaging using deep learning. Smart Agricultural Technology 1, 2021, 100001, ISSN 2772-3755, https://doi.org/10.1016/j.atech.2021.10000</p><br /> <p>Costa L., McBreen J., Ampatzidis Y., Guo J., Reisi Gahrooei M., Babar A., 2021. Using UAV-based hyperspectral imaging and functional regression to assist in predicting grain yield and related traits in wheat under heat-related stress environments for the purpose of stable yielding genotypes. Precision Agriculture, 23 (2), 622-642.</p><br /> <p>Costa L., Ampatzidis Y., Rohla C., Maness N., Cheary B., Zhang L., 2021. Measuring pecan nut growth utilizing machine vision and deep learning for the better understanding of the fruit growth curve. Computers and Electronics in Agriculture, 181, 105964, <a href="https://doi.org/10.1016/j.compag.2020.105964">doi.org/10.1016/j.compag.2020.105964</a>.</p><br /> <p>Costa L., Archer L., Ampatzidis Y., Casteluci L., Caurin G.A.P., Albrecht U., 2021. Determining leaf stomatal properties in citrus trees utilizing machine vision and artificial intelligence. Precision Agriculture 22, 1107-1119, <a href="https://doi.org/10.1007/s11119-020-09771-x">https://doi.org/10.1007/s11119-020-09771-x</a>.</p><br /> <p>Nunes L., Ampatzidis Y., Costa L., Wallau M., 2021. Horse foraging behavior detection using sound recognition techniques and artificial intelligence. Computers and Electronics in Agriculture, 183, 106080, <a href="https://doi.org/10.1016/j.compag.2021.106080">doi.org/10.1016/j.compag.2021.106080</a>.</p><br /> <p>Vijayakumar V., Costa L., Ampatzidis Y., 2021. Prediction of citrus yield with AI using ground-based fruit detection and UAV imagery. 2021 Virtual ASABE Annual International Meeting, July 11-14, 2021, 2100493, doi:10.13031/aim.202100493.</p><br /> <p>Zhou, C., W. S. Lee, O. E. Liburd, I. Aygun, J. K. Schueller, and I. Ampatzidis. 2021. Smartphone-based tool for two-spotted spider mite detection in strawberry. ASABE Paper No. 2100558. St. Joseph, MI.: ASABE.</p><br /> <p>Zhou, X., Y. Ampatzidis, W. S. Lee, and S. Agehara. 2021. Postharvest strawberry bruise detection using deep learning. ASABE Paper No. 2100458. St. Joseph, MI.: ASABE.</p><br /> <p>Influence of Planting Date, Maturity Group, and Harvest Timing on Soybean (Glycine max (L.) Yield and Seed Quality, PRISCILA CAMPOS, DONNIE MILLER, JOSH COPES, MELANIE NETTERVILLE, SEBE BROWN, TREY PRICE, DAVID MOSELEY, THANOS GENTIMIS, PETERS EGBEDI, RASEL PARVEJ3 (Accepted by Crop, Forage, & Turfgrass Management, Summer 2022). In this paper, modern methodologies were implemented in the analysis of the results, as well as more traditional statistical techniques.</p><br /> <p>The Time of Day Is Key to Discriminate Cultivars of Sugarcane upon Imagery Data from Unmanned Aerial Vehicle, BARBOSA JÚNIOR, M.R.; TEDESCO, D.; CARREIRA, V.S.; PINTO, A.A.; MOREIRA, B.R.A.; SHIRATSUCHI, L.S.; ZERBATO, C.; SILVA, R.P., Drones 2022, 6, 112. https://doi.org/10.3390/drones6050112</p><br /> <p>UAVs to Monitor and Manage Sugarcane: Integrative Review, BARBOSA JÚNIOR, M.R.; MOREIRA, B.R.A.; BRITO FILHO, A.L.; TEDESCO, D.; SHIRATSUCHI, L.S.; SILVA, R.P., Agronomy 2022, 12, 661. https://doi.org/10.3390/agronomy12030661</p><br /> <p>Predicting Days to Maturity, Plant Height, and Grain Yield in Soybean: A Machine and Deep Learning Approach Using Multispectral Data, TEODORO, P. E.; TEODORO, L. P. R.; BAIO, F. H. R.; SILVA JUNIOR, C. A.; SANTOS, R. G.; RAMOS, A. P. M.; PINHEIRO, M. M. F.; OSCO, L. P.; GONCALVES, W. N.; CARNEIRO, A. M.; MARCATO JUNIOR, J.; PISTORI, H.; SHIRATSUCHI, L. S., Remote Sensing, v. 13, p. 4632, 2021</p><br /> <p>Comparison of Machine Learning Techniques in Cotton Yield Prediction Using Satellite Remote Sensing, MORELLI-FERREIRA, F.; MAIA, N.J.C.; TEDESCO, D.; KAZAMA, E.H.; MORLIN CARNEIRO, F.; SANTOS, L.B.; SEBEN JUNIOR, G.F.; ROLIM, G.S.; SHIRATSUCHI, L.S.; SILVA, R.P. Preprints 2021, 2021120138 (doi: 10.20944/preprints202112.0138.v2). Published and in preparation for Remote Sensing.</p><br /> <p> </p><br /> <p> </p><br /> <p>Kentucky: University of Kentucky</p><br /> <p>Ekramirad, N., Khaled, Y.A., Doyle, L., Loeb, J., Donohue, K.D., Villanueva, R., and <strong>Adedeji, A.A.</strong> (2022). Nondestructive detection of codling moth infestation in apples using pixel-based NIR hyperspectral imaging with machine learning and feature selection. <em>Foods</em> 11(8), 1 - 16. (Citation: 2)</p><br /> <p>Rady, A., Watson, N., and <strong>Adedeji, A.A. </strong>(2021). Color imaging and machine learning for adulteration detection in minced meat. <em>Journal of Agriculture and Food Research </em>6(100251), 1-11. (Citation: 1)</p><br /> <p>Watson, N.J., Bowler, A.L., Rady, A., Fisher, O.J., Simeone, A., Escrig, J., Woolley, E., and <strong>Adedeji, A.A</strong>. (2021). Intelligent sensors for sustainable food and drink manufacturing. <em>Frontiers in Sustainable Food Systems.</em> 5, 642786. (Citation: 5)</p><br /> <p>Ekramirad, N., Al Khaled, Y.A., Donohue, K., Villanueva, R., Parrish, C.A., and <strong>Adedeji, A</strong>. (2021). Development of pattern recognition and classification models for the detection of vibro-acoustic emissions from codling moth infested apples. <em>Postharvest Biology and Technology </em>181, 111633. (Citation: 1)</p><br /> <p>Khaled, Y.A., Parrish, C. and *<strong>Adedeji, A.A</strong>. (2021). Emerging nondestructive approaches for meat quality and safety evaluation. <em>Comprehensive Reviews in Food Science and Food Safety. </em>20(4): 3438-3463. (Citation: 15)</p><br /> <p> </p><br /> <p>Mississppi: Mississippi State University</p><br /> <p>Chen, D., Lu, Y., Li, Z., and Young, S. 2022. Performance evaluation of deep transfer learning on multi-class identification of common weed species in cotton production systems. <em>Computers and Electronics in Agriculture</em>.</p><br /> <p>Yadav, P.K., Thomasson, J.A., Hardin, R.G., Searcy, S.W., Braga-Neto, U., Popescu, S.C., Martin, D.E., Rodriguez III, R., Meza, K., Enciso, J. and Solorzano, J. 2022. Volunteer cotton plant detection in corn field with deep learning. In Proc. <em>Autonomous Air and Ground Sensing Systems for Agricultural Optimization and Phenotyping VII</em> (Vol. 12114, pp. 15-22). SPIE.</p><br /> <p> </p><br /> <p>North Carolina: NC State </p><br /> <p>Edward L. Kick, Laura McKinney, Steve McDonald, Andrew Jorgenson. “A Multiple-Network Analysis of the World System of Nations” is printed in Chinese and is used in what we hope will be a forthcoming publication in Sustainability.” That paper introduces the possibility of using artificial intelligence in farming around the world.</p><br /> <p>Edward L. Kick, Gregory Fulkerson, and Ahad Pezeshkpoor. “Agriculture Grains, and Beef Production: Remedies for food for Food Insecurity and the Ecological Footprint When the Cataclysm Comes?” Agricultural Research and Technology 23: 53-57.</p><br /> <p>Edward L. Kick “Cross-National Empirical Studies of Sustainability, Agriculture and the Environment: Cumulating Forward or Erring in an About Face?” Agricultural Research and Technology 25: 601-603.</p><br /> <p>Edward L. Kick. “Taking a World View”. College of Agriculture and Life Sciences Newsletter.</p><br /> <p>Edward L. Kick and Ahad Pezeshkpoor. “Biomes, World-System Positions, and National Characteristics as linked Precursors to Global Undernourishment and the Ecological Footprint” Under revision for publication in Sustainability.</p><br /> <p> </p><br /> <p> </p><br /> <p>South Carolina: Clemson University</p><br /> <p>Abenina MIA, Maja JM, Cutulle M, Melgar JC, Liu H. Prediction of Potassium in Peach Leaves Using Hyperspectral Imaging and Multivariate Analysis.</p><br /> <p>AgriEngineering.2022;4(2):400-413. https://doi.org/10.3390/agriengineering4020027</p><br /> <p class="x_MsoNormal"> </p><br /> <p class="x_MsoNormal">Tennessee; University of Tennessee</p><br /> <p>Nasiri, A., Yoder, J., Zhao, Y., Hawkins, S., Prado, M., & Gan, H. (2022). Pose estimation-based lameness recognition in broiler using CNN-LSTM network. Computers and Electronics in Agriculture, 197, 106931.</p><br /> <p> </p><br /> <p>Texas: Texas A&M</p><br /> <p>Bhandari, M.; Baker, S.; Rudd, J. C.; Ibrahim, A. M. H.; Chang, A.; Xue, Q.; Jung, J.; Landivar, J.; Auvermann, B. Assessing the Effect of Drought on Winter Wheat Growth Using Unmanned Aerial System (Uas)-Based Phenotyping. Remote Sens. 2021, 13 (6). https://doi.org/10.3390/rs13061144.</p><br /> <p>W. Wu, S. Hague, J. Jung, A. Ashapure, M. Maeda, A. Maeda, A. Chang, D. Jones, J.A. Thomasson, J. Landivar, "Cotton row spacing and Unmanned Aerial Vehicle sensors," Agronomy Journal, https://doi.org/10.1002/agj2.20902, 2021</p><br /> <p>A. Chang, J. Jung, J. Landivar, J. Landivar, B. Barker, R. Ghosh, "Performance evaluation of parallel structure from motion (SfM) processing with public cloud computing and an on-premise cluster system for UAS images in agriculture," International Journal of Geo-Information, 10, 677, https://doi.org/10.3390/ijgi10100677, 2021</p><br /> <p>S. Oh, A. Chang, A. Ashapure, J. Jung, N. Dube, M. Maeda, D. Gonzalez, J. Landivar, "Plant Counting of Cotton from UAS Imagery Using Deep Learning-Based Object Detection Framework", Remote Sensing, 12(18):2981, DOI: 10.3390/rs12182981, 2020</p><br /> <p>M. Bhandari, A. Ibrahim, Q. Xue, J. Jung, A. Chang, J. Rudd, M. Maeda, N. Rajan, H. Neely, J. Landivar, "Assessing winter wheat foliage disease severity using aerial imagery acquired from small Unmanned Aerial Vehicle (UAV)", Computers and Electronics in Agriculture, 176:105665, DOI: 10.1016/j.compag.2020.105665, 2020</p><br /> <p>Landivar J., J. Jung, A. Ashapure, M. Bhandari, M. Maeda, J. Landivar, A. Chang and D. Gonzalez. 2021. In-Season Management of Cotton Crops Using “Digital Twins” Models. ASA-CSSA-SSSA International Annual Meeting, Salt Lake City, UT, November 9-11, 2021.</p><br /> <p>Landivar J, M. Maeda, A. Chang, J. Jung, J. McGinty, C. Bednarz, 2021. "Estimating the time and rate of harvest aid chemicals using an Unmanned Aircraft System," 2021 Beltwide Cotton Conferences, Online Conference, January 5 - 7, 2021</p><br /> <p>Ashapure A., J. Jung, A. Chang, S. Oh, J. Yeom, M. Maeda, A. Maeda, N. Dube, J. Landivar, S. Hague, W. Smith, 2020. "Developing a machine learning based cotton yield estimation framework using multi-temporal UAS data", ISPRS Journal of Photogrammetry and Remote Sensing, vol. 169, pp. 180-194.</p><br /> <p>J. Jung, M. Maeda, A. Chang, M. Bhandari, A. Ashapure, J. Landivar, "The potential of remote sensing and artificial intelligence as tools to improve the resilience of agriculture production systems", Current Opinion in Biotechnology, vol. 70, pp. 15-22, 2021</p><br /> <p> </p><br /> <p> </p>Impact Statements
- Our first year of project activities has had positive impacts on Southern regional agriculture through a diverse range of AI activities as detailed in the Accomplishments section of this report. Our accomplishments include advances in row crop production, fruit production and harvesting, and in food processing and manufacturing.
Date of Annual Report: 07/24/2023
Report Information
Period the Report Covers: 09/01/2022 - 04/17/2023
Participants
Please see attached belowBrief Summary of Minutes
Accomplishments
Publications
Impact Statements
Date of Annual Report: 09/05/2023
Report Information
Period the Report Covers: 09/01/2022 - 01/01/2023
Participants
Brief Summary of Minutes
Duirng the meeting, we had the opportunity to discuss various collaborative efforts, that culminated to a google document spreadsheet with multiple participants "buying in to various projects"
The first day of the conference was dedicated to showcasing the use of AI in agriculture to a specialty crop that is important for Louisiana (sugarcane). The participants had the opportunity to visit the John Deere factory at Thibodaux and discuss cutting edge technology initiatives with the technical lead team there. The participants also got to tour the facility on site. The second stop was the LSU Ag Center Sugarcane Station, where the participants got to see first hand the use of AI to improve the Sugarcane Breeding program in Louisiana. The team there presented us with the whole process, and showed how an AI powered app is fundamental in their new breeding scheduling.
The second day of the conference was dedicated to creating possible collaborations, both for common papers and common grant writting.