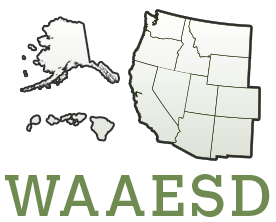
W3009: Integrated Systems Research and Development in Automation and Sensors for Sustainability of Specialty Crops
(Multistate Research Project)
Status: Inactive/Terminating
Date of Annual Report: 10/04/2019
Report Information
Period the Report Covers: 10/01/2018 - 09/30/2019
Participants
Cheng Zhongyang, Auburn UniversityIrwin Gonzalez, University of California, Davis
Joseph Dvorak, University of Kentucky
Ning Wang, Oklahoma State University
Daeun Choi, Pennsylvania State University
Paul Heineman, Pennsylvania State University
Long He, Pennsylvania State University
Alex Thomasson, Texas A&M University
Qin Zhang, Washington State University
Karen Lewis, Washington State University
Brief Summary of Minutes
The meeting started with a tour of the University of Kentucky Horticulture Research Farm at 8am where the discussion focused on the development of diversified vegetable production to help in the transition away from tobacco production. The farm is on the site of an historic hemp plantation, and at 9am there was an introduction to the history of hemp production in Kentucky and its recent resurgence. At 10am, the group toured Elmwood Stock Farm which is a diversified vegetable and livestock farm that primarily produces for Community Supported Agriculture (CSA) and farmers’ markets. After lunch the group traveled to the University of Kentucky’s Spindletop Research Farm to see various hemp production trial plots for fiber/grain/CBD production. The group then toured the Zelios CBD-processing facility to understand how the hemp is handled post-harvest and how the CBD oil is extracted. The day finished with a tour at Kenneth Anderson’s farm, which has shifted from tobacco production to hemp but while using the tobacco transplant growing model. Discussion focused on crop characteristics and harvest.
The business meeting began at 8am on Friday, September 6th, in the C.E. Barnhart Building on the University of Kentucky campus. At the beginning of the meeting, a trainer provided an impact writing workshop to help researchers improve their ability to communicate the broader impacts of their research. After a presentation, there was an opportunity to practice writing. The workshop was followed by the business meeting. The first item of business was to select the location for the next meeting. It will be hosted by Washington State University and be in the Tri-cities area. There was a desire to adjust the format of the meeting to increase the meeting to a full two days. The meeting will still include demonstration of local advances in specialty crop production and the business meeting. Additionally, it will include a Brainstorming session and Roadmap planning workshop so that we can identify the key challenges and opportunities in specialty crop automation for the next several years. For tours, the intention is to include demonstrations of the newly commercialized robotic apple picking machines. Joe Dvorak requested that each station provide brief items for inclusion in the annual report within two weeks. Dana Choi of Pennsylvania State University was selected as the next secretary (nominated by Irwin, Alex seconded, all were in favor). Long He transitioned to Vice Chair, and Irwin transitioned to the Chair. This was followed by station reports which continued through lunch.
Highlights of station reports:
Washington State University – Qin Zhang
- Smart irrigation system development
- Robotic weed control
- Targeted apple harvesting (shake-and-catch harvesting, robotic picking)
- Crop load estimate using cellphone
- Robotic pruning research
- Blossom thinning research
- Green shoot thinning for grapevines
- UAS bird deterrence
- SSCDS- Solid spay system for tree orchards
- Steam treatment for citrus HLB
- Field Phenomics for variety selection
- Fire blight assessment for apple trees
- Potato/onion rot detection
- Preventing frost damage for fruit crops
Auburn University – Zhongyang Cheng
- Sensor development (Real-time detection of bacteria/spores)
- With the capability of direct detection on fresh surface, detection from extra-low concentration, and in-Field detection
- Sensor for agriculture
Water/Humidity sensors (extra low cost), and soil elements mapping
UC Davis – Irwin Donis-Gonzalez
- Handheld spectrophotometer (F-750)
- Working with breeding/genetics for tomato and pepper.
- Non-Constructive characteristics of structure changes in vitro gastric digestion of apples using 3D time series micro computed tomography (Micro-CT)
Texas A & M – Alex Thomasson
- UAV Image data calibration
- Fixed wings UAV, using manned aircraft for ground truth
- Plant height calibration
- 3D canopy model estimation
- Thermal calibration tiles
Penn State – Daeun Choi
- Fruit detection for yield estimation using depth image
- Mushroom harvester
- Maize root phenotyping system
- Sensor based irrigation system for specialty crops
- Robotic branch pruning system for apple trees
- Frost protection using UAV and UGV
Oklahoma State University – Ning Wang
- Developing phenotyping tools for peanut breeders
- Canopy architecture
- Flower counts
- Stress indicators
- Developing sensing and control technologies for pecans and processors
- X-ray imaging for detecting peanut smut disease and automated system for peanut smut detection.
University of Kentucky – Joe Dvorak
- UAV imaging for Alfa-Alfa (NDF, ADF, CP, Yield model estimation)
- Future: Precision navigation in specialty crops
The station reports and discussion on the reports were concluded and the business meeting adjourned at 12:30pm.
Accomplishments
<h3>Arizona</h3><br /> <p>A high speed, centimeter scale resolution sprayer that can spot apply herbicides to weeds while traveling at speeds that are viable for commercial farming operations was developed and tested. Results showed that targeting accuracy of spray delivered was ± 2 mm and that the percentage of off-target spray was less than 3%. Weed control efficacy exceeded 95% and there was no observable crop injury. Results were presented at the 2019 ASABE Annual International Meeting.</p><br /> <h3>California</h3><br /> <p><strong>Objective 3: Study interactions between machinery and crops</strong></p><br /> <p>A small fruit-catching system that utilizes inflatable tubes was developed for shake-catch harvesting. The effect of inflated tubes on fruit damage was tested in pear and cling-peach orchards. Fruits hanging up to 1 ft above the tubes were dropped on the tubes. Results showed no significant difference between dropped fruits and fruits collected manually, as control.</p><br /> <p><strong>Objective 5: Design and evaluate automation systems</strong></p><br /> <p>A harvest-aid orchard platform was modified so that pickers on the right side of the platform can work at variable heights from the ground. Actuation was performed via hydraulic cylinders under computer control. The platform’s speed was controlled with a computer-controlled hydraulic valve that modulated flow to the hydraulic motors. A stereo camera system in the front of the platform detected apples, and two instrumented picking bags measured each picker’s harvesting rate. An online optimizing controller varied each picker’s working height and platform speed, with the objective of maximizing the machine’s harvesting throughput. Experiments in a Fuji apple orchard block, in Lodi CA, showed that the machine’s harvesting throughput increased by an average of 9% over the throughput when pickers worked at fixed heights, which is the current situation.</p><br /> <p>To address the challenge of quantifying Plant water Status (PWS) in a convenient manner, a continuous sensor suite (- leaf monitor) was developed at UC Davis to measure leaf temperature and other microclimatic variables (i.e., photosynthetically active radiation (PAR), relative humidity, air temperature, and wind speed) and subsequently tested in almond, walnut, and grape crops. Filed tests were conducted in an almond orchard located in Arbuckle, CA using a wireless network of leaf monitors and controllers to implement precision irrigation. The analysis of the data indicated significant water savings without impacting yield or harvest quality. Compared to evapotranspiration estimates and soil moisture based methods used by grower, this plant-sensing irrigation scheme used an average of 70% and 83% of water, respectively. Moreover, water productivity was significantly higher for the plant stress-based (1870 ± 190 lb/acre-ft) treatment compared to grower treatment (2110 ± 300 lb/acre-ft). In addition, a commercial version of these leaf monitors were installed and monitored throughout the 2018 growing season in a vineyard as well as in walnut and pistachio orchards. In these vineyard and orchards, growers implemented their conventional irrigation practices and the leaf monitors were used to track PWS. The system has performed well during the whole season and additional tests are being conducted during 2019 growing season.</p><br /> <h3>Connecticut</h3><br /> <p>A multi-disciplinary team at the University of Connecticut began work to develop a monitoring system for insect pest damage using small unmanned aerial systems (UAS) outfitted with spectral sensors. The project objective is to detect the presence and early plant damage caused by the potato leafhopper <em>Empoasca fabae</em> on beans. Initial steps are underway and primarily deal with documenting the leaf spectral responses generated during leafhopper feeding in a controlled setting. Another insect under investigation is the cabbage aphid <em>Brevicoryne brassicae</em>. The plant spectral data collected so far were used in a preliminary analysis of spectral indices suggested in the literature for detection of plant stress. Further work will follow up on these results.</p><br /> <h3>Florida</h3><br /> <p>An automated imaging system was developed to count the number of flowers and fruit in the strawberry field using artificial intelligence. Maps for flower distribution and estimated fruit yield were created to help growers for more efficient harvesting operations. Algorithms for detecting strawberry plant wetness were developed using color and thermal imaging. The results will be used for the Strawberry Advisory System which provides real-time fungicide recommendations to strawberry growers. </p><br /> <h3><strong>Iowa</strong></h3><br /> <p>Model and analyzed the effect of the tines of a mechanical weeder on soil and simulated plant disturbance. </p><br /> <p>Investigated the current state of art in robotic weed management and control systems and wrote a book chapter that is now distributed globally.</p><br /> <p>Analyzed data from spray drift experiments and found patterns in the likelihood of drift volumes with different wind velocity and distance into off-target regions.</p><br /> <p>Complete the robotic rover development for Enviratron facility for plant performance research under different environmental conditions. Designed and prototyped a field-based plant phenotyping robot for genomics research.</p><br /> <h3>Kentucky</h3><br /> <p>A UAV-based photogrammetry method to create a 3D model of the plant canopy has been developed for alfalfa. This 3D model is being used to estimate nutritive value and yield in this forage crop. Testing has also been performed in lettuce to determine if the procedure will be useful to predict lettuce yield as well.</p><br /> <h3>Oklahoma</h3><br /> <p>The practice of hand-opening pods for rating disease is a major bottleneck in breeding for peanut smut resistance. This method is so slow that disease ratings for one season are often not completed until seed for the next season is being planted. Healthy peanut pods filled with seed are denser than infected pods filled with teliospores of <em>Thecaphora frezzii. </em>In 2018-19, we have been evaluating alternative, efficient methods for screening peanut smut in the U.S. using faux-infected pods. The methods included X-ray imaging, gravity separation, microwave dielectric sensors, microwave resonant cavity, and audible sensors. The x-ray imaging method showed an advantage on detecting damaged seed(s) in pod. </p><br /> <h3>Pennsylvania</h3><br /> <p>The RootRobot for plant excavation and phenotyping was fabricated and components were lab tested.</p><br /> <p>A sensor-based irrigation system using soil-moisture, evapotranspiration, and plant stress was installed and tested in apple orchards.</p><br /> <p>A robotic pruning end-effector with two rotational mechanism was developed for apple trees.</p><br /> <p>A machine vision system for early season apple yield estimation was developed.</p><br /> <p>Prototypes of automatic mushroom harvester were developed and tested. The system consisted of a vision system for maturity and size evaluation and an end-effector for pulling crops from production bed.</p><br /> <h3>Washington</h3><br /> <p>WA team has worked on multiple aspects of sensing and automation technologies, including robotic devices, crop sensing systems, advanced spraying technologies, for supporting more effective specialty crop, from vegetables, grapevines, to fruit tree, productions. The accomplishments in the past year including (but not limited to): (1) developed and integrated a vision-based fruit orientation estimation and obstacle avoidance system to a 12-armed robotic apple harvester, and evaluated in commercial orchards environment; (2) improved and further evaluated targeted shake-and-catch fresh-market harvesting system and reached a 90% or higher fruit removal efficiency with 10% or less fruit damage in formally trained orchards for selected apple varieties; (3) modified the self-leveling mechanism and improved the vision weed detection system of a self-propelling weeding robot and further evaluated it on commercial carrot and onion fields. This improved machine system achieved a 99% or higher weed detection accuracy and 2 mm or less spraying accuracy while travelling on uneven natural terrain; (4) conceptualized an automated green shoot thinning mechanism for grapevines using a deep-learning-based machine vision system for detecting vine cordon and shoots and developed a trajectory fitting model to represent cordon position and orientation which reached a 80% cordon trajectory estimation; (5) further optimized a solid set chemical delivery system (SSCDS) for vineyards and orchards applications through creating a reservoir sub-system to maintain the pressure for achieving more precise application control and reducing drift losses to the air; (6) developed an Internet-of-Things (IoT) enabled Crop Physiology Sensing System (CPSS) through encompassing a thermal-RGB imager integrated with a single board computer for monitoring apple fruit surface temperature and controlling SSCDS performing automated evaporative cooling; and (7) conducted a preliminary field trial of spraying cellulose nanocrystal (CNC) for frost protection at two commercial orchards in 2019 Spring, and found it could increase bud cold hardness by up to 5 °C and did not observe any adverse impact on trees nor fruits.</p><br /> <h3>Activities in Support of Accomplishments</h3><br /> <p>Collaborated with a provide equipment manufacturer (FFRobotics) in developing a 12-armed full-scale robotic apple harvesting system (with the main contribution on designing the visual-based fruit detection and obstacle avoidance system), and participated in field evaluations in commercial orchards. </p><br /> <p>Developed a new self-propelled research prototype of multi-layer shake-and-catch harvesting system fresh market apples and evaluated the new prototype in commercial orchards in 2018 harvest season. </p><br /> <p>Modified an improved full-scale research prototype of self-propelled robotic weeding machine based on lessons learned from field tests of the previous prototype. This new machine was successfully tested on natural terrain commercial vegetable fields in 2019 weeding season. </p><br /> <p>Another robotic machinery development activity was the improvement of a mechanized red raspberry cane bundling and tying device, focused on design, fabricate and field evaluation of an innovative cane tying mechanism. </p><br /> <p>Initiated the project of SMART IRRIGATION, including setup test blocks in a research vineyard, developing research platform(s) for field data collection and bigdata processing. This project was aimed to optimize water use in wine grape using big data analytics. </p><br /> <p>Continued the development of machine vision systems for automating green shoot thinning on grapevines; and extensively conducted in laboratory evaluation for tuning the developed deep learning algorithm for image processing. </p><br /> <p>Continued working on developing sensing and automation technologies based on both ground platforms and UASs, including for rapid quantifying and/or evaluating plant biotic and abiotic stressors using hyperspectral imaging and associated data analytics methods. The team has also evaluated the applicability of small-UAS-based multi-spectral sensing modules to monitor retrofitted or modified irrigation techniques.</p><br /> <p>Developed and evaluated an Internet-of-Things enabled Crop Physiology Sensing System (CPSS) for tree fruit crop loss management with initial focus on apple sunburn management.</p><br /> <p>Developed and evaluated a prototype horticultural oil thermotherapy system for pear psylla management.</p><br /> <h3>West Virginia</h3><br /> <p>Dr. Tabb gave five talks at industry groups, academic departments in horticulture and computer science, and gave a webinar on camera calibration for the American Society of Plant Biologists. Through these talks, she shared her research on shape estimation for automating orchard work and phenotyping. She also participated in some community initiatives, talking about research in agricultural robotics.</p><br /> <p>Automation and phenotyping for tree fruit. E&J Gallo precision agriculture group. Modesto, California. September 10, 2019.</p><br /> <p>Autonomously generating shape estimates of plant parts across scales. Phenome 2019. Tucson, Arizona. February 7, 2019.</p><br /> <p>Transforming Pixels to Millimeters: Geometric Camera Calibration. Plantae webinar series, American Society of Plant Biologists. November 29, 2018.</p><br /> <p>Autonomous shape phenotyping of trees: strategies using computer vision and robotics. Michigan State University Horticulture Department. East Lansing, Michigan. September 13, 2018.</p><br /> <p>Estimating plant shape in field settings. University of Minnesota Computer Science and Engineering colloquium. Minneapolis, Minnesota. September 10, 2018.</p><br /> <p>Panellist: sustainable agriculture at Sweet Briar College, April 23, 2019.</p><br /> <p>Panellist: Farms 2 Schools, WVU extension service, June 19, 2019.</p>Publications
<h3>Arizona</h3><br /> <p>Raja, R., Slaughter, D.C., Fennimore, S.A., Nguyen, T.T., Vuong, V.L., Sinha, N., Tourte, L., Smith, R.F. & Siemens, M.C. 2019. Crop signaling: A novel crop recognition technique for robotic weed control. Biosystems Eng. (submitted)</p><br /> <p>Govindaraj. D.K., Zhu, L., Siemens, M.C., Nolte, K.D., Brassill, N., Rios, D.V., Galvez, R., Fonseca, J.M. & Ravishankar, S. 2018. Modified Coring Tool Designs Reduce Iceberg Lettuce Cross-Contamination. J. Food Protection. 82(3): 454-462. DOI: 10.4315/0362-028X.JFP-18-317.</p><br /> <p>Lefcourt, A.M., Siemens, M.C. & Rivadeneira, P. 2018. Optical parameters for using visible-wavelength reflectance or fluorescence imaging to detect bird excrements in produce fields. Appl. Sci., 9(4), [715]; DOI:10.3390/app9040715.</p><br /> <p>Everard, C.D., Kim, M.S., Siemens, M.C., Cho, H., Lefcourt, A.M. & O’Donnell, C. 2018. A multispectral imaging system using solar illumination to distinguish fecal matter on leafy greens and soils. Biosystems Eng. 171: 258-264.</p><br /> <h3>California</h3><br /> <p>Vougioukas, S.G. (2019). Agricultural Robotics. Annual Review of Control, Robotics, and Autonomous Systems. 2:365-392. https://doi.org/10.1146/annurev-control-053018-023617</p><br /> <p>Charlton, D., Edward Taylor, j. E., Vougioukas, S.G. (2019). Can Wages Rise Quickly Enough to Keep Workers in the Fields? Choices, 2nd Quarter 34(2). http://www.choicesmagazine.org/choices-magazine/submitted-articles/can-wages-rise-quickly-enough-to-keep-workers-in-the-fields</p><br /> <p>Charlton, D., Edward Taylor, J.E., Vougioukas, S.G., Rutledge, Z. (2019). Innovations for a Shrinking Agricultural Workforce. Choices, 2nd Quarter 34(2). http://www.choicesmagazine.org/choices-magazine/submitted-articles/estimating-value-damages-and-remedies-when-farm-data-are-misappropriated/innovations-for-a-shrinking-agricultural-workforce</p><br /> <p>Peng, C., Vougioukas, S.G. (2019). Scheduling performance of harvest-aiding crop-transport robots under varying earliness in access to transport-request predictions. Accepted. ASABE Annual International Meeting. Boston, Massachusetts.</p><br /> <p>Kizer, E., S. K. Upadhyaya, C. Ko-Madden, F. Rojo, K. Drechsler, and J. Meyers.2018. Good to the last drop-Getting the most out of precision irrigation. Progressive Crop Consultant. May/June: 20,22,24-26.</p><br /> <p>Bazzi, C.L., K. Schenatto, S. K. Upadhyaya, F. Rojo, E. Kizer, and C. Ko-madden. 2019. Optimal placement of proximal sensors for precision irrigation in tree crops. J. Precision Ag. 20(4):663-674.</p><br /> <p>Dhillon, R., F. Rojo., S. K. Upadhyaya, J. Roach, R. Coates, and M. Delwiche. 2019. Prediction of plant water status in almond and walnut trees using a continous leaf monitoring system. Precision Ag. 20(4):723-745.</p><br /> <p>Bazzi, C. L., K. Schenatto, S. Upadhyaya and F. Rojo. 2018. Optimal placement of proximal sensors for precision irrigation for in tree crops. Proceedings of the 14th International Conference on Precision Agriculture, Montreal, Canada. 8pp.</p><br /> <p>Drechsler, K., I. Kisekka, and S. Upadhyaya. 2018. A comprehensive stress index for evaluating water stress in almond trees. Proceedings of the 14th International Conference on Precision Agriculture, Montreal, Canada. 9pp.</p><br /> <p>Ko-Madden, C. T. 2018 Optimal placement of minimal number of proximal sensors for precision irrigation management. Unpublished MS thesis, Biological and Agricultural Engineering Department, University of California Davis, 145pp.</p><br /> <p>Kizer, E. E. 2018. A precision irrigation scheme to manage plant water status using leaf monitors in almonds. Unpublished MS thesis, Biological and Agricultural Engineering Department, University of California Davis. 116pp. </p><br /> <h3>Florida</h3><br /> <p>Chen, Y., W. S. Lee, H. Gan, N. Peres, C. Fraisse, Y. Zhang, and Y. He. 2019. Strawberry yield prediction based on a deep neural network using high-resolution aerial orthoimages. Remote Sensing, 11: 1584. Doi:10.3390/rs11131584.</p><br /> <p>Lin, P., W. S. Lee, Y. M. Chen, N. Peres, and C. Fraisse. 2019. A deep-level region-based visual representation architecture for detecting strawberry flowers in an outdoor field. Precision Agriculture. Published online: 07 June 2019. https://doi.org/10.1007/s11119-019-09673-7. </p><br /> <h3>Iowa</h3><br /> <h3>Articles</h3><br /> <p>Kshetri, S., B. L. Steward, J.J. Jiken, L. Tang, and M. Tekeste. 2019. Investigating effects of interaction of single tine and rotating tine mechanism with soil on weeding performance using simulated weeds. Transactions of the ASABE. doi: 10.13031/trans.13301</p><br /> <p>Schramm, M. W., H. M. Hanna, M. J. Darr, and S. J. Hoff, and B. L. Steward. 2019. Sub-second wind velocity changes one meter above the ground. Applied Engineering in Agriculture. doi: 10.13031/aea.12264.</p><br /> <p>Zhang, W., J. Gai, L. Tang, Y. Ding, Q. Liao. 2019. Double-DQN-based path smoothing and tracking control method for in-field robotic vehicle navigation. <em>Computers and Electronics in Agriculture</em>. DOI: 10.1016/j.compag.2019.104985.</p><br /> <p>Tu, X., J. Gai, L. Tang. 2019. Robust navigation control of a 4WD/4WS agricultural robotic vehicle. <em>Computers and Electronics in Agriculture </em>164. DOI: 10.1016/j.compag.2019.104892</p><br /> <p>Gai, J., L. Tang, B. L. Steward. 2019. Automated crop plant detection based on the fusion of color and depth images for robotic weed control. <em>Journal of Field Robotics </em>2019: 1-18. DOI: 10.1002/rob.21897.</p><br /> <p>Xiang, L., Y. Bao, L. Tang, D. Ortiz, M. G. Salas-Fernandez. 2019. Automated morphological traits extraction for sorghum plants via 3D point cloud data analysis. <em>Computers and Electronics in Agriculture</em> 162: 951-961. DOI: 10.1016/j.compag.2019.05.043.</p><br /> <p>Bao, Y., L. Tang, S. Srinivasan, P. S. Schnable. 2018. Plant architectural traits characterization for maize using time-of-flight 3D imaging. <em>Biosystems Engineering</em> 178: 86-101. DOI: 10.1016/j.biosystemseng.2018.11.005.</p><br /> <h3>Book Chapter</h3><br /> <p>Steward, B. L., J. Gai, and L. Tang. 2019. The use of agricultural robots in weed management and control. In <em>Robotics and Automation for Improving Agriculture.</em> ed. J. Billingsley. Burleigh Dodds Science Publishing: Cambridge, UK.</p><br /> <h3>Conference Paper</h3><br /> <p>Steward, B. L., H. M. Hanna, P. M. Dixon, R. K. Mompremier. 2019. Measuring and modeling the movement of spray droplets into off-target areas. ASABE Paper No. 1901496. St. Joseph, Mich.: ASABE. DOI: doi.org/10.13031/aim.201901496</p><br /> <h3>Pennsylvania </h3><br /> <p>Shi, X., Choi, D., Heinemann, P., Lynch, J., and Hanlon, M. 2019. RootRobot: A field-based platform for maize root system architecture phenotyping. ASABE Paper No. 1900806. American Society of Agricultural and Biological Engineers. 6 pp.</p><br /> <p>He, L., Zeng, L., and Choi, D. 2019. Investigation of sensor-based irrigation systems for apple orchards. NABEC Paper <em>No. 19-013</em>. American Society of Agricultural and Biological Engineers. ASABE: St. Joseph, MI. <em> </em></p><br /> <p>Zahid, A., He, L. and Zeng, L. 2019. Development of a Robotic End Effector for Apple Tree Pruning. ASABE Paper <em>No. 1900964</em>. American Society of Agricultural and Biological Engineers. ASABE: St. Joseph, MI. <em> </em></p><br /> <p>He, L., Zhang, X., Ye, Y., Karkee, M., and Zhang, Q. 2019. Effect of shaking location and duration on mechanical harvesting of fresh market apples. Applied Engineering in Agriculture, <em>35</em>(2), 175-183.</p><br /> <p>Feng, J., Zeng, L., and He, L. 2019. Apple fruit recognition algorithm based on multi-spectral dynamic image analysis. <em>Sensors, 19</em>(4), p. 949.</p><br /> <p>Lee, C., Choi, D., Pecchia, J., He, L., & Heinemann, P. 2019. Development of A Mushroom Harvesting Assistance System using Computer Vision. 2019 ASABE Annual International Meeting, Paper No. 190050, page 1-5, July 7 – July 10, 2019.</p><br /> <p>Jarvinen, T., Choi, D., Heinemann, P., Schupp, J., & Baugher, T. A. 2019. Tree trunk position estimation for accurate fruit counts in apple yield mapping2019 ASABE Annual International Meeting, Paper No. 1900918, page 1-7, July 7 – July 10, 2019.</p><br /> <p>Shi, Xiaomeng., Choi, D., Heinemann, P., Lynch, J., & Hanlon, M. 2019. RootRobot: A Field-based Platform for Maize Root System Architecture Phenotyping. 2019 ASABE Annual International Meeting, Paper No.1900806, page 1-7, July 7 – July 10, 2019.</p><br /> <p>Jarvinen, T., Choi, D., Heinemann, P., & Baugher, T. A. 2018. Multiple object tracking-by-detection for apple fruit counting on a tree canopy. 2018 ASABE Annual International Meeting, Paper No. 1801193, page 1-8, July 29 – Aug 1, 2018.</p><br /> <p>Choi, D., & Jarvinen, T. 2018. "A video processing strategy using camera movement estimation for apple yield forecasting." Proceedings of the 9th International Symposium on Machinery and Mechatronics for Agriculture and Biosystems Engineering, page 1-5, Jeju, South Korea, May 28-30, 2018.</p><br /> <p>Wang, C., Lee, W. S., Zou, X., Choi, D., Gan, H., & Diamond, J. 2018. Detection and counting of immature green citrus fruit based on the Local Binary Patterns (LBP) feature using illumination-normalized images. Precision Agriculture. ISBN/ISSN #/Case #/DOI #: https://doi.org/10.1007/s11119-018-9574-5. Online publication.</p><br /> <h3>Washington</h3><br /> <p>Bhusal, S., K. Khanal, S. Goel, M. Karkee, and M. Taylor. 2019. Bird deterrence in a vineyard using an unmanned aerial system (UAS). <em>Transactions of the ASABE;</em> 62(2): 561-569 (doi: 10.13031/trans.12923).</p><br /> <p>Chakraborty, M., L.R. Khot, S. Sankaran, and P. Jacoby. 2019. Evaluation of mobile 3D light detection and ranging based canopy mapping system for tree fruit crops. <em>Computers and Electronics in Agriculture,</em>158: 284-293 (doi: 10.1016/j.compag.2019.02.012).</p><br /> <p>Chakraborty, M., L.R. Khot, and R.T. Peters. 2019. Assessing suitability of modified center pivot irrigation systems in corn production using low altitude aerial imaging techniques. <em>Information Processing in Agriculture,</em> In Press (doi: 10.1016/j.inpa.2019.06.001).</p><br /> <p>Chandel, A., L.R. Khot, Y. Osroosh, and R.T. Peters. 2018. Thermal-RGB imager derived in-field apple surface temperature estimates for sunburn management. <em>Agricultural and Forest Meteorology</em>, 253-254: 132-140 (doi: 10.1016/j.agrformet.2018.02.013).</p><br /> <p>He, L., X. Zhang, Y. Ye, M. Karkee, and Q. Zhang. 2019. Effect of shaking location and duration on mechanical harvesting of fresh market apples. <em>Applied Engineering in Agriculture;</em> 35(2): 175-183 (doi: 10.13031/aea.12974).</p><br /> <p>Hohimer, C.J., H. Wang, S. Bhusal, J. Miller, C. Mo, and M. Karkee. 2019. Design and field evaluation of a robot apple harvesting system with 3D printed soft-robotic end-effector. <em>Transactions of the ASABE</em>; 62(2): 405-414 (doi: 10.13031/trans.12986).</p><br /> <p>Khanal, K., S. Bhusal, M. Karkee, P. Scharf, and Q. Zhang. 2019. Design of improved and semi-automated red raspberry cane bundling and tying machine based on the field evaluation results. <em>Transactions of the ASABE. </em>62(3): 821-829 (doi: 10.13031/trans.12973).</p><br /> <p>Osroosh, Y., L.R. Khot, and R.T. Peters. 2019. Detecting fruit surface wetness using a custom-built low-resolution thermal-RGB imager. <em>Computers and Electronics in Agriculture, </em>157: 509–517 (doi: 10.1016/j.compag.2019.01.023).</p><br /> <p>Pena Quinones, A.J., M. Keller, M.R. Salazar-Gutierrez, L.R. Khot, and G. Hoogenboom. 2019. Comparison between grapevine tissue temperature and air temperature. <em>Scientia Horticulturae</em>, 247: 407–420 (doi: 10.1016/j.scienta.2018.12.032). </p><br /> <p>Ranjan, R., A. Chandel, L.R. Khot, H. Bahlol, J. Zhou, R. Boydston, and P. Miklas. 2019. Irrigated pinto bean crop stress and yield assessment using ground based low altitude remote sensing technology. <em>Information Processing in Agriculture</em>, In Press (doi: 10.1016/j.inpa.2019.01.005)<em> </em></p><br /> <p>Ranjan, R., G. Shi, R. Sinha, L. R. Khot, G. Hoheisel, and M. Grieshop. 2019. Automated solid set canopy delivery system for large scale spray applications in perennial tree–fruit crops. <em>Transactions of the ASABE</em>, 62(3): 585-592 (doi: 10.13031/trans.13258). <em> </em></p><br /> <p>Sharda, A., M. Karkee, G. Hoheisel, and Q. Zhang. 2019. Design and evaluation of solid set canopy delivery system for spray application in high-density apple orchards. <em>Applied Engineering in Agriculture</em> 35(5): 751-757 (doi: 10.13031/aea.12512).</p><br /> <p>Sinha, R., L.R. Khot, A. Rathnayake, Z. Gao, and N. Rayapati. 2019. Visible−near infrared spectroradiometry-based detection of grapevine leafroll-associated virus in a red−fruited wine grape cultivar. <em>Computers and Electronics in Agriculture,</em> 162: 165-173 (doi: 10.1016/j.compag.2019.04.008).</p><br /> <p>Sinha, R., L.R. Khot, GA. Hoheisel, M. Grieshop, and H.Y. Bahlol. 2019. Feasibility of a solid set canopy delivery system for efficient agrochemical delivery in vertical shoot positioning trained vineyards. <em>Biosystems Engineering</em>, 179: 59-70 (doi: 10.1016/j.biosystemseng.2018.12.011). </p><br /> <p>Karkee, M., J. Gordón, B. Sallto and M. Whiting, Optimizing fruit production efficiencies via mechanization. 2019. In <em>Achieving sustainable cultivation of temperate zone tree fruits and berries,</em> <em>Volume 1 - Physiology, genetics and cultivation (Editor: Dr Greg Lang)</em>; Burleigh Dodds Science Publishing.</p><br /> <p>Zhang, Q., M. Karkee, and A. Tabb, 2019. The Use of Agricultural Robots in Orchard Management. In <em>Robotics and Automation for a More Sustainable Agriculture (Editor: John Billingsley)</em>; rXiv preprint arXiv:1907.13114.</p><br /> <p>Zhang, Q. 2019. <em>Basics of Hydraulic Systems (2<sup>nd</sup> Edition)</em>. CRC Press, (324 pp).</p><br /> <p>Zhang, X., C. Mo, M.D. Whiting, and Q. Zhang. 2019. Plant-based compositions for the protection of plants from cold damage. US Patent (filed, Docket No. 12770096TA). </p><br /> <h3>West Virginia</h3><br /> <p>L.J. Nixon, A. Tabb, W. M. Morrison, K. Rice, E. G. Brockerhoff, T.C. Leskey, S. Goldson, M. Rostas, Volatile release, mobility, and mortality of diapausing Halyomorpha halys during simulated shipping movements and temperature changes," J Pest Sci (2019). Doi 10.1007/s10340-019-01084-x</p><br /> <ol start="2018"><br /> <li>A. Dias, A. Tabb and H. Medeiros, “Multispecies Fruit Flower Detection Using a Refined Semantic Segmentation Network," in IEEE Robotics and Automation Letters, vol. 3, no. 4, pp. 3003-3010, Oct. 2018. Doi 10.1109/LRA.2018.2849498</li><br /> <li>Stumph, M. Hernandez Virto, H. Medeiros, A. Tabb, S. Wolford, K. Rice, T. Leskey, “Quantifcation of Dispersal Patterns of Invasive Insects with Unmanned Aerial Vehicles," in 2019 IEEE International Conference on Robotics and Automation (ICRA), 2019. doi: 10.1109/ICRA.2019.8794116 and arXiv:1903.00815 [cs.RO].</li><br /> </ol><br /> <p>P.A. Dias, Z. Shen, A. Tabb and H. Medeiros, “FreeLabel: a publicly available annotation tool based on freehand traces," in 2019 IEEE Winter Conference on Applications of Computer Vision (WACV). doi: 10.1109/WACV.2019.00010</p><br /> <h3>Datasets and code releases</h3><br /> <ol start="2019"><br /> <li>Tabb, K. E. Duncan, C. N. Topp, “Code and Data from: Segmenting Root Systems in X-Ray Computed Tomography Images Using Level Sets," [Data set]. Zenodo. 2019. 10.5281/zenodo.3333709, 10.5281/zenodo.3344906</li><br /> <li>Tabb, “Code from: Using cameras for precise measurement of two-dimensional plant features," Ag Data Commons, 2019. https://data.nal.usda.gov/dataset/code-using-cameras-precise-measurement-two-dimensional-plant-features</li><br /> <li>A. Dias, A. Tabb, H. Medeiros, “Multi-species fruit flower detection using a refined semantic segmentation network," Ag Data Commons, 2018. 10.15482/USDA.ADC/1423466</li><br /> </ol>Impact Statements
- West Virginia: Provided resources (data and code) to plant biology community on shape estimation and camera calibration, which is needed for many aspects of plant phenotyping. These resources are publicly posted at Github. Released data and code for root segmentation in XRay CT (for phenotyping of roots), which since July 2019 has had 117 downloads.
Date of Annual Report: 11/05/2020
Report Information
Period the Report Covers: 10/01/2019 - 09/30/2020
Participants
Irwin Gonzalez, University of California, DavisDaeun Choi, Pennsylvania State University
Long He, Pennsylvania State University
Qin Zhang, Washington State University
Brief Summary of Minutes
The 2020 annual meeting was originally planned to be held at Washington State University in September 2020. Due to the Covid-19 pandemic, the meeting was cancelled. The officer team had a zoom meeting to discuss the final report, new officer nomination, and the location for the next annual meeting. The team nominated Yu Jiang (Cornell University) for the new secretary, Daeun Choi (Penn State) and Long He (Penn State) promoted to the vice chair and chair respectively, and Irwin Donis-Gonzalez (UC Davis) became the past chair. The team also agreed to have the 2021 annual meeting at Washington State University.
Accomplishments
<p>Arizona<br />• A high speed and centimeter scale resolution sprayer that can spot apply herbicides to weeds while traveling at speeds that are viable for commercial farming operations was developed and tested. During this review period, an extensive outreach effort was made to educate stakeholders and garner commercial interest in the technology. Over 500 individuals were reached via presentations at field days (1), extension/ag industry meetings (4) and by organizing workshops/tours/field days (2) and hosting student groups (2). Many more were reached through publishing ag industry articles (3) and on-line postings of presentations (3) and device in action videos (2). This activity has led to in-person commercialization conversations with seven start-up companies that are developing automated in-row weeding robots.<br />• Additionally, the technology was further developed so that it is capable of sub-centimeter scale resolution spot spraying at commercially viable speeds. Performance testing and research efforts towards developing a computer imaging system that provides a real-time weed targeting map is planned.</p><br /> <p><br />California<br />• Low-cost wireless mesh network of soil moisture sensors for precision irrigation<br />We have designed a low cost solution of wireless moisture sensors suitable for precision agriculture and urban landscape using LoRa. LoRa communication has been adopted as it enables the mesh network to relay over distances longer than the traditional Wifi or Bluetooth, and with power consumption lower than Zigbee. LoRa module was enabled to work as a provisioned mesh node in which nodes are inter-connected dynamically and non-hierarchically to route to the base node. This way the entire system is decentralized and easy to self-heal in case of node failure. Also the lack of dependency on one node allows for every other node to participate in the relay of information. Low cost capacitance sensors were calibrated and programmed to collect moisture measurement at a frequency of 1 hour. Cloud-based server has been used for off-site data storage and visualization. <br />• Data driven model to estimate actual evapotranspiration from simple on-site weather stations<br />Two commercial all-in-one weather stations were installed next to an EC station over an alfalfa-field to evaluate the possibility of using simple and commercial all-in-one meteorological stations on-site as an alternative to EC measurements to estimate hourly Eta. Different sets of input data (weather parameters) and different machine learning models (Linear regression, Gaussian process regression, support vector machine, and artificial neural networks) will be employed to analyze the ability of data-driven modeling to estimate actual evapotranspiration. The ability of optimization-based machine learning methods like genetic programming and group method of data handling can be analyzed as well. To identify the most relevant set of inputs in ETa modeling, Average Mutual Information (AMI), and Minimum Redundancy Maximum Relevance (MRMR) algorithms will also be used. <br />• Operational and safety evaluation criteria for All-Terrain Vehicles<br /> Several operational and safety evaluation criteria for All-Terrain Vehicle (ATV) equipped with Crush Protection Devices were developed in a study. Enhancing criteria in previous studies. Also, previous studies regarding the CPD performance in ATV rollover incidents were reviewed. <br />Offered a virtual extension course through the Postharvest technology center short course series in the field of agricultural safety and health. <br />Several ATV safety booths were held in different events such as the World Ag Expo and UC Davis field day for FFA and 4H students.<br />• Lift-assist mechanism to adjust the foldable rollover protective structures (FROPS)<br />A lift-assist mechanism to raise and lower the foldable rollover protective structures (FROPS) from the operator's seat was designed, manufactured, and tested. The design considered can be retrofitted and will not modify or compromise the FROPS structure. A universal lift-assist lever design has been constructed and successfully tested for three FROPS of different sizes meeting appropriate ergonomics engineering standards. <br />• Review of injury burden of ATV use in agriculture<br />A comprehensive review was conducted to evaluate the current injury burden of ATV use in agriculture, the need for future research, and possible solutions related to agricultural ATV safety. Potential injury prevention approaches are evaluated based on the hierarchy of control, including elimination or substitution, engineering control, administrative authority, training, and use of personal protective equipment. <br />• Integrated color computer vision system for fresh walnut kernels<br />Designed, evaluated and published findings for a fully integrated color computer vision system to determine external properties such as surface color and textural aspects of peeled fresh walnut kernels. <br />• Portable spectrometers to non-invasively predict table grape quality<br />Published findings related to the study were two commercially available portable spectrometers (F-750: Felix Instruments, WA, USA; and SCiO: Consumer Physics, Tel Aviv, Israel) were evaluated to non-invasively predict quality attributes, including the dry matter (DM), and total soluble solids (TSS) content of three fresh table grape cultivars (‘Autumn Royal’, ‘Timpson’, and ‘Sweet Scarlet’) and one peach cultivar (‘Cassie’). <br />• Internal microstructure of apples using micro computed tomography<br />Developed and published a technique to evaluate internal microstructure of apples using micro computed tomography (-CT) during digestion trials. -CT image slices showed differences in cell membrane structure after digestion. Digested samples contained larger cells, suggesting cellular breakdown induced by the presence of gastric fluid. This observation is not the result of water absorption; as larger cellular structures were not present in apples soaked in water. <br />Offered virtual extension courses through the Postharvest technology center short course series, in the field of none-invasive assessment of quality. <br />Built an online sensing system to predict water activity and moisture content of dried produce using a commercial Time-Domain reflectometry device. Evaluation and validation is ongoing. <br />• Efficient use of water resources in orchard crop production<br />During the 2020 growing season, a leaf monitor system was used in a walnut orchard in Winters, CA to monitor plant water status. The continuous data obtained from leaf monitors were analyzed on a weekly basis and 15-day changes in plant water status were estimated to determine the incidence of stress, if any. The system appeared to work well in keeping the walnut trees stress free throughout the whole season (- only incidence of stress detected was due to pump failure towards the end of the season). A dynamic heat and mass transfer model of the leaf monitoring system is being developed to address the acclimatization issues which appear to effect plant response as season progresses. <br />• Computer-controlled orchard harvest platform<br />A commercial harvest-aid orchard platform was modified so that pickers on the right side of the platform can work at variable heights from the ground. Actuation was performed via hydraulic cylinders under computer control. The platform’s speed was also computer controlled. A stereo camera system in the front of the platform detected apples, and two instrumented picking bags measured each picker’s harvesting rate. An online optimizing controller was developed, tested and tuned to adjust picker working height and platform speed, based on the sensed fruit load and workers’ picking speeds. The objective was to maximize the machine’s harvesting throughput. Experiments were performed in September 2020, in a Fuji apple orchard, in Lodi CA, and 3.3 tn of apples were harvested, in total. Results showed that the machine’s harvesting speed increased by 11.3% to 26% compared to the speed of the conventional, commercial platform.<br />A simulator was further developed and calibrated to model crew harvest activities and robot fruit-transporting activities during strawberry harvesting. Robot scheduling algorithms were tested using the simulator, and labor efficiency increases ranged between 15% and 24%.<br />• Novel crop recognition technique for robotic weed control<br />A novel technique using crop signaling to detect and classify weeds vs. crop plants for robotic weed control in leafy vegetables was successfully developed. Three different crop signaling-based machine vision systems were developed for plant labels, topical markers, and systemic markers. Several trials have been conducted for weed control in both tomato and lettuce fields. The experimental result shows that the system can detect and distinguish crop plants from weeds with 100% accuracy in a field having densely populated weed with no false-positive error.<br />• AI technologies for agricultural production<br />Developed a deep learning imaging and analysis system for direct biomass prediction of leafy greens grown in a controlled indoor environment. The system enables non-destructive monitoring of growth rates for individual plants and is capable of detecting stress induction with 1-2 days. We are in the process of preparing a manuscript for submission. <br />Developed and tested an AI-enabled a prototype sensing kit that mounts on existing farm vehicles and uses computer vision to estimate yield at about 2 – 3 m resolution within vineyards. The kit includes custom lighting, GPU processing, GPS, power distribution, and accompanying enclosures. We have recorded data for about 50k grapevine plants with corresponding harvester-based yield monitor data. This data will be used to train a deep learning model for predicting, and eventually forecasting yield.</p><br /> <p><br />Connecticut<br />A multi-disciplinary team at the University of Connecticut began work to develop a monitoring system for insect pest damage using small unmanned aerial systems (UAS) outfitted with spectral sensors. The project objective is to detect the presence and early plant damage caused by the potato leafhopper Empoasca fabae on beans. Experiments are underway to test the impact of the potato leafhopper feeding on 3 different bean cultivars and associated leaf spectra. Unfortunately, due to the COVID-19 pandemic, field tests were not possible as originally planned.</p><br /> <p><br />Georgia<br />• Fruit traits such as cluster compactness, fruit maturity, and berry number per clusters are important to blueberry breeders and producers for making informed decisions about genotype selection related to yield traits and harvestability as well as for plant management. We developed a data processing pipeline to count berries, to measure maturity, and to evaluate compactness (cluster tightness) automatically using a deep learning image segmentation method for four southern highbush blueberry cultivars (‘Emerald’, ‘Farthing’, ‘Meadowlark’, and ‘Star’). <br />• Early detection of internal bruises in blueberries is a significant challenge for the blueberry industry. We developed a method to detect blueberries’ internal bruising accurately, after mechanical damage from hyperspectral transmittance images (HSTIs), using the deep learning-based method of fully convolutional networks (FCNs) for segmentation tasks. To improve detection accuracy, a total of three classes (bruised tissue, unbruised tissue, and calyx end of blueberries), were treated as segmentation targets. A near-infrared hyperspectral imaging system was used to acquire transmittance images of 1200 blueberries, and the images were divided randomly to form training, validation, and testing sets. Three categories of input HSTIs were used to evaluate the FCN models using pre-trained weights (transfer learning) and random initialization. Random forests and linear discriminant analysis were applied to generate 9-channel and 3-channel input images along with full-wavelength multi-channel images. The results indicate that when using the deep learning approach, blueberry bruises and calyx ends can be segmented from the blueberry fruit as early as 30 min after mechanical damage has been inflicted on the blueberries. The new full-wavelength model with random initialization had the highest accuracy (81.2% over the entire test set), and can be used to research the resistance of blueberry fruit to mechanical damage. The new 3-channel and 9-channel models show potential for application to packing-line detection and online inspection.<br />• We also developed robotic platforms to be used for in-field plant high throughput phenotyping.</p><br /> <p><br />Iowa<br />• Modelled and measured the soil reaction forces on a rotating time mechanism to be use for robotic mechanical weeding.<br />• Launched the ISU Soil-Machine Dynamics Laboratory for investigating ground-engaging tool performance and wear and traction system mobility.<br />• Developed navigation controller for the field-based plant phenotyping platform – PhenoBot 3.0. Both under- and above-canopy vision based row detection algorithms and navigation control algorithms based on Pure Pursuit and Linear Quadratic Gaussian were developed for PhenoBot 3.0 and field tested this summer. Machine learning algorithms for stalk (StalkNet) and leaf angle (AngleNet) detection for maize and sorghum plants were developed; and in conjunction with our customized PhenoStereo 3D sensor, highly accurate stalk size and leaf angle measurements were obtained.</p><br /> <p><br />Michigan<br />• The potential for Computed Tomography (CT) was demonstrated in some cases coupled with parallel studies of hyperspectral imaging and spectroscopy, to have good potential for non-destructive sensing of internal defects and attributes in various specialty crops which are important to consumers and not measurable manually or with presently available sorting technology. While no direct studies or research was conducted related to this programming during this reporting period, opportunity for moving the concept of CT technology for sorting specialty crops to commercial application was continued through collaboration with a specialty crop importer/exporter having special interest in chestnut. Through this entity, a company with electronic sorting and measurement experience including the development of new high-speed CT imaging has been brought to the table and is participating in testing and data analysis algorithm development focused on chestnut defect/quality assessment. Results are promising to date with further testing planned this season. <br />• Potential chestnut harvest solutions ranging from manual harvest assist tooling (“basic automation”), low-power mechanical systems, to possible opportunities for feasible small but multipliable robotic or co-robotic technology has been worked on or investigated with a faculty-student extracurricular engineering group and Mechanical Engineering colleagues interested in sustainable agriculture solutions. Possible external proposal opportunities have also been identified and will likely be pursued. Harvest assist prototype systems were built and evaluated, however the programming was put on hold with the COVID-19 closure of the university. Many chestnut producers are ‘caught’ between being of size large enough to afford European mechanical harvesters but yet are too large to conduct harvest manually as their orchards come into full production, and thus a void exists in feasible harvest solutions.<br />• Pawpaws are limited in the opportunity to advance into a more substantial or significant commodity due to their need to nearly fully tree ripen and because they are relatively easy to damage during handling once ripe. Thus, opportunity for incorporation into the fresh market is very limited and the skin/peel must be removed prior to any attempt to process, such as into a puree. A student-faculty-stakeholder team investigated concepts to improve effectiveness and efficiency of peel removal and potentially, either partially or fully, automate the process. Success was obtained in both efficiency and effectiveness in getting a pawpaw to a “state” such that the peel could easily be removed with very minimal loss of desirable flesh. Concepts for automating the process were proposed should the level of pawpaw production warrant such.<br />• Development of a text on Tree Fruit Automation was led by two colleagues of this multi-state project and involved multiple W3009 members as well as other national and international colleagues. The contribution from this specific project has been a chapter on Tree Fruit Harvest Technology.</p><br /> <p><br />Oklahoma<br />• We focused on three tasks: 1) Re-design and re-configure a drone platform; 2) Re-design the ground-based, remote-controlled peanut phenotyping platforms; and 3) Develop image processing and analysis algorithms for counting peanut flowers within canopy and identify diseased plants.<br />• The drone used for experiment in previous years carried only an RGB camera, which limited its applications. We designed a new drone system which could carry multiple cameras/sensors capture images simultaneously. In the summer 2020, the drone was used to collect RGB, thermal, and NDVI images for peanut field. All the data collected were time and location stamped. <br />• The previously designed ground-based peanut phenotyping system was very bulky and the mechanical driving modules were not running smoothly in the field. For the image acquisition, the shadows from the platform affected the quality of the collected images. Another problem was that the platform was driven by two deep-cycle batteries, which could only last for 1.5 hours. We upgraded the old platforms with the new design of the mechanical driving modules to optimize the maneuverability of the platforms, adding new shading materials to avoid the shadow, and using solar panels with the batteries to extend the running time of the platform. <br />• Due to the Covid-19 pandemic and unstable weather conditions, we were not able to complete the all planned field experiment. Hence, the data for validation process on the system design and algorithm development was not sufficient.</p><br /> <p><br />Pennsylvania<br />• Orchard temperature profiles were measured utilizing a propane heater in April 2020. This effort was part of the project on automated frost protection. Air temperatures were measured in horizontal grid and vertical grid patterns to determine the distribution of heat. This will help determine the movement patterns of autonomous ground vehicles carrying the heaters.<br />• The RootRobot unit, part of the DOE ARPA-E DEEPER project, was further tested in the laboratory, then brought to the field in September 2020 to begin tests on actual corn plantings. The RootRobot excavates and prepares corn stalks for phenotyping through imaging<br />• A three-rotational (3R) degrees of freedom (DoF) end-effector was designed and integrated with a cartesian manipulator by considering maneuvering, spatial, mechanical, and horticultural requirements. Simulations and a series of field work were conducted to test the performance of the system. The field tests validated the simulation results, and the end-effector successfully cut branches up to ~25 mm diameter at wide range of orientations. Meanwhile, a simulation study focused on investigating the branch accessibility of a six-rotational (6R) degrees of freedom (DoF) robotic manipulator with a shear blade type end-effector. With the developed algorithms, a collision-free path was created to the targeted branch in the virtual environment. <br />• An unmanned ground-based canopy density measurement system was developed to support precision spraying in apple orchards. A data processing and analysis algorithm was also developed to measure point cloud indices from 3D LiDAR sensor to describe the distribution of tree canopy density. Finally, a canopy density map was generated to provide a graphical view of the tree canopy density in different sections.<br />• An IoT-based precision irrigation system with LoRaWAN technology was developed and evaluated in both vegetable field and tree fruit orchards. Soil moisture sensors were installed in the field, and the data was sent to a cloud-based platform in real-time (10 minutes interval). The irrigation system was automatically operated with controlling the solenoid valves to apply water to the field based on the soil moisture thresholds. <br />• Heating requirement maps were created utilizing UAV-based thermal and RGB cameras, computer vision techniques, and artificial flower bud critical temperatures to simulate orchard heating demands during frost events. The results demonstrated the feasibility of the proposed orchard heating requirement determination methodology, which has the potential to be a critical component of an autonomous, precise frost management system. <br />• A 3D machine vision system was developed to detect individual mushrooms among highly clustered crops. A practical 3D mobile imaging platform for mushroom production beds acquired RGB-D images from various angles, created high-resolution composite point cloud images using an iterative closest algorithm. Mushroom detection accuracies using various point cloud image resolutions were compared to investigate an optimal level of image details for both processing time and accuracy of the system. <br />• An external lighting system using LEDs by over-current was developed to produce a powerful flash was developed as a viable active lighting source for daytime imaging. The system was deployed to an apple orchard to take images in both sunny and cloudy days on different canopy structures. The results indicate a substantial improvement over using a camera’s auto-exposure setting for outdoor imaging regarding both color consistency and motion blur effects on images.</p><br /> <p><br />Washington<br />• Optimized a vision-based fruit orientation estimation and obstacle avoidance system for a 12-armed robotic apple harvester.<br />• Developed a machine-vision system for detecting flower clusters (with 86% accuracy) and estimating flower density (with 84% accuracy) in apple and cherry orchards, which will be used in developing an automated thinning and pollination systems.<br />• Developed a machine-vision and robotic system for pruning apple trees, which was tested in the laboratory environment with more than 90% accuracy in reaching and cutting target branches.<br />• Developed and tested an automated green shoot thinning mechanism for grapevines (with 1.4 cm cordon following accuracy) that used a deep-learning-based machine vision system for detecting vine cordon and shoots and for estimating trajectory fitting model which reached a 80% cordon trajectory estimation accuracy.<br />• Further optimized a fixed spray system (i.e. solid set chemical delivery system configurations and pertinent field validation for tree fruit orchards.<br />• Improved and conducted field testing of an Internet-of-Things (IoT) enabled Crop Physiology Sensing System (CPSS) for apple fruit surface temperature monitoring and real-time actuation of modified evaporative cooling system for heat stress management in fresh market apple cultivars.<br />• Developed hyperspectral imaging based spectral sensing for grapevine leafroll disease detection at asymptomatic stages and pertinent data mining for identification of salient spectral bands.</p><br /> <p><br />West Virginia<br />The USDA-ARS-AFRS location in Kearneysville, WV concentrated on developing tools for plant phenotyping using computer vision, specifically for shape. Publications included the extension of one such tool for trees, applied to medical domain (Zhu et al. 2020), a collaboration in strawberry (Feldmann et al. 2020), and studies for automated mark-and-release for invasive insects (Kirkpatrick et al. 2020). Code and data was released for two tools as well.</p>Publications
<p>Arizona<br />Raja, R., Slaughter, D.C., Fennimore, S.A., Nguyen, T.T., Vuong, V.L., Sinha, N., Tourte, L., Smith, R.F. & Siemens, M.C. 2019. Crop signaling: A novel crop recognition technique for robotic weed control. Biosystems Eng. 187: 278-291.</p><br /> <p><br />California<br />Rojo, Francisco, Rajveer Dhillon, Shrinivasa Upadhyaya, and Bryan Jenkins. 2020. Development of a dynamic model to estimate canopy par interception. Biosystems Eng. 198:120-136<br />Rojo, Francisco, Rajveer Dhillon, and Shrinivasa Upadhyaya. 2020. Comparing ground-based par interception data with UAV images and sun position. Submitted for publication is Applied Engineering in Agriculture.<br />Khorsandi, F., P.D. Ayers, E.J. Fong. 2019. Evaluation of Crush Protection Devices for agricultural All-Terrain Vehicles. Biosystems Engineering. Volume 185, September 2019, Pages 161-173<br />Peng, C., Vougioukas, S.G. (2020). Deterministic predictive dynamic scheduling for crop-transport co-robots acting as harvesting aids. Computers and Electronics in Agriculture, 178, 105702. https://doi.org/10.1016/j.compag.2020.105702 <br />Fei, Z., Shepard, J., Vougioukas, S.G. (2020). Instrumented Picking Bag for Measuring Fruit Weight During Manual Harvesting. Transactions of the American Society of Agricultural and Biological Engineering. IN PRESS.<br />Thayer, T., Vougioukas, S.G., Goldberg, K., Carpin, S. (2020). Multi-Robot Routing Algorithms for Robots Operating in Vineyards. IEEE Transactions on Automation Science and Engineering, 17(3): 1184-1194. https://doi.org/10.1109/TASE.2020.2980854 <br />Seyyedhasani, H., Peng, C., Jang, W., Vougioukas, S.G. (2020). Collaboration of Human Pickers and Crop-transporting Robots during Harvesting - Part I: Model and Simulator Development. Computers and Electronics in Agriculture. (172): p.105324. https://doi.org/10.1016/j.compag.2020.105324 <br />Seyyedhasani, H., Peng, C., Jang, W., Vougioukas, S.G. (2020). Collaboration of Human Pickers and Crop-transporting Robots during Harvesting - Part II: Simulator Evaluation and Robot-Scheduling Case-study. Computers and Electronics in Agriculture. (172): p.105323. https://doi.org/10.1016/j.compag.2020.105323<br />Agricultural All-Terrain Vehicle Safety. Committee on Agricultural Safety and Health Research and Extension. 2020. Agricultural All-Terrain Vehicle Safety. USDA-NIFA. Washington, DC.<br />Ayers, P.D., F.K. Khorsandi, M.J. Poland, C.T. Hilliard. 2019. Foldable rollover protective structures: Universal lift-assist design. Biosystems Engineering. Volume 185, September 2019, Pages 116-125<br />Khorsandi, et al. In Press. A manuscript titled “Agricultural All-Terrain Vehicle Safety: Hazard Control Methods Using the Haddon Matrix” will be published in Journal of Aeromedicine.<br />Donis-Gonzalez, I.R., Valero, C., Momin, M.A., Kaur, A., Slaughter, D.C., 2020. Performance Evaluation of Two Commercially Available Portable Spectrometers to Non-Invasively Determine Table Grape and Peach Quality Attributes. AGRONOMY-BASEL Vol. 10: DOI: 10.3390/agronomy10010148.<br />Donis-Gonzalez, I.R., Sidelli, G., Bergman, S.M., Slaughter, D.C., Crisosto, C.H. 2020. Color Vision System to Assess English Walnut (Juglans Regia) Kernel Pellicle Color. Postharvest Biology and Technology. Volume 167, September 2020, 111199.doi.org/10.1016/j.postharvbio.2020.111199<br />Olenskyj, A.G., I.R. Donis-González, G.M. Bornhorst. 2020. Nondestructive characterization of structural changes during in vitro gastric digestion of apples using 3D time-series micro-computed tomography. Journal of Food Engineering. Volume 267, February 2020, 109692<br />Raja, R., Slaughter, D.C., Fennimore, S.A., Nguyen, T.T., Vuong, V.L., Sinha, N., Tourte, L., Smith, R.F., Siemens, M.C., 2019. Crop signalling: A novel crop recognition technique for robotic weed control. Biosystems Engineering. Vol 187: 278-291. DOI: 10.1016/j.biosystemseng.2019.09.011.<br />Raja, R., Nguyen, T.T., Slaughter, D.C., Fennimore, S.A., 2020. Real-time robotic weed knife control system for tomato and lettuce based on geometric appearance of plant labels. Biosystems Engineering. Vol. 194: 152-164.<br />Kennedy, H. Fennimore, S.A., Slaughter, D.C., Nguyen, T.T., Vuong, V.L., Raja, R., Smith R.F., Crop Signal Markers Facilitate Crop Detection and Weed Removal from Lettuce and Tomato by an Intelligent Cultivator. Weed Technology. DOI: <a href="https://doi.org/10.1017/wet.2019.120">https://doi.org/10.1017/wet.2019.120</a></p><br /> <p><br />Georgia<br />Iqbal, J., Xu, R., Halloran, H., and Li, C. 2020. Development of a Multi-Purpose Autonomous Differential Drive Mobile Robot for Plant Phenotyping and Soil Sensing. Electronics, 9 (9), 1550.<br />Ni, X, Li, C, Jiang, H, and Takeda, F. 2020. Deep learning image segmentation and extraction of blueberry fruit traits associated with harvestability and yield. Horticulture Research, 7 (1), 1-14.<br />Iqbal J., Xu, R., Sun, S., and Li, C. 2020. Simulation of an autonomous mobile robot for LiDAR-based in-field phenotyping and navigation. Robotics, 9 (2), 46.<br />Jiang, Y. and Li, C. 2020. Convolutional neural networks for image-based high throughput plant phenotyping: A review. Plant Phenomics, 2020 (4152816).<br />Jiang, Y., Snider, J. L., Li, C., Rains, G. C., and Paterson, A. H. 2020. Ground based hyperspectral imaging to characterize canopy-level photosynthetic activities. Remote Sensing, 12 (2), 315.<br />Zhang, M., Jiang, Y., Li, C., and Yang, F. 2020. Fully convolutional networks for blueberry bruising and calyx segmentation using hyperspectral transmittance imaging. Biosystems Engineering, 192, 159-175.</p><br /> <p><br />IOWA<br />Kshetri, S., B. L. Steward, J.J. Jiken, L. Tang, and M. Tekeste. 2019. Investigating effects of interaction of single tine and rotating tine mechanism with soil on weeding performance using simulated weeds. Transactions of the ASABE 62(5): 1283-1291. <br />Gai, J., L. Tang, and B.L. Steward. 2020. Automated crop plant detection based on the fusion of color and depth images for robotic weed control. Journal of Field Robotics. 37(1): 35-52. <br />Schramm, M. W., H. M. Hanna, M. J. Darr, and S. J. Hoff, and B. L. Steward. 2019. Sub-second wind velocity changes one meter above the ground. Applied Engineering in Agriculture 35(5): 697-704. <br />Mantilla-Perez, M. B., Y. Bao, L.Tang, P. S. Schnable, M. G. Salas-Fernandez. 2020. Towards "smart canopy" sorghum: discovery of the genetic control of leaf angle across layers. Plant Physiology, DOI: https://doi.org/10.1104/pp.20.00632</p><br /> <p>Steward, B. L., H. M. Hanna, P. M. Dixon, R. K. Mompremier. 2019. Measuring and modeling the movement of spray droplets into off-target areas. ASABE Paper No. 1901496. St. Joseph, Mich.: ASABE. DOI: doi.org/10.13031/aim.201901496 <br />Gai, J., T. Tuel, L. Xiang, L. Tang. 2020. PhenoBot 3.0 - an Autonomous Robot for Field-based Maize/Sorghum Plant Phenotyping, Phenome 2020, Tucson, AZ, February 24-27.<br />Gai, J., T. Tuel, L. Xiang, L. Tang. 2020. Developing the Control System of an Autonomous Robot for Field-based Maize/Sorghum Plant Phenotyping, 2020 ASABE Annual International Meeting, Omaha, Nebraska, July 12–15, 2020.<br />Xiang, L., J. Gai, L. Tang. 2020. Developing a high-throughput stereo vision system for plant phenotyping. 2020 Phenome, Tucson, AZ, Feb. 24-27, 2020. <br />Xiang, L., L. Tang, J. Gai, & L. Wang. 2020. PhenoStereo: a high-throughput stereo vision system for field-based plant phenotyping-with an application in sorghum stem diameter estimation. 2020 ASABE Annual International Virtual Meeting. July 13-15, 2020. Paper No. 2001190</p><br /> <p><br />Michigan<br />Rady, A.M., Guyer, D.E., Donis-Gonzalez, I.R., Kirk, W., Watson, N.J. 2020. A comparison of different optical instruments and machine learning techniques to identify sprouting activity in potatoes during storage. Journal of Food Measurement and Characterization. doi.org/10.1007/s11694-020-00590-2.</p><br /> <p><br />Pennsylvania<br />Refereed Journals<br />Caliskan-Aydogan, O., H. Yi, J.R. Schupp, D. Choi, P. H. Heinemann, V. M. Puri. 2020. Changes in thermal properties of 'Gala' apple during the growing season. Transactions of the ASABE, 63(2), 305-315.<br />Fu, H., Karkee, M., He, L., Duan, J., Li, J., & Zhang, Q. (2020). Bruise Patterns of Fresh Market Apples Caused by Fruit-to-Fruit Impact. Agronomy, 10(1), 13. <br />Kon, T. M., J. R. Schupp, M. A. Schupp, and H.E. Winzeler. 2020. Screening thermal shock as an apple blossom thinning strategy. II. Pollen tube growth and spur leaf injury in response to thermal shock temperature and duration. HortScience, 55(5), 632-636.<br />Kon, T. M., M. A. Schupp, H.E. Winzeler and J. R. Schupp. 2020. Screening thermal shock as an apple blossom thinning strategy. I. Stigmatic receptivity, pollen tube growth, and leaf injury in response to thermal shock temperature and timing. HortScience, 55(5), 625-631.<br />Zahid, A., He, L., Zeng, L., Choi, D., Schupp, J., & Heinemann, P. (2020). Development of a Robotic End-Effector for Apple Tree Pruning. Transactions of the ASABE, 63, 847-856.<br />Zahid, A., Mahmud, M., He, L., Choi, D., Schupp, J., & Heinemann, P. Development of an Integrated 3R End-effector with a Cartesian Manipulator for Pruning Apple Trees. Computers and Electronics in Agriculture, 179.<br />Zeng, L., Feng, J., & He, L. (2020). Semantic segmentation of sparse 3D point cloud based on geometrical features for trellis-structured apple orchard. Biosystems Engineering, 196, 46-55.<br />Zhang, X., He, L., Karkee, M., Whitting, M., & Zhang, Q. (2020). Field Evaluation of Targeted Shake-and-Catch Harvesting Technologies for Fresh Market Apple. Transactions of the ASABE. [In press].<br />Zhang, X., He, L., Zhang, J., Whiting, M. D., Karkee, M., & Zhang, Q. (2020). Determination of key canopy parameters for mass mechanical apple harvesting using supervised machine learning and principal component analysis (PCA). Biosystems Engineering, 193, 247-263.</p><br /> <p><br />Non-refereed publications<br />Choi, D., J. Schupp, T. Baugher, and L. He. Evaluation of effective canopy depths of apple trees for optimal machine sensing performance – Year2/2. PA Fruit News 100(1):39-41.<br />He, L. (2020). Drip Irrigation and Sensor-Based Precision Irrigation. In the Penn State Tree Fruit Production Guide. (2020-2021), (pp. 426-430).<br />He, L., & Weber, D. (2020). Updates on Soil Moisture-Based Irrigation for Orchards. Pennsylvania Fruit News.<br />He, L., D. Choi, J. Schupp and T. Baugher. 2020. A sensor-based irrigation test system for apple orchards (Final report). PA Fruit News 100(1):24-26.<br />He, L., J. Schupp, D. Choi and D. Weber. 2020. Branch and fruit accessibility for mechanical operations with various tree canopies (Year 1 report). PA Fruit News 100(1):22-24.<br />Huang, M., He, L., Jiang, X., Choi, D., & Pecchia, J. (2020). Hand-picking Dynamic Analysis for Robotic Agaricus Mushroom Harvesting. Paper No. 2000415. 2020 ASABE Annual International Meeting.<br />Jarvinen, T., Choi, D., Heinemann, P., & Baugher, T. A. (2019). Tree trunk position estimation for accurate fruit counts in apple yield mapping. 2019 ASABE Annual International Meeting, Paper No. 1900918, July 7 – July 10, 2019. (pp. 1-7).<br />Jiang, X., He, L., & Tong, J. (2020). Investigation of Soil Wetting Pattern in Drip Irrigation using LoraWAN Technology. Paper No. 2000419. 2020 ASABE Annual International Meeting.<br />Lee, C.-H., Choi, D., Pecchia, J., He, L., & Heinemann, P. (2019). Development of A Mushroom Harvesting Assistance System using Computer Vision. 2019 ASABE Annual International Meeting, Paper No. 190050, July 7 – July 10, 2019. (pp. 1-5).<br />Mahmud, M. S., & He, L. (2020). Measuring Tree Canopy Density Using A Lidar-Guided System for Precision Spraying. Paper No. 2000554. 2020 ASABE Annual International Meeting.<br />Mirbod, O., Choi, D., Heinemann, P., & Marini, R. (2020). Towards image-based measurement of accurate apple size and yield using stereo vision cameras. 2020 ASABE Annual International Meeting, Paper No. 2001115, July 12- 15, 2020. (pp. 1-6).<br />Schupp, J., H. E. Winzeler and M. Schupp. 2020. Blossom Thinning Pennsylvania Apples Using the Pollen Tube Growth Model. PA Fruit News 100 (1):46-47.<br />Schupp, J., H. E. Winzeler and M. Schupp. 2020. Development of a High Density, Highly Mechanized, Pedestrian Peach System. PA Fruit News 100 (1): 43-44.<br />Schupp, J., L. He, H. E. Winzeler, M. Schupp and M. Clowney. 2020. Improving orchard performance with terrain analysis using drone technology and Geographical Information Systems. PA Fruit News 100 (1):45-46. <br />Shi, X., Choi, D., Heinemann, P., Lynch, J. P., & Hanlon, M. (2019). RootRobot: A Field-based Platform for Maize Root System Architecture Phenotyping. 2019 ASABE Annual International Meeting, Paper No.1900806, July 7 – July 10, 2019. (pp. 1-7).<br />Zahid, A., He, L., Choi, D., Schupp, J., & Heinemann, P. (2020). Collision free Path Planning of a Robotic Manipulator for Pruning Apple Trees. Paper No. 2000439. 2020 ASABE Annual International Meeting.<br />Zhang, H., He, L., Di Gioia, F., Choi, D., & Heinemann, P. (2020). Internet of Things (IoT)-based Precision Irrigation with LoRaWAN Technology Applied to High Tunnel Vegetable Production. Paper No. 2000762. 2020 ASABE Annual International Meeting.</p><br /> <p><br />Patent<br />Lyons, D.J. & Heinemann, P.H. 2019. US patent No. 10,448,578 B2: Selective Automated Blossom Thinning. October 22, 2019.</p><br /> <p><br />Extension Presentation<br />Schupp, J. 2020. Blossom thinning Golden Delicious using lime sulfur and the pollen tube growth model. Mid-Atlantic Fruit and Vegetable Conference, Hershey, PA. January 28, 2020.<br />Schupp, J., 2020. Research on fruit thinning. Ohio Produce Network, Columbus, OH. 23 Jan 2020.<br />Schupp, J., 2020. Research on orchard systems/ pruning. Ohio Produce Network, Columbus, OH. 23 Jan 2020<br />Schupp, J., and D. Weber. 2020. Demonstrations of the REDpulse pneumatic defoliator for increasing red coloration of apples. Biglerville, PA. 19 and 20 August 2020.<br />Schupp, J., He, L., H. E. Winzeler, M. Schupp and M. Clowney. 2020. Improving orchard performance with terrain analysis using drone technology and Geographical Information Systems (GIS). Mid-Atlantic Fruit and Vegetable Conference, Hershey, PA. January 28-30, 2020 (poster).<br />Schupp, J., M. Schupp and H. E. Winzeler. 2020. High density mechanized pedestrian peach system. Mid-Atlantic Fruit and Vegetable Conference, Hershey, PA. January 28-30, 2020 (poster).</p><br /> <p><br />Award<br />Crassweller, R., K. Peter, G. Krawczyk, J. Schupp, T. Ford, M. Brittingham, J. Johnson, L. LaBorde, J. Harper, K., Kephart, R. Pifer, K. Kelley, L. He, P. Heinemann, D. Biddinger, M. Lopez-Uribe, R. Marini, T. Baugher, D. Weber, L. Kime, E. Crow, E. Weaver, B. Lehman. 2020. 2020-21 Penn State Tree Fruit Production Guide. The Outstanding Book Publication from the American Society for Horticultural Science.</p><br /> <p><br />Washington<br />Journal Articles<br />Bahlol, H., A. Chandel, G.-A. Hoheisel and L. R. Khot. 2020. Developing understanding on orchard sprayer air-assists and volume output patterns using smart spray analytical system. Crop Protection, 127: 104977. https://doi.org/10.1016/j.cropro.2019.104977 <br />Bahlol, H. Y., A. Chandel, G.-A. Hoheisel, and L. R. Khot. 2020. Smart spray analytical system for orchard sprayer calibration: a-proof-of-concept and preliminary results. Transactions of the ASABE, 62(6): 29-35. https://doi.org/10.13031/trans.13196 .<br />Davidson, J., S. Bhusal, C. Mo, M. Karkee, and Q. Zhang. 2020. Robotic Manipulation for Specialty Crop Harvesting: A Review of Manipulator and End-Effector Technologies. Global Journal of Agriculture and Allied Sciences, 2(1), 25-41. https://doi.org/10.35251/gjaas.2020.004. <br />Fu, H., M. Karkee, L. He, J. Duan, J. Li, and Q. Zhang, 2020. Bruise patterns of fresh market apples caused by fruit-to-fruit impact. Agronomy, 10(1), Article 59. (http://doi.org/10.3390/agronomy 10010059).<br />Fu, L., Y. Majeed, X. Zhang, M. Karkee and Q. Zhang, 2020. Faster R-CNN-based apple detection in dense-foliage fruiting-wall trees using RGB and depth features for robotic harvesting. Biosystems Engineering, 197: 245-256. http://doi.org/10.1016/j.biosystemseng.2020.07.007.<br />Gao, F., L. Fu, X. Zhang, Y. Majeed, R. Li, M. Karkee and Q. Zhang. 2020. Multi-class fruit-on-plant detection for apple in SNAP system using Faster R-CNN. Computers and Electronics in Agriculture, 176, 105634. http://doi.org/10.1016/j.compag.2020.105634. <br />Gao, Z., R. A. Naidu, Q. Zhang, and L. R. Khot. 2020. Early detection of grapevine leafroll disease in a red-berried wine grape cultivar using hyperspectral imaging. Computers and Electronics in Agriculture, 179, 105807 https://doi.org/10.1016/j.compag.2020.105807<br />Khot, L. R.*. 2020. Transitioning from precision to decision horticulture: technology landscape. ISHS Acta Horticulturae 1279, XXX International Horticultural Congress IHC2018: VII Conference on Landscape and Urban Horticulture, IV Conference on Turfgrass Management and Science for Sports Fields and II Symposium on Mechanization, Precision Horticulture, and Robotics, 43:1, https://doi.org/10.17660/ActaHortic.2020.1279.29 <br />Long, Y., M. Li, D. Gao, Z. Zhang, H. Sun, and Q. Zhang, 2020. Chlorophyll content detection based on image segmentation by plant spectroscopy. Spectroscopy and Spectral Analysis, 40(7): 2253-2258. https://doi.org/10.3964/j.issn. 1000-0593(2020)07-2253-06.<br />Majeed, Y., J. Zhang, X. Zhang, L. Fu, M. Karkee, Q. Zhang, and M.D. Whiting, 2020. Deep learning based segmentation for automated training of apple trees on trellis wires. Computers and Electronics in Agriculture, 170. Article 105277. (https://doi.org/10.1016/J.COMPAG.2020.105277).<br />Majeed, Y., M. Karkee, Q. Zhang, L. Fu, and M.D. Whiting, 2020. Determining grapevine cordon shape for automated green shoot thinning using semantic segmentation-based deep learning networks. Computers and Electronics in Agriculture, 171. Article 105308. (https://doi.org/10.1016/J.COMPAG.2020.105308).<br />Majeed, Y., M. Karkee, and Q. Zhang, 2020. Estimating the trajectories of vine cordons in full foliage canopies for automated green shoot thinning in vineyards. Computers and Electronics in Agriculture, 176. Article 105671. (https://doi.org/10.1016/j.compag.2020.105671).<br />Zhang, J., M. Karkee, Q. Zhang, X. Zhang, Y. Majeed, L. FU, and S. Wang, 2020. Multi-class object detection using faster R-CNN and estimation of shaking locations for automated shake-and-catch apple harvesting. Computers and Electronics in Agriculture, 173. Article 105384. (doi: 10.1016/J.COMPAG.2020.105384).<br />Zhang, X., L. He, J. Zhang, M.D. Whiting, M. Karkee and Q. Zhang, 2020. Determination of key canopy parameters for mass mechanical apple harvesting using supervised machine learning and principal component analysis (PCA). Biosystems Engineering, 193: 247-263. (doi: 10.1016/j.biosystemseng.2020.03.006).<br />Zhang, X., He, L., Karkee, M., Whiting, M. D., and Zhang, Q. 2020. Field Evaluation of Targeted Shake-and-Catch Harvesting Technologies for Fresh Market Apple. Transactions of the ASABE, 2020. doi: 10.13031/trans.13779.<br />Ranjan, R., L. R. Khot, R. Troy Peters, M. R. Salazar-Gutierrez and G. Shi. 2020. In-field crop physiology sensing aided real-time apple fruit surface temperature monitoring for sunburn prediction. Computers and Electronics in Agriculture, 157: 105558. https://doi.org/10.1016/j.compag.2020.105558.<br />Santiago, W. E., N. J. Leite, B. J. Teruel, M. Karkee, and C. A.M. Azania. 2019. Evaluation of bag-of-features (BoF) technique for weed management in sugarcane production. Australian Journal Crop of Science, 13(11):1819-1825. <br />Sinha, R., R. Ranjan, H. Y. Bahlol, L. R. Khot, G.–A. Hoheisel and M. Grieshop. 2020. Development and performance evaluation of a pneumatic spray delivery based solid set canopy delivery system for high-density apple orchard. Transactions of the ASABE, 62(6): 37-48. https://doi.org/10.13031/trans.13411 <br />Sinha, R., R. Ranjan, G. Shi, G.-A. Hoheisel, M. Grieshop and L. R. Khot. 2020. Solid set canopy delivery system for efficient agrochemical delivery in modern architecture apple and grapevine canopies. Acta Horticulturae 1269: II International Symposium on Innovative Plant Protection in Horticulture, 277-286. https://doi.org/10.17660/ActaHortic.2020.1269.38 <br />Wang, B., R. Ranjan, L. R. Khot and R. Troy Peters. 2020. Smartphone application‐enabled apple fruit surface temperature monitoring tool for in‐field and real‐time sunburn susceptibility prediction. Sensors, 20, 608. <a href="https://doi:10.3390">https://doi:10.3390/s20030608</a></p><br /> <p><br />Thesis/Dissertations<br />Gao, Zongmei (2020). Spectral imaging based non-contact detection of biotic and abiotic stress in berry crops. Ph.D. Dissertation. April 2020, Washington State University.<br />Majeed, Yaqoob (2020). Machine Vision System for the Automated Green Shoot Thinning in Vineyards. Ph.D. Dissertation. April 2020, Washington State University.<br />Zhang, Xin (2020). Study of Canopy-Machine Interaction in Mass Mechanical Harvest of Fresh Market Apples. Ph.D. Dissertation. March 2020, Washington State University.</p><br /> <p><br />Conference Paper and Presentations<br />Anura P. Rathnayake, G. A. Hoheisel and L. R. Khot. 2020. A PWM based retrofit controller for optimized spray applications in perennial specialty crops. Paper No. 2001023, ASABE 2020 Virtual Annual International Meeting, July 12-15, 2020 (Oral Presentation).<br />Bhattarai, U. Automated Blossom Detection in Apple Trees using Deep Learning. Twenty First IFAC World Congress, Berlin, Germany, July 12-17, 2020 (Virtual).<br />Bhusal, S., Bhattarai, U., and Karkee, M. 2019. Improving Pest Bird Detection in a Vineyard Environment Using Super-Resolution and Deep Learning. IFAC-PapersOnLine, 52(30), 18-23.<br />Fu, H., J. Duan, M. Karkee, L. He, H. Xia, J. Li and Q. Zhang. 2019. Effect of shaking amplitude and capturing height on mechanical harvesting of fresh market apples. IFAC-PapersOnLine, 52(30), 306-311.<br />Majeed, Y., Karkee, M., Zhang, Q. Fu, L. and Whiting, M.D. 2019. A study on the detection of visible parts of cordons using deep learning networks for the automated green shoot thinning in vineyards. IFAC-PapersOnLine, 52(30), pp.82-86.<br />Ranjan, R., R. Sinha, L. R. Khot, G. A. Hoheisel, M. Grieshop and M. Ledebhur. 2020. Effect of emitter modifications on spraying attributes of a pneumatic spray delivery based solid set canopy delivery system configured for high-density apple orchard. Paper No. 2000164, ASABE 2020 Virtual Annual International Meeting, July 12-15, 2020 (Oral Presentation).<br />Ranjan, R., L. R. Khot, R. T. Peters, and M. R. Salazar-Gutierrez. 2020. Field evaluation of visible-infrared and microclimate sensing aided crop physiology sensing system for apple sunburn management. Paper No. 2000165, ASABE 2020 Virtual Annual International Meeting, July 12-15, 2020 (Oral Presentation).<br />You, A., F. Sukkar, R. Fitch, M. Karkee, and J.R. Davidson. 2020. An efficient planning and control framework for pruning fruit trees. IEEE International Conference on Robotics and Automation. May 31 – Aug 31, 2020 (Virtual).<br />Zhang, Q. 2019. Digital Agriculture: Opportunities and Challenges, A View of Automation. WSU Digital Agriculture Summit, December 4-6, 2020 (Invited Keynote Speech).<br />Zhang, X., Fu, L., Karkee, M., Whiting, M. D., & Zhang, Q. 2019. Canopy segmentation using ResNet for mechanical harvesting of apples. IFAC-PapersOnLine, 52 (30), 306-311.</p><br /> <p><br />Other Products<br />Khot, L., R. Sinha, G.-A. Hoheisel, and Matthew Grieshop. 2019. Solid set canopy delivery system for WA vineyards. Washington State University - Viticulture and Enology Extension News, Spring 2019. <a href="http://wine.wsu.edu/extension/viticulture-enology-news-veen/">http://wine.wsu.edu/extension/viticulture-enology-news-veen/</a>.</p><br /> <p><br />West Virginia<br />Kirkpatrick, D., Rice, K., Ibrahim, A. Fleischer, S., Tooker, J., Tabb, A., Medeiros, H., Morrison, W., Leskey, T. 2020. The Influence of Marking Methods on Mobility, Survivorship, and Field Recovery of Halyomorpha halys (Hemiptera: Pentatomidae) Adults and Nymphs. Environmental Entomology, nvaa095, https://doi.org/10.1093/ee/nvaa095 .<br />Zhu, J., Teolis, S., Biassou, N., Tabb, A., Jabin, P., and Lavi, O. 2020. Tracking the adaptation and compensation processes of patients brain arterial network to an evolving glioblastoma.IEEE Transactions on Pattern Analysis & Machine Intelligence (accepted, currently preprint).<br />Feldmann, M. J., Hardigan, M. A., Famula, R. A., López, C. M., Tabb, A., Cole, G. S., Knapp, S. J. 2020. Multi-Dimensional Machine Learning Approaches for Fruit Shape Phenotyping in Strawberry. GigaScience. <a href="https://doi.org/10.1093/gigascience/giaa030">https://doi.org/10.1093/gigascience/giaa030</a></p><br /> <p><br />Code releases<br />Tabb, A. “Data and Code from: Using cameras for precise measurement of two-dimensional plant features: CASS.” Zenodo, 2020. http://doi.org/10.5281/zenodo.3677473<br />Tabb, A. and Feldmann, M. J. "Data and Code from: Calibration of Asynchronous Camera Networks: CALICO," (Version v.1) [Data set]. Zenodo, 2019. http://doi.org/10.5281/zenodo.3520866</p>Impact Statements
- Washington - Labor shortage and work-induced safety are two of major challenges in Washington State agriculture. Washington State University (WSU) team has focused on developing mechanization and automation solutions for mechanical harvest of apples for fresh market, and also worked closely with equipment manufacturers to support technology transfer from research to products. For example, WSU team has collaborated with FFRobotics Inc. (Israel) in developing and testing a full scale (12-armed) robotic machine for apple picking. In the test with planner apple trees in WA, it achieved a 100% accuracy in detecting fruit in canopies with a machine vision system and about 70% success rate in picking. Growers witnessed field tests have shown their willingness to adopt a robotic picking system like this one as it can perform the majority of picking job to allow growers maintain a small proportion of current workforce necessary for apple harvesting. The result is promising for commercial adoption of such a robotic picking system in near future, which will make a huge positive impact to apple industry to minimize the need and cost associated with farm labor and improve long term sustainability of the industry. In addition, the WSU team have also developed and tested an alternative approach of fresh market apple harvesting using a targeted shake-and-catch harvesting system. This system has much higher throughput than a robotic picking system and can achieve more than 90% fruit picking rate while keeping fruit damage about 10% for some varieties such as Jazz and Fuji. The tree fruit industry has recognized the potential of this complementary solution for apple harvesting (there was good coverage of this technology in Good Fruit Growers Magazine about a year ago) and the design concept was award ‘2019 Rainbird Engineering Concept of the Year Award’ by American Society of Agricultural and Biological Engineers.
Date of Annual Report: 09/20/2021
Report Information
Period the Report Covers: 10/01/2020 - 09/30/2021
Participants
Mark Siemens, University of Arizona;Pedro Andrade, University of Arizona;
Irwin Gonzalez, University of California, Davis;
Farzaneh Khorsandi, University of California, Davis;
Stavros Vougioukas, University of California, Davis;
Andre Daccache, University of California, Davis;
Yu Jiang, Cornell University;
Won Sun (Daniel) Lee, University of Florida;
Yiannis Ampatzidis, University of Florida;
Daniel Guyer, Michigan State University;
Filip To, Mississippi State University;
Alex Thomasson, Mississippi State University;
Clark Seavert, Oregon State University;
Joseph Dvorak, University of Kentucky;
Ning Wang, Oklahoma State University;
Daeun Choi, Pennsylvania State University;
Paul Heineman, Pennsylvania State University;
Long He, Pennsylvania State University;
Ganesh Bora, USDA-NIFA;
Qin Zhang, Washington State University;
Manoj Karkee, Washington State University;
Lav Khot, Washington State University;
Hao Gan, University of Tennessee
Brief Summary of Minutes
Meeting Summary:
11:00 am: Meeting call to order (Long He)
11:05 am: Member introduction
11:20 am: Business meeting
- Summary of 2020 meeting/reports
- Available on the NIMSS
- Approved by the committee
- 2022 meeting location and time
- Potential options:
- California, hosted by UC Davis group, mid-June (tentative), 2022
- Tentatively targeted at a few days after 10th of June
- It is planned to be a two-day in-person meeting
- Motion made by Stavros, seconded by Ning, approved by the committee
- Dana Choi, Qin Zhang, and UC Davis host team will work together in planning 2022 meeting
- New officer nomination/election
- Farzaneh Khorsandi of UC Davis has been nominated then elected as the new Secretary in 2022
- Yu Jiang of Cornell University will serve as the new Vice Chair in 2022
- Dana Choi of Pennsylvania State University will serve as the new Chair in 2022
- Paul made motion and Alex seconded,
- New announcements and opportunities of collaboration
- Farm of the Future RFA
- Consider inviting NIFA and/or NSF national program leaders to share information on potential funding opportunities in future meetings
- Ganesh Bora: NIFA is working on a workshop/projects related to robot safety (e.g., robot operation safety, potential concerns on robot to human safety)
- PSU: mushroom harvesting SCRI
- WSU: AI Institute (Contact: Qin Zhang)
- UC Davis: Workshop on emerging technologies addressing grand challenges in the produce industry, (January 18-20, 2022, Contact: Irwin Gonzalez)
- UC Davis: opportunities on robot safety and human health with cobot system (Contact: Farzaneh Khorsandi)
- WSU: Special issue in Journal of Field Robotics for agriculture robots (Contact: Manoj Karkee)
- MSU: new positions at USDA-ARS in Michigan and MSU (Contact: Daniel Guyer)
- Annual Report
- Written report of participating institutes for 2021 is due one month from the date of this meeting to Long He (current Chair)
- Long He will share 2020 report to the group as sample and format template
- Report from participating institutes should be concise and should emphasize accomplishments and impacts
- Long He will compile the report, send it to Qin Zhang (Admin advisor) 30 days after the meeting. Qin will review, edit and finalize the report with admin staffs and submit it to NIFA before the deadline
- Other issues
- Manoj Karkee share the news with the group: Abundant Robotics has discontinued its business on developing and commercializing apple harvesting robots mainly due to funding limitations. The company plans to sell its IPs on the technology to other interested potential buyer(s).
- WSU team is working with and will continue to collaborate with FF Robotics in developing practical robotic solutions (and hopeful with a final marketable product) for robotic harvest of fresh market apples
- Robot cost is still higher than labor cost
12:30 pm: State report presentations by participating institutes
16:15 pm: State report presentations completed
16:30 pm: Meeting adjourned
Accomplishments
<h2>Arizona</h2><br /> <ul><br /> <li>A novel, energy efficient band-steam applicator for controlling soilborne pests was developed and tested. Trial results showed that band-steam reduced fusarium wilt and lettuce drop disease incidence by >70%, improved weed control by > 85%, reduced hand weeding labor requirements by roughly 30% and increased crop yield increases trends of 24% as compared to the untreated control. Machine work rate (0.5 ac/hr) and energy costs ($325/ac) were considered reasonable in field conditions where initial soil temperature was high (>110 °F).</li><br /> <li>On-going field research with vegetable grower cooperators is focused on characterizing the spatial distribution of soil attributes that influence the ability of soil to retain and make available nitrogen applied as fertilizer to vegetable crops during the early phases of growth development. Results have shown that tailored variable-rate applications can reduce the overall rate of nitrogen fertilizer by as much as 40%. Results are shared with vegetable growers during Extension events and grower meetings.</li><br /> </ul><br /> <h2>California</h2><br /> <ul><br /> <li>A prototype of an inline moisture estimation system for green coffee using time-domain reflectometry (TDR) was developed. The system can automatically estimate the moisture content of green coffee. Future work will evaluate the implementation of this system in large-scale coffee dry mill facilities.</li><br /> <li>A smart machine was developed and deployed that can automatically perform the following tasks: create a deaerated tomato juice sample, sequentially perform color, soluble solids content and pH measurements of the juice, electronically transfer the measurement results to a statewide database, dispose of the tomato juice sample, and clean itself. The fully automated procedure ensures that the inspection process is conducted equitably and reliably statewide and eliminates data transcription errors associated with manual data entry. A fleet of 30 of these smart machines were designed, fabricated and deployed in the state of California. The machines were installed at each and every processing tomato facility in California in the 2021 production season. </li><br /> <li>A semi-automated almond weighing system was developed to measure individual tree yields, during commercial harvesting. The system consists of a custom designed funnel-shaped bin, that hangs from an aluminum frame from four load cells. At the bottom of the bin, a trap door is actuated by a hydraulic cylinder, at the push of a button, controlled by the machine operator. The bin can attach to existing harvesters, and specifically, to the receiver-vehicle of a shaker-receiver pair of vehicles. During shaking, the almonds are collected on the catching frames of the shaker and receiver units and diverted to a conveyor belt, which transports the almonds inside the bin. Once all the almonds of a given tree are collected inside the bin, their weight is measured, and the trap door releases them on the ground to empty the bin and prepare for the next tree-yield measurement. An RTK-GPS records the bin’s position, so that yield can be associated with individual trees, given a pre-existing map of the orchard. A custom-build data acquisition unit collects and stores all data. The system was built, calibrated and tested, and deployed in a commercial almond orchard in California.</li><br /> <li>An autonomous ATV was developed to evaluate the performance of roll bars in the agricultural ATV incidents. In addition, a sensing setup and simulation models to evaluate the youth capabilities to ride ATV on farms, were developed. Our long-term goal is to reduce the likelihood of ATV-related fatalities and injuries of youth in the agriculture industry. The proposed study is in response to the need, as outlined by several agencies, to scientifically measure physical and ergonomic factors that if lacking may put youth at risk of injury or death while operating utility ATVs. The study will provide critical evidence that contributes to the scientific bases for modifying regulatory/advisory guidelines, and state and national policy for operating ATVs. In this project, we identify potential strength and anthropometric discrepancies between the requirements for operating utility ATVs and the physical characteristics and strength of youths of varying ages and height percentiles.</li><br /> <li>An agricultural sensing kit (georeferenced RGB + depth imagery) that easily mounts on the front of a tractor and automatically turns on and off to sense the canopy during routine operations like spraying and sulfur dusting was developed. The system is used for early-season yield estimation in grape and almond. Future work will deploy multiple sensing kits during the growing season.</li><br /> </ul><br /> <h2>New York</h2><br /> <ul><br /> <li>Ground autonomous robot for versatile vineyard research and management: A ground autonomous robot was developed with a reconfigurable mounting structure for various sensors. The robot is equipped with an RTK-GPS and IMU to provide GNSS-INS-based navigation. The robot was tested in multiple research vineyards for automated data collection in 2021.</li><br /> <li>Development and testing of an AI-based imaging system for grape downy mildew monitoring: Developed a deep learning-based image analysis pipeline that can process RGB images collected using a custom stereo camera with strobe light illumination for grape downy mildew detection and quantification.</li><br /> <li>Evaluation of an autonomous robotic carriage and UV-C lamp arrays light for plant disease suppression: We used an autonomous robotic carriage and UV-C lamp array to suppress diverse grapevine diseases, including powdery mildew (Erysiphe necator) and sour rot (a complex of bacteria, insects, and fungi). The robot was deployed in both a research vineyard at Cornell AgriTech (Geneva, NY) and a commercial vineyard in Penn Yan, NY. UV-C at 100 to 200 J/m**2, either once or twice weekly provided suppression equivalent to that provided by many conventional pesticides. In parallel trials, the same technology suppressed powdery mildew on strawberry and cucurbits, fire blight on apple, and Cercospora leaf spot on beets. However, UV-C provided only minimal suppress of the Oomycete pathogen causing grapevine downy mildew (Plasmopara viticola). The foregoing experiments were expanded in 2021 to include cooperators in FL, CA, OR, WA, Canada (Nova Scotia), Great Britain, Italy, and Norway.</li><br /> </ul><br /> <h2>Florida</h2><br /> <ul><br /> <li>A strawberry plant wetness detection system has been developed using color imaging and deep learning for strawberry production. Leaf wetness duration is an important measure to determine the risks of disease. The system could replace currently used commercial plant wetness sensors, which are unreliable and hard to calibrate.</li><br /> <li>A smartphone-based tool was developed to detect and count two-spotted spider mites (TSSM) on strawberry plants. A deep learning method, You Look Only Once (YOLO), was used to detect TSSM and eggs, and a mean average precision of 0.65 was obtained.</li><br /> <li>A strawberry bruise detection system for postharvest fruit quality evaluation was developed utilizing machine vision and deep learning.</li><br /> <li>A disease detection and monitoring system was developed for downy mildew in watermelon utilizing UAV-based hyperspectral imaging and machine learning. This technique was able to classify several severity stages of the disease.</li><br /> <li>A yield and related traits prediction system was developed for wheat under heat-related stress environments. This high-throughput system utilizes UAV-based hyperspectral imaging and machine learning. A yield prediction system was developed for citrus too utilizing UAV-based multispectral imaging and AI.</li><br /> <li>A system was developed to determine leaf stomatal properties in citrus trees utilizing machine vision and artificial intelligence.</li><br /> <li>A machine vision based system was developed to measure pecan nut growth utilizing deep learning for the better understanding of the fruit growth curve.</li><br /> </ul><br /> <h2>Kentucky</h2><br /> <ul><br /> <li>An automated guidance and weed control study was performed at the University of Kentucky in kale. Horticulturalists at UK have developed machinery recommendations for organic crops in non-plasticulture growing systems. This study used the previously developed machinery field operations (bed preparation, drip tape laying, bed forming, transplanting, weeding), but considered the impact of using high precision RTK automated guidance for tractor control. With precision guidance for the tractor, it was possible to eliminate (with acceptable levels of crop damage) the requirement for secondary guidance on the weeding implement.</li><br /> </ul><br /> <h2>Mississippi</h2><br /> <ul><br /> <li>Plastic Contaminant Sensor for Ginning Contaminant-Free Cotton: We are applying our sensor technology for specialty crop into a real-time system for detecting plastic contaminant in seed cotton (raw cotton) using machine learning algorithms. We integrate this sensor with a separator system designed to segregate contaminated cotton from “clean” cotton in a stream in real-time. The impact of this technology will include production of contaminant-free cotton which has better market value and preserve good reputation of producers.</li><br /> <li>Inline Moisture monitoring system: We are incorporating capacitive sensor technology to monitor moisture of cotton stream in an inline real-time fashion to produce control data for automating drying systems inside a cotton gin. The impact of this technology will include a higher efficiency ginning process and the production of higher quality cotton.</li><br /> </ul><br /> <h2>Michigan</h2><br /> <ul><br /> <li>Indirect collaboration on trials and testing continue with a U.S. specialty crops importer and also an international technology entity toward the commercial development and implementation of utilizing the concepts of application of Computed Tomography developed at Michigan State University for evaluation of internal quality of fresh chestnut. Through these entities, electronic sorting and measurement experience, including the development of new high-speed CT imaging, has been brought to the table and is involving testing and data analysis algorithm development focused on internal chestnut defect/quality assessment. Advancement has been promising to date with continued testing planned this season. The effort to date has been worked on or investigated with a faculty-student extracurricular engineering group and Mechanical Engineering colleagues interested in sustainable agriculture solutions. Possible external proposal opportunities have also been identified and will likely be pursued. At least one Mechanical Engineering Capstone Design team is planned to work on this project in this fiscal year.</li><br /> </ul><br /> <h2>Oklahoma</h2><br /> <ul><br /> <li>We conducted an experiment on using X-ray imaging techniques to detect peanut smut damages. As peanut with smut fungus could not be found in the US, our collaborator at USAD ARS made “fake peanut” samples for the tests using similar materials to the smut. The result showed a clear correlation between features extracted from the x-ray images and fake smut damaged peanuts. Based on the results, we designed an X-ray imaging system for peanut smut detection. The mechanical design for an X-ray Imaging system was completed and the system is being constructed. The outcome of this work will be an automated, x-ray imaging system for peanut smut detection. The system can also be used for other nuts on damage detection with revisions on image processing and analysis algorithms.</li><br /> </ul><br /> <h2>Oregon</h2><br /> <ul><br /> <li>A recent study evaluated labor-saving machines in the wine grape industry with the aim of preserving and enhancing fruit and wine quality while using automation. Four vineyard tasks were evaluated that field data was readily available from growers, and the technology had the highest near-term chance of success. The four tasks were 1) precision pruning, 2) shoot thinning and desuckering, 3) leaf pulling, and 4) mechanical harvesting.</li><br /> </ul><br /> <h2>Pennsylvania</h2><br /> <ul><br /> <li>A LoRaWAN based Internet of things (IoT) platform was developed for the soil moisture based precision irrigation system. The system was tested in a peach orchard, and the results indicated that the developed precision irrigation system can monitor the real-time (at one minute frequency) soil moisture levels and control the valve automatically to apply water to the crops based on the soil moisture levels.</li><br /> <li>An inlet airflow control system was developed for the precision sprayer to control the airflow speed based on the canopy density. In the system, a 3D LiDAR was used to measure the canopy density, and an iris damper was mounted at the rear of the sprayer fan to adjust the inlet airflow. The system was tested and validated in the field condition with various tree canopy densities, and has been proven to be effective for precise spraying and drifting reduction.</li><br /> <li>A series of green fruit removal dynamic tests were conducted for a few apple cultivars, and the required fruit removal forces were recorded. Then a green fruit removal end-effector was developed and tested with both a handheld mechanism and a robotic manipulator. This study provided guideline information for developing a robotic green fruit thinning system.</li><br /> <li>To develop a robotic precision pollination system, a Mask R-CNN based detection algorithm was developed to identify the king flowers in the apple trees. The developed algorithm was able to detect and locate apple king flowers with the accuracy range from 60%-91% depending on different flowering stage.</li><br /> <li>The RootRobot for maize root phenotyping was field tested in Fall 2020. Issues with robustness were addressed. Upgrades were completed in the lab in Winter, Spring, and early Summer 2021. The ability to handle multiple stalks for processing was added. Field tests in Summer 2021 revealed issues with sensor light sensitivity and also the unit’s ability to excavate and grab the corn stalks. An imaging chamber was developed, tested, and upgraded for taking images from 10 cameras at 1 degree increments, resulting in 3600 images per stalk. These are used for developing 3-D models of the root architecture.</li><br /> <li>A computational fluid dynamics model of orchard heating was created for the CPS frost project. This links with the UAV sensing and communication to ground robots that would carry the heaters to cold locations within the orchard.</li><br /> <li>A camera system with active lighting was developed to overcome varying outdoor conditions. There was substantial improvement in image brightness and color consistency by using the LED flashes. Images captured by the prototype system during an 11-hour period showed an average decrease of 85% in standard deviation for the Hue-Saturation-Value (HSV) channels compared to that of the auto-exposure setting. Additionally, the prototype system was able to fix motion blur in images averaging 7 mm in error for a stereo vision application with the camera moving at 7 km/hr.</li><br /> <li>Heating requirement assessment methodology for frost protection in an apple orchard was developed utilizing UAV-based thermal and RGB cameras. A thermal image stitching algorithm using BRISK feature was developed for creating georeferenced orchard temperature maps, which attained a sub-centimeter map resolution and a stitching speed of 100 thermal images within 30 seconds. YOLOv4 classifiers for six apple flower bud growth stages in various network sizes were trained based on 5040 RGB images, and the best model achieved a 71.57% mAP for a test dataset consisted of 360 images.</li><br /> <li>We developed a UAV-LiDAR system employing UAV’s built-in navigation units. A novel approach for registering UAV-LiDAR data of level agricultural fields was developed utilizing a colored iterative closest point (ICP) algorithm and GNSS location and IMU orientation information from the UAV. The proposed algorithm was tested in a peach tree parameter estimation experiment. Using manually measured crown widths in two perpendicular dimensions and heights of 11 trees as evaluation metrics, our proposed algorithm achieved a root mean square error (RMSE) range of 0.05 to 0.2 m depending on the tree parameter and flight altitude, and it was able to register tree point clouds up to 67% more accurately in terms of the extracted tree parameters than the georeferencing method.</li><br /> </ul><br /> <h2>Washington</h2><br /> <ul><br /> <li>Developed a trellis wire detection technique for a 12-armed robotic apple harvester and continued field evaluation of the harvester in commercial orchards.</li><br /> <li>Developed a machine-vision system for detecting flower clusters (with 86% accuracy) and estimating flower density (with 84% accuracy) in apple and cherry orchards, which will be used in developing an automated thinning and pollination systems.</li><br /> <li>Further developed a machine-vision (using a tracking camera) and robotic system for pruning apple trees, which was tested in the laboratory environment with actual apple trees brought from the field.</li><br /> <li>Improved the design of the automated green shoot thinning machine and built the second version of the prototype (larger and faster than first prototype; installed in a gator for continuous operation) which has been tested in the lab environment.</li><br /> <li>Developed a machine vision and end-effector technique for robotic pollination of apples.</li><br /> <li>Optimized and refined fixed spray system (i.e. solid set chemical delivery system) configurations capable of spraying longer loop lengths using larger sized reservoirs, and conducted pertinent field validation in tree fruit orchards and spray deposition/coverage comparisons with conventional airblast sprayers. Added automation capabilities to operate & monitor system performance via mobile application.</li><br /> <li>Improved and conducted field testing of an Internet-of-Things (IoT) enabled Crop Physiology Sensing System (CPSS 3.0) for apple fruit surface temperature monitoring and real-time actuation of modified evaporative cooling system for heat stress management in fresh market apple cultivars (cv Honeycrisp and Cosmic Crisp). CPSS 3.0 has been refined to extract other canopy/fruit quality attributes to aid grower decision support.</li><br /> <li>Developed aerial imagery (multispectral and thermal) based spectral sensing integrated energy balance modeling approach to estimate evapotranspiration (ET) at high spatial resolution (10 cm/pixel). Validate the approach for ET and leaf level transpiration (aka crop water use) estimation in grapevine, apple and field crops (mint, alfalfa, potato).</li><br /> <li>Developed and validated tree-row-volume and unit-canopy-ration driven base spray rate estimation approaches for an intelligent orchard sprayer to perform efficient spray applications in tall spindle apple crop canopies.</li><br /> </ul>Publications
<h2>Arizona</h2><br /> <p>Siemens, M.C., Godinez, Jr., V. & Gayler, R.R. 2021. Centimeter Scale Resolution Spot Sprayer for Precision In-Row Weed Control. In Proc. 73rd Annual California Weed Science Society 73:44. Salinas, Calif.: California Weed Science Society.</p><br /> <p>Siemens, M.C. Godinez, Jr., V., Bahr, N. & Fennimore, S.A. 2021. Development and evaluation of a novel band-steam applicator for controlling soilborne pathogens and weeds in lettuce. ASABE Paper No. 2100185. St. Joseph, Mich.: ASABE.</p><br /> <h2>California</h2><br /> <p>Rotta, N.M., Curry, S., Han, J., Reconco, R., Spang, E., Ristenpart, W., & Donis-González, I.R. 2021. A comprehensive analysis of operations and mass flows in postharvest processing of washed coffee. Resources, Conservation and Recycling 170: 105554.</p><br /> <p>Félix-Palomares, L., & & Donis-González, I.R. 2021. Optimization and Validation of Rancimat Operational Parameters to Determine Walnut Oil Oxidative Stability. Processes 9 (4): 651.</p><br /> <p>Kilinya Mayanja, I., Coates, M.C., Niederholzer, F., & Donis-González, I.R. 2021. Development of a Stockpile Heated and Ambient Air Dryer (SHAD) for Freshly Harvested Almonds. Applied Engineering in Agriculture. 37(3): 417-425. (doi: 10.13031/aea.14364).</p><br /> <p>Donis-González, I.R., Bergman, S.M., Sideli, G.M. ,Slaughter, D.C., & Crisosto, C.H. 2020. Color vision system to assess English walnut (Juglans Regia) kernel pellicle color. Postharvest Biology and Technology 167 (111199): 1-11.</p><br /> <p>Su, W-H, Fennimore, S.A., Slaughter, D.C. 2020. Development of a systemic crop signalling system for automated real-time plant care in vegetable crops. Biosystems Engineering. Vol. 193: 62-74. </p><br /> <p>Raja, R., Nguyen, T.T., Vuong, V.L., Slaughter, D.C., Fennimore, S.A., 2020. RTD-SEPs: Real-time detection of stem emerging points and classification of crop-weed for robotic weed control in producing tomato. Biosystems Engineering. Vol. 195: 152-171.</p><br /> <p>Arikapudi R., Vougioukas, S.G. (2021). Robotic Tree-fruit Harvesting with Telescoping Arms: A study of Linear Fruit Reachability under Geometric Constraints. IEEE Access. (9): 17114-17126 https://doi.org/10.1109/ACCESS.2021.3053490</p><br /> <p>Fei, Z., Vougioukas, S.G. (2021). Co-Robotic Harvest-aid Platforms: Real-time Control of Picker Lift Heights to Maximize Harvesting Efficiency. Computers and Electronics in Agriculture. (180): 105894. https://doi.org/10.1016/j.compag.2020.105894</p><br /> <p>Araujo, G. D. M., Khorsandi, F., & Abdullah, A. (2021). Ability of Youth to Activate Agricultural All-Terrain Vehicles’ Main Controls. In 2021 ASABE Annual International Virtual Meeting (p. 1). American Society of Agricultural and Biological Engineers.</p><br /> <p>Araujo, G. D. M., Khorsandi, F., Kabakibo, S., & Kreylos, O. (2021). Can youth reach agricultural all-terrain vehicle controls? In 2021 ASABE Annual International Virtual Meeting (p. 1). American Society of Agricultural and Biological Engineers.</p><br /> <p>Khorsandi, F., Ayers, P. D., Myers, M., Oesch, S., & White, D. J. (2021). Engineering Control Technologies to Protect Operators in Agricultural All-Terrain Vehicle Rollover Incidents. Journal of Agricultural Safety and Health, 0.</p><br /> <p>Khorsandi, F., Ayers, P., Denning, G., Jennissen, C., Jepsen, D., Myers, M., ... & White, D. J. (2020). Hazard control methods to improve agricultural all-terrain vehicle safety. Journal of agromedicine, 1-16.</p><br /> <p>Khorsandi, F., Ayers, P., Denning, G., Jennissen, C., Jepsen, D., Myers, M., ... & White, D. J. (2020). Hazard control methods to improve agricultural all-terrain vehicle safety. Journal of agromedicine, 1-16.</p><br /> <p>Chou, H. Y., Khorsandi, F., & Vougioukas, S. G. (2020). Developing and testing a gps-based steering control system for an autonomous all-terrain vehicle. In 2020 ASABE Annual International Virtual Meeting (p. 1). American Society of Agricultural and Biological Engineers.</p><br /> <p>Fei, Z., Olenskyj, A., Bailey, B., and Earles. J.M. (accepted). Enlisting 3D crop models and GANs for more data efficient and generalizable fruit detection. International Conference on Computer Vision (ICCV), 7th workshop on Computer Vision in Plant Phenotyping and Agriculture.</p><br /> <h2>New York</h2><br /> <p>Ertai Liu, Kaitlin M Gold, David Combs, Lance Cadle-Davidson, Yu Jiang. 2021. Deep Learning-based Autonomous Downy Mildew Detection and Severity Estimation in Vineyards. 2021 ASABE Annual International Virtual Meeting, Paper # 2100486. doi:10.13031/aim.202100486.</p><br /> <p>Rodrigo Borba Onofre, David M Gadoury, Arne Stensvand, Andrew Bierma, Mark S Rea, and Natalia A. Peres. 2021. Use of Ultraviolet Light to Suppress Powdery Mildew in Strawberry Fruit Production Fields. Plant Disease. https://doi.org/10.1094/PDIS-04-20-0781-RE</p><br /> <h2>Florida</h2><br /> <p><strong>Publications </strong></p><br /> <p>Costa L., McBreen J., Ampatzidis Y., Guo J., Reisi Gahrooei M., Babar A., 2021. Using UAV-based hyperspectral imaging and functional regression to assist in predicting grain yield and related traits in wheat under heat-related stress environments for the purpose of stable yielding genotypes. Precision Agriculture (accepted).</p><br /> <p>Costa L., Ampatzidis Y., Rohla C., Maness N., Cheary B., Zhang L., 2021. Measuring pecan nut growth utilizing machine vision and deep learning for the better understanding of the fruit growth curve. Computers and Electronics in Agriculture, 181, 105964, <a href="https://doi.org/10.1016/j.compag.2020.105964">doi.org/10.1016/j.compag.2020.105964</a>.</p><br /> <p>Costa L., Archer L., Ampatzidis Y., Casteluci L., Caurin G.A.P., Albrecht U., 2021. Determining leaf stomatal properties in citrus trees utilizing machine vision and artificial intelligence. Precision Agriculture 22, 1107-1119, <a href="https://doi.org/10.1007/s11119-020-09771-x">https://doi.org/10.1007/s11119-020-09771-x</a>.</p><br /> <p>Kim, W.-S., D.-H. Lee, Y.-J. Kim, T. Kim, W. S. Lee, and C.-H. Choi. 2021. Stereo-vision-based crop height estimation for agricultural robots. Computers and Electronics in Agriculture 181: 105937. <a href="https://doi.org/10.1016/j.compag.2020.105937">https://doi.org/10.1016/j.compag.2020.105937</a>. </p><br /> <p>Kim, W. S., W. S. Lee, and Y. J. Kim. 2020. A Review of the applications of the Internet of Things (IoT) for agricultural automation. J. Biosyst. Eng. 45: 385–400. <a href="https://doi.org/10.1007/s42853-020-00078-3">https://doi.org/10.1007/s42853-020-00078-3</a>.</p><br /> <p>Nunes L., Ampatzidis Y., Costa L., Wallau M., 2021. Horse foraging behavior detection using sound recognition techniques and artificial intelligence. Computers and Electronics in Agriculture, 183, 106080, <a href="https://doi.org/10.1016/j.compag.2021.106080">doi.org/10.1016/j.compag.2021.106080</a>.</p><br /> <p>Swarup, A., W. S. Lee, N. Peres, and C. Fraisse. 2020. Strawberry plant wetness detection using color and thermal imaging. J. of Biosystems Engineering. 45: 409-421. <a href="https://doi.org/10.1007/s42853-020-00080-9">https://doi.org/10.1007/s42853-020-00080-9</a>. </p><br /> <p>Uyeh, D. D., J. Kim, S. Lohumi, T. Park, B.-K. Cho, S. Woo, W. S. Lee, and Y. Ha. 2021. Rapid and non-destructive monitoring of moisture content in livestock feed using a global hyperspectral model. Animals 11, 1299. <a href="https://doi.org/10.3390/ani11051299">https://doi.org/10.3390/ani11051299</a>.</p><br /> <p>Vijayakumar V., Costa L., Ampatzidis Y., 2021. Prediction of citrus yield with AI using ground-based fruit detection and UAV imagery. 2021 Virtual ASABE Annual International Meeting, July 11-14, 2021, 2100493, doi:10.13031/aim.202100493.</p><br /> <p>Xie, C. and W. S. Lee. 2021. Detection of citrus black spot symptoms using spectral reflectance. Postharvest Biology and Technology 180: 111627. <a href="https://doi.org/10.1016/j.postharvbio.2021.111627">https://doi.org/10.1016/j.postharvbio.2021.111627</a>.</p><br /> <p>Zhou, C., W. S. Lee, O. E. Liburd, I. Aygun, J. K. Schueller, and I. Ampatzidis. 2021. Smartphone-based tool for two-spotted spider mite detection in strawberry. ASABE Paper No. 2100558. St. Joseph, MI.: ASABE.</p><br /> <p>Zhou, X., Y. Ampatzidis, W. S. Lee, and S. Agehara. 2021. Postharvest strawberry bruise detection using deep learning. ASABE Paper No. 2100458. St. Joseph, MI.: ASABE.</p><br /> <p><strong>Presentations</strong></p><br /> <p>Abdulridha J., Ampatzidis Y., Qureshi J., Batuman O., Kakarla S., 2021. Detecting and monitoring the progress of downy mildew disease in watermelon by utilizing UAV–based hyperspectral imaging and machine learning. 2021 Virtual ASABE Annual International Meeting, July 11-14, 2021.</p><br /> <p>Adosoglou G., Park S., Ampatzidis Y., Pardalos P., 2021. A high-level task planning of autonomous robots with multi-dimensional loading constraints for precision weed management under field variability. 2021 Virtual ASABE Annual International Meeting, July 11-14, 2021.</p><br /> <p>Ampatzidis Y., 2021. AI applications in specialty crops. 2021 Virtual ASABE Annual International Meeting, Special Session - Processing Systems AI and Data Science Application in Food and Biological Material Processing, July 11-14, 2021.</p><br /> <p>Ampatzidis Y., 2021. Automation, artificial intelligence and robotics in strawberry production. 9th International Strawberry Symposium (ISHS – ISS2021), May 1-5, 2021. Keynote Speaker.</p><br /> <p>Costa L., Ampatzidis Y., Shukla S., 2021. Citrus fruit maturity prediction utilizing UAV multispectral imaging and machine learning. 2021 Virtual ASABE Annual International Meeting, July 11-14, 2021.</p><br /> <p>Vijayakumar V., Archer L., Ampatzidis Y., Albrecht U., Batuman O., 2021. An automated delivery system for therapeutic materials using needle-based trunk injection to treat HLB affected Citrus. 2021 Virtual ASABE Annual International Meeting, July 11-14, 2021.</p><br /> <p>Vijayakumar V., Partel V., Ampatzidis Y., Silwal A., Kantor G., 2021. Autonomous smart sprayer for precision weed management using machine vision and AI. 2021 Virtual ASABE Annual International Meeting, July 11-14, 2021.</p><br /> <p><strong>Extension-Outreach</strong></p><br /> <p>Dr. Ampatzidis (Invited talks):</p><br /> <p>Emerging Technologies and AI for BMP. UF/IFAS Water Wednesdays. February 24, 2021.</p><br /> <p>Artificial Intelligence in Agriculture. UF EGN1935-FOAI (31730) - Freshman Engineering: Frontiers of AI (virtual lecture). February 9, 2021.</p><br /> <p>Applications of Artificial Intelligence in Precision Agriculture. Central District Ag BMP virtual meeting. February 3, 2021.</p><br /> <p>Drones, artificial intelligence, and the future of pest management in vegetable crops. Annual vegetable growers virtual meeting. The Oregon processed vegetable commission. January 25, 2021.</p><br /> <p>Saving Citrus with NVIDIA. NVIDIA Podcast #75 (<a href="https://www.storagereview.com/podcast/podcast-75-saving-citrus-with-nvidia-ai">https://www.storagereview.com/podcast/podcast-75-saving-citrus-with-nvidia-ai</a>; 45 min), January 2021.</p><br /> <h2>Kentucky</h2><br /> <p>Dvorak, J., Pampolini, L., Jackson, J., Seyyedhasani, H., Goff, B., Sama, M. (2021). Predicting Quality and Yield of Growing Alfalfa from a UAV. Transactions of the ASABE. 64(1): 63-72. doi: 10.13031/trans.13769</p><br /> <p>Minch, C.*, Dvorak, J., Jackson, J., & Sheffield, S. T. (2021). Creating a Field-Wide Forage Canopy Model Using UAVs and Photogrammetry Processing. Remote Sensing, 13(13), 2487. MDPI AG. <a href="http://dx.doi.org/10.3390/rs13132487">http://dx.doi.org/10.3390/rs13132487</a></p><br /> <h2>Mississippi</h2><br /> <p>Lucas Gay, Filip To, Ruixiu Sui: Moisture Determination of Cotton in Static Conditions Via Capacitive Sensing. Belt-wide Cotton Conference Proceedings, Cotton Engineering Systems, #20434, 3.5.2021</p><br /> <p>Joshua Tandio, Filip To , Ruixiu Sui: Bench Top Plastic Contaminant Detection in Cotton Using Deep Learning Neural Network Trained with Images Taken under 4 Lighting Colors, Belt-wide Cotton Conference Proceedings, Cotton Engineering Systems, #20420, 3.5.2021</p><br /> <h2>Oregon</h2><br /> <p>Western FarmPress, April 10, 2019, <a href="https://www.farmprogress.com/grapes/upstart-states-push-precision-viticulture">Startup States Push Precision Viticulture</a></p><br /> <p>Good Fruit Grower, May 22, 2019, The Margins of Mechanization: Oregon State University economist assesses the costs and benefits of mechanizing vineyard tasks. <a href="https://www.goodfruit.com/the-margins-of-mechanization/">The Margins of Mechanization</a></p><br /> <p>OWRI and Washington Wine Commission sponsored webinar, June 11, 2019, titled "Can Mechanizing Vineyard Tasks Make you Money?" <a href="https://media.oregonstate.edu/media/t/1_twc0q2zw">Webinar Recording</a></p><br /> <p>National Grape Research Alliance Newsletter, June 2019: <a href="https://www.agbizlogic.com/static/resources/publications/National%20Grape%20Research%20Alliance%20The%20True%20Cost%20of%20Mechanization.pdf">The True Cost of Mechanization</a></p><br /> <h2>Pennsylvania</h2><br /> <p><strong>Journal Articles</strong></p><br /> <p>Mirbod, O., Choi, D., Thomas, R. and He, L. 2021. Overcurrent-driven LEDs for consistent image colour and brightness in agricultural machine vision applications. Computers and Electronics in Agriculture, 187, 106266.</p><br /> <p>Yuan, W., & Choi, D. (2021). UAV-Based Heating Requirement Determination for Frost Management in Apple Orchard. Remote Sensing, 13(2), 273.</p><br /> <p>Zahid, A., He, L., Choi, D., Schupp, J. and Heinemann, P. 2021. Investigation of Branch Accessibility with a Robotic Pruner for Pruning Apple Trees. Transactions of the ASABE, 64(5).</p><br /> <p>Zahid, A., Mahmud, M.S., He, L., Heinemann, P., Choi, D. and Schupp, J. 2021. Technological advancements towards developing a robotic pruner for apple trees: A review. Computers and Electronics in Agriculture, 189, 106383.</p><br /> <p>Huang, M., He, L., Choi, D., Pecchia, J. and Li, Y. 2021. Picking dynamic analysis for robotic harvesting of Agaricus bisporus mushrooms. Computers and Electronics in Agriculture, 185, 106145.</p><br /> <p>Huang, M., Jiang, X., He, L., Choi, D., Pecchia, J. and Li, Y. 2021. Development of A Robotic Harvesting Mechanism for Button Mushrooms. Transactions of the ASABE, 64(2), 565-575.</p><br /> <p>Mahmud, M.S., Zahid, A., He, L., Choi, D., Krawczyk, G., Zhu, H. and Heinemann, P. 2021. Development of a LiDAR-guided section-based tree canopy density measurement system for precision spray applications. Computers and Electronics in Agriculture, 182, 106053.</p><br /> <p>Jiang, X. and He, L. 2021. Investigation of Effective Irrigation Strategies for High-Density Apple Orchards in Pennsylvania. Agronomy, 11(4), 732.</p><br /> <p>Mahmud, M.S., Zahid, A., He, L. and Martin, P. 2021. Opportunities and Possibilities of Developing an Advanced Precision Spraying System for Tree Fruits. Sensors, 21(9), p.3262.</p><br /> <p><strong>Thesis/Dissertations</strong></p><br /> <p>Zahid, Azlan (2021). Development of a robotic manipulator for pruning apple trees. PhD dissertation. August 2021. Pennsylvania State University.</p><br /> <p>Zhang, Haozhe (2021). Internet of things (IoT)-based precision irrigation with LoRaWAN technology applied to vegetable production. MS thesis. May 2021. Pennsylvania State University.</p><br /> <p>Mirbod, Omeed (2021). In-field imaging of apple orchards using stereo vision for improved fruit size and yield estimation. MS thesis. May 2021. Pennsylvania State University.</p><br /> <p><strong>Extension Talks (Long He)</strong></p><br /> <p>Internet of Things (IoT) for Precision Irrigation Management in Tree Fruit Orchards. February 4, 2021. 2021 Cornell NYS Tree Fruit Conference.</p><br /> <p>Precision Irrigation Systems for Tree Fruit Orchards-2020 Season Updates. February 11, 2021. 2021 Mid-Atlantic Fruit and Vegetable Convention.</p><br /> <p>Developing Sensor-Based Smart Irrigation Systems for Vegetable Crops. February 11, 2021. 2021 Mid-Atlantic Fruit and Vegetable Convention.</p><br /> <p>Potential of Using Robotic Systems on Crop Load Management for Apples. On-Line, 234 Participants. (February 22, 2021). 2021 Penn State Extension Winter Fruit School.</p><br /> <h2>Washington</h2><br /> <p><strong>Journal Articles</strong></p><br /> <p>Arai, R., S. Sakai, A. Tatsuoka, and Q. Zhang, 2021. Analytical, experimental, and numerical investigation of energy in hydraulic cylinder dynamics of agriculture scale excavators. Energies, Accepted, In Press, <a href="https://doi.org/10.3390/en1010000">https://doi.org/10.3390/en1010000</a></p><br /> <p>Chandel, A. K., B. Molaei, L. R. Khot, R. T. Peters, C. O. Stöckle, and P. W. Jacoby. 2021. High-resolution spatiotemporal water use mapping of surface and direct-root-zone drip irrigated grapevines using UAS-based thermal and multispectral remote sensing. Remote Sensing, 13, 954. <a href="https://doi.org/10.3390/rs13050954">https://doi.org/10.3390/rs13050954</a></p><br /> <p>Chandel , A. K., B. Molaei, L. R. Khot, R. T. Peters, and C. O. Stöckle. 2020. High resolution geospatial evapotranspiration mapping of irrigated field crops using multispectral and thermal infrared imagery with METRIC energy balance model. Drones, 4(3), 52. <a href="https://doi.org/10.3390/drones4030052">https://doi.org/10.3390/drones4030052</a> </p><br /> <p>Chandel , A., L. R. Khot, and L.-X. Yu. 2021. Alfalfa (Medicago sativa L.) crop vigor and yield characterization using high-resolution aerial multispectral and thermal infrared imaging technique. Computers and Electronics in Agriculture, 182, 105999. <a href="https://doi.org/10.1016/j.compag.2021.105999">https://doi.org/10.1016/j.compag.2021.105999</a></p><br /> <p>Kothawade , G., S. Sankaran, A. A. Bates, B. K. Schroeder and L. R. Khot. 2020. Feasibility of volatile biomarker‐based detection of Pythium leak in postharvest stored potato tubers using field asymmetric ion mobility spectrometry. Sensors, 20(24), 7350. <a href="https://doi.org/10.3390/s20247350">https://doi.org/10.3390/s20247350</a></p><br /> <p>Kothawade, G., A. K. Chandel , L. R. Khot, S. Sankaran, A. A. Bates, and B. Schroeder. 2021. Field asymmetric ion mobility spectrometry for pre-symptomatic rot detection in stored Ranger Russet and Russet Burbank potatoes. Postharvest Biology and Technology, 181, 111679 <a href="https://doi.org/10.1016/j.postharvbio.2021.111679">https://doi.org/10.1016/j.postharvbio.2021.111679</a></p><br /> <p>Majeed, Y., M. Karkee, Q. Zhang, L. Fu, and M.D. Whiting, 2021. Development and performance evaluation of a machine vision system and an integrated prototype for automated green shoot thinning in vineyards. Journal of Field Robotics, 38: 898-916. <a href="https://doi.org/10.1002/rob.22013">https://doi.org/10.1002/rob.22013</a></p><br /> <p>Marzougui, A., Y. Ma, R. J. McGee, L. R. Khot, and S. Sankaran. 2020. Generalized linear model with elastic net regularization and convolutional neural network for evaluating Aphanomyces root rot severity in Lentil. Plant Phenomics, 20, Article ID 2393062. <a href="https://doi.org/10.34133/2020/2393062">https://doi.org/10.34133/2020/2393062</a></p><br /> <p>Marzougui, A., Y. Ma, R. J. McGee, L. R. Khot, and S. Sankaran. 2020. Generalized linear model with elastic net regularization and convolutional neural network for evaluating Aphanomyces root rot severity in lentil [Dataset]. Zenodo. <a href="http://doi.org/10.5281/zenodo.4018168">http://doi.org/10.5281/zenodo.4018168</a> </p><br /> <p>McCoy, M. L., G.-A. Hoheisel, L. R. Khot, and M. M. Moyer. 2021. Assessment of three commercial over-the-row sprayer technologies in Eastern Washington vineyards. American Journal of Enology and Viticulture, <a href="https://doi.org/10.5344/ajev.2021.20058">https://doi.org/10.5344/ajev.2021.20058</a>.</p><br /> <p>Molaei, B., A. Chandel, R.T. Peters, L.R. Khot, and J.Q. Vargas. 2021. Investigating lodging in Spearmint with overhead sprinklers compared to drag hoses using the texture feature from low altitude RGB imagery. Information Processing in Agriculture, <a href="https://doi.org/10.1016/j.inpa.2021.02.003">https://doi.org/10.1016/j.inpa.2021.02.003</a> </p><br /> <p>Ranjan , R.; R. Sinha , L.R. Khot, G.-A. Hoheisel, M. Grieshop, and M. Ledebuhr. 2021. Spatial distribution of spray from a solid set canopy delivery system in a high-density apple orchard retrofitted with modified emitters. Applied Sciences, 11, 709. <a href="https://doi.org/10.3390/app11020709">https://doi.org/10.3390/app11020709</a></p><br /> <p>Ranjan , R., L. R. Khot, R. Troy Peters, M. R. Salazar-Gutierrez and G. Shi . 2020. In-field crop physiology sensing aided real-time apple fruit surface temperature monitoring for sunburn prediction. Computers and Electronics in Agriculture, 157: 105558. <a href="https://doi.org/10.1016/j.compag.2020.105558">https://doi.org/10.1016/j.compag.2020.105558</a></p><br /> <p>Rathnayake, A. P., A. Chandel, M. Schrader, G.-A. Hoheisel and L. R. Khot. 2021. Spray patterns and perceptive canopy interaction assessment of commercial airblast sprayers used in Pacific Northwest perennial specialty crop production. Computers and Electronics in Agriculture, 184, 106097 <a href="https://doi.org/10.1016/j.compag.2021.106097">https://doi.org/10.1016/j.compag.2021.106097</a></p><br /> <p>Rathnayake, A. P., A. Chandel, M. Schrader, G.-A. Hoheisel and L. R. Khot. 2021. Air-assisted velocity profiles and perceptive canopy interactions of commercial airblast sprayers used in Pacific Northwest perennial specialty crop production. CIGR e-journal, Accepted, In Press.</p><br /> <p>Rathnayake, A. P., L. R. Khot, G. A. Hoheisel, H. W. Thistle, M. E. Teske, and M. J. Willett. 2021. Downwind spray drift assessment for airblast sprayer applications in a modern apple orchard system. Transactions of the ASABE, 64(2): 601-613. <a href="https://doi.org/10.13031/trans.14324">https://doi.org/10.13031/trans.14324</a> </p><br /> <p>Sinha, R., J. Quiros Vargas, L. R. Khot and S. Sankaran. 2021. High resolution aerial photogrammetry based 3D mapping of fruit crop canopies for precision inputs management. Information Processing in Agriculture, <a href="https://doi.org/10.1016/j.inpa.2021.01.006">https://doi.org/10.1016/j.inpa.2021.01.006</a>.</p><br /> <p>Worasit, S., A. Marzougui, S. Sankaran, L. R. Khot, A. A. Bates, and B. Schroeder. 2021. Identification of volatile biomarkers for high-throughput sensing of soft rot and Pythium leak diseases in stored potatoes. Food Chemistry, Accepted, In Press.</p><br /> <p>Zhang, X., He, L., Karkee, M., Whiting, M. D., and Zhang, Q. 2020. Field Evaluation of Targeted Shake-and-Catch Harvesting Technologies for Fresh Market Apple. Transactions of the ASABE, 63(6): 1759-1771. <a href="https://doi.org/10.13031/trans.13779">https://doi.org/10.13031/trans.13779</a> </p><br /> <p><strong>Thesis/Dissertations</strong></p><br /> <p>Chandel, Abhilash (2021). Small unmanned aerial system based remote sensing to map geospatial water use of field and perennial specialty crops. April 2021. Washington State University.</p><br /> <p>Ranjan, Rakesh (2021). Sensing integrated automated solid set canopy delivery system for crop loss management in deciduous fruits and grapevines. April 2021. Washington State University.</p><br /> <p><strong>Books and Book Chapters</strong></p><br /> <p>Huang, Y. and Q. Zhang, 2021. Agricultural Cybernetics. Springer, ISBN: 978-3-030-72102-2, (255 pp).</p><br /> <p>He, Y., P. Nie, Q. Zhang and F. Liu, 2021. Agricultural Internet of Things: Technologies and Applications. Springer, ISBN: 978-3-030-65701-7, (439 pp).</p><br /> <p>Karkee, M. and Q. Zhang, 2021. Fundamentals of Agricultural and Field Robotics. Springer, ISBN: 978-3-030-70399-8, (455 pp).</p><br /> <p>Karkee, M., Q. Zhang, and A. Silwal, 2021. Chapter 4. Agricultural Robots for Precision Agricultural Tasks in Tree Fruit Orchards. In: Bechar, A. (ed). Innovation in Agricultural Robotics for Precision Agriculture. Springer (26 pp).</p><br /> <p>Zhang, Q. and M. Karkee, 2021. Chapter 1. Agricultural Robotics: An Introduction. In: Karkee, M. & Q. Zhang (eds.). Fundamentals of Agricultural and Field Robotics. Springer (10 pp).</p><br /> <p>Karkee, M., B. Santosh, and Q. Zhang, 2021. Chapter 3. 3D Sensing Techniques and Systems. In: Karkee, M. & Q. Zhang (eds.). Fundamentals of Agricultural and Field Robotics. Springer (39 pp).</p><br /> <p>Zhang, X., Q. Zhang, M. Karkee, and M.D. Whiting, 2021. Chapter 16. Machinery-Canopy Interactions in Tree Fruit Crops. In: Karkee, M. & Q. Zhang (eds.). Fundamentals of Agricultural and Field Robotics. Springer (28 pp).</p><br /> <p>He, Y., Q. Zhang, and P. Nie, 2021. Chapter 1. Introduction of Agricultural IoT. In: He, Y., P. Nie, Q. Zhang, & F. Liu (eds.). Agricultural Internet of Things, Technologies and Applications. Springer (21 pp).</p><br /> <p>He, Y., Y. Tang, Q. Zhang, and Y. Zhao, 2021. Chapter 2. Agricultural IoT Standardization and System Applications. In: He, Y., P. Nie, Q. Zhang, & F. Liu (eds.). Agricultural Internet of Things, Technologies and Applications. Springer (17 pp).</p><br /> <p>Zhang, Q., Y. He, P. Nie, and S. Xiao, 2021. Chapter 3. Data Communication and Networking Technologies. In: He, Y., P. Nie, Q. Zhang, & F. Liu (eds.). Agricultural Internet of Things, Technologies and Applications. Springer (56 pp).</p><br /> <p>Liu, F., Y. He, Q. Zhang, W. Wang, and T. Shen, 2021. Chapter 5. Crop Information Sensing Technology. In: He, Y., P. Nie, Q. Zhang, & F. Liu (eds.). Agricultural Internet of Things, Technologies and Applications. Springer (32 pp).</p><br /> <p>Fang, H., Y. He, Q. Zhang, J. Zhang, and Y. Shi, 2021. Chapter 6. Field Condition Sensing Technology. In: He, Y., P. Nie, Q. Zhang, & F. Liu (eds.). Agricultural Internet of Things, Technologies and Applications. Springer (28 pp).</p><br /> <p>Nie, P., Q. Zhang, and Y. He, 2021. Chapter 10. IoT Management of Field Crops and Orchards. In: He, Y., P. Nie, Q. Zhang, & F. Liu (eds.). Agricultural Internet of Things, Technologies and Applications. Springer (12 pp).</p><br /> <p>Rovira-Más, F., Q. Zhang, and V. Saiz-Rubio, 2020. Chapter 11. Mechatronics and Intelligent Systems in Agricultural Machinery. In: Holden, N. M., Wolfe, M. L., Ogejo, J. A., & Cummins, E. J. (Ed.), Introduction to Biosystems Engineering. Virginia Tech Publishing.</p>Impact Statements
- Washington • Labor shortage and work-induced safety are two of major challenges in Washington State agriculture. Washington State University (WSU) team has focused on developing mechanization and automation solutions for mechanical harvest of apples for fresh market, and also worked closely with equipment manufacturers to support technology transfer from research to products. For example, WSU team has collaborated with FFRobotics Inc. (Israel) in developing and testing a full scale (12-armed) robotic machine for apple picking. In the test with planner apple trees in WA, it achieved a 100% accuracy in detecting fruit in canopies with a machine vision system and about 70% success rate in picking. Growers witnessed field tests have shown their willingness to adopt a robotic picking system like this one as it can perform the majority of picking job to allow growers maintain a small proportion of current workforce necessary for apple harvesting. The result is promising for commercial adoption of such a robotic picking system in near future, which will make a huge positive impact to apple industry to minimize the need and cost associated with farm labor and improve long term sustainability of the industry. In addition, the WSU team has also developed and tested an alternative approach of fresh market apple harvesting using a targeted shake-and-catch harvesting system. This system has much higher throughput than a robotic picking system and can achieve more than 90% fruit picking rate while keeping fruit damage to about 10% for some varieties such as Jazz and Fuji. The tree fruit industry has recognized the potential of this complementary solution for apple harvesting (there was good coverage of this technology in Good Fruit Growers Magazine about a year ago) and the design concept was award ‘2019 Rainbird Engineering Concept of the Year Award’ by American Society of Agricultural and Biological Engineers.
Date of Annual Report: 08/01/2022
Report Information
Period the Report Covers: 10/01/2021 - 09/30/2022
Participants
In-person:1. Zahid Aslan (Texas A&M Univ.)
2. Dana Choi (Univ. of Florida)
3. Joe Dvorak (Univ. of Kentucky)
4. Hao Gan (Univ. Tennessee)
5. Daniel Guyer (Michigan State Univ.)
6. Long He (Pennsylvania State. Univ.)
7. Xin Zhang (Mississippi State Univ.)
8. Qin Zhang (Washington State Univ.)
9. Irwin Donis Gonzalez (UC Davis)
10. Mason Earles (UC Davis)
11. Stavros Vougioukas (UC Davis)
Online:
1. Yu Jiang (Cornell Univ.);
2. Hao Gan (Univ. of Tennessee)
3. Joseph Dvorak (Univ. of Kentucky)
4. Long He (Pennsylvania State Univ.)
5. Mark Siemens (Univ. of Arizona)
6. Qin Zhang (Washington State Univ.)
7. Xin Zhang (Mississippi State Univ.)
8. Steven Thompson (USDA NIFA)
9. Ganesh Bora (USDA NIFA)
10. Won Suk “Daniel” Lee (Univ. of Florida)
11. Mark Siemens (Univ. of Arizona)
12. Ning Wang (Oklahoma State Univ.
13. Alex Thomasson (Mississippi State Univ.)
14. Daniel E Guyer (Michigan State Univ.)
Brief Summary of Minutes
Meeting Summary:
8:00 AM - Meeting called to order (Dana Choi)
8:00 AM – 8:15 AM - Attendee introduction
8:15 AM – 8:45 AM – Ganesh Bora – National Program Leader, NIFA-USDA
- History of legislation for USDA
- Review of different acts related to multistate
- USDA fiscal priorities
- Formation of multi-state projects
- Some pertinent competitive grant program
- New investigator and seed grants (USDA NIFA-AFRI)
- Priorities to be addressed (CAFF)
- Steven Thompson (USDA NIFA) introduced himself and his work in USDA.
8:45 AM – 10:00 AM – Business meeting
- Summary of 2021 meeting/reports approved with a minor edit by participants
- 2023 meeting location and time
Cornel, in June 2023, Yu Jiang (Cornell University) will host
- New secretary nomination/election
Hao Gun (University of Tennessee)
- Project renewal (proposal writing team organization)
Submit the proposal by Jan 15, 2023
Or termination by Sep
Suggestions for changing the title of the group or adding the number of objectives
Stavros Vougioukas (UC Davis) leads the proposal
Zahid Aslan (Texas A&M Univ.)
Joe Dvorak (Univ. of Kentucky)
Hao Gan (Univ. Tennessee)
Irwin Donis Gonzalez (UC Davis)
- Announcements
FIRA, Fresno, CA, Oct 18-20, 2022, 3 Day conference organizes by the international robotic association.
Agricontrol Sensing, Control and Automation Technologies for Agriculture - 6th AGRICONTROL
CIGR congress http://cigr2022.org/
ASABE robotic competition, request for judges, start from Sunday (July 17, 2022) and continue to Tuesday (July 19, 2022)
Cornel institute of digital agriculture (annual event and symposium) invite researchers to present at this event
S1090 Multistate project (2021-2026) AI in agroecosystems: big data and smart technology-driven sustainable production. 2022, Annual meeting Gainesville, FL, Aug 4
- Opportunities for collaboration
- Annual Report
Each institute sends one report by July 24 deadline. The one-page report should include accomplishment and impact (the most important part)
10:00 AM – 10:10 AM - Break
10:10 AM – 1:10 PM - Institute report presentations (Alphabetical order)
Order | Institute | Presenter |
1 | Texas A&M University | Zahid Aslan |
2 (Hybrid) | University of Florida | Dana Choi, Yiannis Ampatzidis; Won Suk “Daniel” Lee |
3 | University of California, Davis | Irwin Donis-Gonzalez; Stavros Vougioukas; Mason Earles |
4 | University of Kentucky | Joe Dvorak |
5 | University of Tennessee | Hao Gan |
Break – 10 minutes | ||
6 | Michigan State University | Daniel Guyer |
7 | Pennsylvania State University | Long He |
8 (Zoom) | University of Arizona | Mark Siemens; Pedro Andrade-Sanchez |
9 (Zoom) | Oklahoma State University | Ning Wang |
10 | Washington State University | Qin Zhang |
11 | Mississippi State University | Xin Zhang |
Accomplishments
<h2>Arizona</h2><br /> <ul><br /> <li>The novel, energy efficient band-steam applicator for controlling soilborne pests developed in the project was further evaluated with iceberg and romaine lettuce in field with a known history of Fusarium wilt of lettuce. Trial results showed that band-steam reduced fusarium wilt disease incidence by ~50%, increased head weight by > 20% and improved yield by > 300% and > 90% in iceberg and romaine lettuce respectively as compared to the untreated control. Additionally, use of band steam improved weed control by ~80% and reduced hand weeding labor requirements by > 50%. Machine work rate (0.05 ac/hr) and energy costs ($891/ac) were considered acceptable if labor reductions and yield increases can be realized.</li><br /> </ul><br /> <h2>California</h2><br /> <ul><br /> <li>An industry assessment study was conducted to quantify the performance of the current quality and food safety assessment system used in the Californian dehydrator onion and garlic industries. The study results show that the current system cannot reliably produce a statistically random sample due to several factors, including systematic bias created by 1) the design of the current mechanical sampling system and 2) the use of subjective decision-making processes by non-third-party individuals who may be perceived to have a conflict of interest in the assessment results. A design proposal was developed that would create a semi-automated sampling system capable of collecting a statistically random sample by eliminating sources of bias in the process as well as by eliminating the use of subjective human-controlled decisions. Ongoing research is being conducted to determine the economic feasibility of the proposed system as well as a timeline for potential implementation.</li><br /> <li>An automated high-throughput phenotyping (HTPP) system with a real-time, proximal plant architecture sensor with integrated GPS was created. The research was conducted that developed methods to measure architectural plant phenotypes (height, width, and volume) of different genotypes in the Solanaceae family utilizing 3D model data from a time-of-flight camera over the course of an entire growing season. The data collected and processed by the system using automated methods created a more comprehensive picture of the growth of a genotype over the course of a season with many more sample points, higher temporal resolution, and an entire 3D model as compared to traditional manual methods.</li><br /> <li>An inline moisture estimation system was built to measure the moisture content (MCwb) of green coffee using a time-domain reflectometry (TDR) probe. Model validation yielded a high correlation (R2 = 0.93) with accuracy of up to 91%. The TDR inline green coffee moisture estimation system has the potential to be applied in real-time, industrial-scale operations.</li><br /> <li>A hybrid (virtual and in-person) applied extension workshop was organized on the topic of emerging technologies addressing grand challenges in the produce industry. The workshop brought together academics and industry representatives to discuss challenges facing the produce distribution industry. Topics included: Automation in agriculture, mechanical and robotic harvesting, postharvest sensing, and controlling of quality.</li><br /> <li>While there have been discussions about how new technologies can increase output, optimize inputs, and save the environment, there hasn't been much attention to how safe these technologies are for farmers. A comprehensive study in collaboration with the National Institute of Safety and Health assesses the current situation of new technology safety in Agriculture. In addition, a workshop titled "Safety For Emerging Robotics and Autonomous aGriculture (SAFER AG) will be held November 9-10, 2022, in Urbana, Illinois.</li><br /> <li>A fruit-harvesting robot is being developed that comprises an array of custom-made linear robot arms. A ROS-based simulator was developed and tested for the robot, and a Mixed Integer Programming model was formulated to minimize the overall picking time, also known as the makespan. This new formulation computes the optimal harvester travel speed and the optimal overall arms-to-fruits schedule that maximizes the fruit picking throughput for a given minimum picking efficiency.</li><br /> <li>An automated Internet of Things (IoT) double ring infiltrometer (DRI) was developed and validated with the aim to facilitate soil infiltration mapping for precision agriculture and to build a soil infiltration inventory that could be used to continuously improve existing soil database.</li><br /> </ul><br /> <h2>Florida</h2><br /> <ul><br /> <li>A method for biomarker reflectance signature specification for disease detection and classification was developed</li><br /> <li>A technique was developed for sugarcane yield prediction and genotype selection using UAV-based hyperspectral imaging and machine learning</li><br /> <li>A method was developed for determining leaf nutrient concentrations in citrus trees using UAV imagery and machine learning</li><br /> <li>A yield prediction model was developed for citrus trees utilizing aerial and ground sensing and machine learning</li><br /> <li>A method was developed for the diagnosis of grape leaf diseases using automatic K-means clustering and machine learning</li><br /> <li>A smart tree crop sprayer was developed utilizing sensor fusion and artificial intelligence</li><br /> </ul><br /> <h2>Michigan</h2><br /> <ul><br /> <li>Three formal student senior design course projects focused on small- to medium-scale chestnut harvest automation were the primary efforts for Michigan under this multi-state project. The first project followed taking the common and functional hand-harvest tool of a “Nut Wizard” and designing an approach of coupling multiple of such together into a fully mechanical system that could be pushed or pulled by a small tractor or ATV to collect and deposit into an on-board containment bin. A prototype was built and tested. The second and third approaches were addressing the development of a pick-up concept and component for chestnuts on the ground (as is necessary due to maturation) if given a higher-technology small electric unmanned ground vehicle (UGV) platform system to move about the orchard having a vision system to locate the centroid of the chestnut within +/- 10 mm and move to the appropriate location. Thus, the task was focused on only the pick-up and on-boarding of the chestnut given vision and robotic vehicle technology already quite well developed. One of the concepts pursued the more traditional multi-jointed robotic type arm and a unique gripper development with the latter aspect being the most advancing and applicable. The other concept worked toward an automated, and less complex, approach of coming down onto the nut in a single dimension linear fashion/stroke and forcing the chestnut up into a tube and collecting the chestnuts until automatically dumping a set of multiple nuts after the tube fills. This last concept required engineering a static flexible end effector with similarity to a “shag bag” for collecting golf balls (however, chestnuts greatly vary in size and shape!). All concepts demonstrated approximately 80% harvest pick up efficiency depending on ground cover type/status.</li><br /> <li>Tangential indirect effort continues to advance, with technology and produce handling collaborators, to commercial-level prototyping, and hopefully beyond, of highly successful previous work under this project of non-destructive sorting of internal defects/quality using Computed Tomography (CT) and image processing. Examples include commodities with hard coatings such as chestnuts, and undesirable tissue characteristics such as fiber in whole carrots or asparagus.</li><br /> <li>Additionally, work was finished on one book chapter related to concepts behind mechanical harvesting of fruit trees and another book chapter is in final review on image-based automated insect identification toward the goal of automated scouting.</li><br /> <li>The current Michigan representative to this Multi-State Project is retiring and a new representative with likely a somewhat different specific focus will come on board in the coming year.</li><br /> </ul><br /> <h2>Mississippi</h2><br /> <ul><br /> <li>Improved fruit detectors: Various fruit detection models were improved architecturally for better detection accuracy and faster speed, including canopy-attention-YOLOv4 and Res-Dense-focal-DeepLabv3+ on apples or wine grapes in field conditions. Accurate machine vision system will be able to serve as a solid foundation for efficient and reliable robotic harvesting and canopy management.</li><br /> <li>Tested robotic end-effector: A machine vision enabled robotic end-effector was designed and tested indoor for automatic cotton harvesting. The end-effector was able to locate and reach to the cotton tissue automatically. It also can detach the cotton from the cotton ball completely without cutting off the cotton tissue for better cotton quality.</li><br /> </ul><br /> <h2>Oklahoma</h2><br /> <ul><br /> <li>A line-scan X-ray camera system was designed and developed to automate the inspection of peanut pods. In X-ray images, healthy peanut pods filled with seed are denser than infected pods filled with teliospores of <em>Thecaphora frezzii. </em>We developed a control system and a computer-based user interface to allow easy operations of the X-ray imaging system. A previously developed image processing and analysis algorithm was modified to fit for the developed line-scan camera.</li><br /> <li>The system will improve the efficiency of disease rating process during peanut breeding and new variety development for peanut smut resistance. Current hand-opening peanut pods method during the disease rating will be replaced with the automated, multipods detection system. This new technology is hoping to greatly improve the speed and accuracy of the disease rating process and allow automatic data storage and backup.</li><br /> </ul><br /> <h2>Oregon</h2><br /> <ul><br /> <li>The Economic Model to Compare Crop Rotations is an interactive tool written in Excel<sup>©</sup> that allows agricultural producers to compare the financial impacts of changing to an alternative crop rotation. The model will enable growers to develop two alternative rotations compared to their current cropping system based on whole-farm net returns, quantities of production inputs, and crops grown. Producers can select from 21 crops and two fallow options provided in a drop-down menu. These crops are typically grown in the dryland production systems in the inland Pacific Northwest. Also provided is one livestock budget for rotations that may include livestock grazing. This model is unique in that when a crop is added, replaced, or acres increased or decreased, the costs of producing other crops in that rotation also change. Not only are budgets dynamic, but each of the three rotations is independent of the other. As acres of crops are modified, the budget information only within that specified rotation changes. The foundation of this model is enterprise budgets, which include gross income, out-of-pocket cash costs, and fixed costs, broken into cash fixed and fixed non-cash costs. Producers can modify inputs, such as crop yields and prices, seeding rates, chemicals applied, fertilizer requirements, custom hire operations, the number of times a field operation occurs during a production season, machinery costs, the width of implements, speed of operations, and field efficiencies. The results show the total farm net returns for each rotation, the number of inputs used, and the value difference and percentage change from the grower's current rotation. The output also includes the total amounts of crop seeds, fertilizers, pesticides, machinery labor, fuel use, repairs and maintenance, and production units. This model allows producers to assess the financial and environmental tradeoffs in dryland farming. Producers can use this model to make cropping decisions based on 1) total net returns, 2) reducing fertilizer and chemical inputs, or 3) a balanced approach based on their financial and environmental goals. The Economic Model to Compare Crop Rotations was designed as an easy-to-use tool. However, instructional videos are included to assist producers with limited understanding of Excel or knowledge of financial data.</li><br /> </ul><br /> <h2>Pennsylvania</h2><br /> <ul><br /> <li>Two green fruit thinning end-effectors were developed and tested in the orchard environment. The test results showed that the end-effectors were able to singulate the targeted fruits with high success.</li><br /> <li>A deep learning based algorithm was developed to segment green fruits and fruit stems, then the orientation of the fruits were identified to provide guidance for the robotic green fruit system to remove fruits. A path planning algorithm was also developed with a six-degree-freedom robotic arm to engage targeted green fruits.</li><br /> <li>A series of early apple buds images were acquired with two image acquisition systems, and a YOLOv4 model was developed to detect the buds in the tree canopies.</li><br /> <li>A Cartesian robotic thinning system was developed to apply targeted blossom thinning, including a Cartesian robot, a ZED camera, and a solenoid valve controlled nozzle. Field tests indicated that the robotic thinning system saved more than 50% chemical and achieve relatively better thinning performance in the narrow tree canopy trees.</li><br /> <li>A computational fluid dynamic model (CFD) for heat transfer in porous canopies was developed and validated. The influences of heater output, heating duration, and heating angles were simulated, and validated in the field condition with two propane heaters. Developed a ground vehicle platform that can be operated autonomously using a RF-based transceiver and UDP protocol, built and tested a UAV that can capture and send spatiotemporal maps in real-time using a heuristic approach.</li><br /> <li>Continued the field tests with the developed precision irrigation and precision spraying system in the apple and peach orchards for various purposes.</li><br /> </ul><br /> <h2>Tennessee</h2><br /> <ul><br /> <li>A mobile robotic farm (Farmbot) was built in preparation of simulating drone-based autonomous pollination operation. A mobile raised bed was constructed as the supporting infrastructure for both a robotic gantry system, bedding materials and crops. Tomato plants have been grown in the bed since March 2021. The robotic farm has been controlled through custom-developed Python code. Images has been taken which will be used to develop computer vision algorithms for flower identification and localization.</li><br /> <li>A mini-IoT system including five independent units were developed for real-time environmental sensing. The system consists of a raspberry pi-based central data transmission unit, and four Arduino-based end devices. The end devices collect soil moisture, CO2, temperature and relative humidity data for display on a cloud-based dashboard. The system was developed with the goal of being ultra-low power. The end devices are powered by four AA batteries and can work continuously for roughly 4 - 6 months.</li><br /> </ul><br /> <h2>Texas</h2><br /> <ul><br /> <li>A deep learning model was developed to detect the bacterial wilt disease in greenhouse tomato crops. Over-amplification of the contrast due to uneven illumination is a major concern for color image-based disease identification systems. We used image enhancement algorithms to adjust the light and contrast in the images to improve the model performance. The developed model successfully detected the disease symptoms and severity levels on leaves and stems with superior accuracy, precision, and recall.</li><br /> </ul><br /> <h2>Washington</h2><br /> <ul><br /> <li>A vision-based flower cluster detection system has been developed and tested in apple and cherry orchards. This system reached an 86% accuracy on cluster location detection and an 84% accuracy on flower density estimation. This system could be used on both fruit tree blossom thinning robot and pollination robot underdevelopment at WSU.</li><br /> <li>Continue improve a machine-vision (using a tracking camera) and robotic system for pruning apple trees, which has been tested in the laboratory environment and has started the tuning and testing in commercial apple orchards.</li><br /> <li>Continue working with a commercial partner to convert and integrate the outcomes from our robotic apple harvesting research to a product prototype to make it usable to apple growers in Washington, PNW of US, and beyond.</li><br /> <li>Improved the design of the automated green shoot thinning machine and tested a research prototype in commercial vineyards. The project has moved into the third phase of collaborating with a local manufacturer in developing a product prototype. The first commercial prototype is expected to be fabricated for field test in 2023 growing season.</li><br /> <li>Designed an information management platform for a DEMO farm of smart agriculture. The infostructure of this demo farm will consist of a field network of sensors, a (or more) mobile equipment connected to the field network, an in-house control center, and a data communication link between field and farm office. The construction of this platform is under way.</li><br /> <li>Working on transmitting a developed technology of synthesized Cellulose NanoCrystals (CNC)-based dispersion for insulating tree fruit buds thus protecting them from cold damage. A licensing agreement has been reached between WSU and an interested commercial partner.</li><br /> <li>Improved and conducted field testing of an Internet-of-Things (IoT) enabled Crop Physiology Sensing System (CPSS 3.0) for apple fruit surface temperature monitoring and real-time actuation of modified evaporative cooling system for heat stress management in fresh market apple cultivars (cv Honeycrisp and Cosmic Crisp). CPSS 3.0 has been refined to extract other canopy/fruit quality attributes to aid grower decision support.</li><br /> <li>Developed aerial imagery (multispectral and thermal) based spectral sensing integrated energy balance modeling approach to estimate evapotranspiration (ET) at high spatial resolution (10 cm/pixel). Validate the approach for ET and leaf level transpiration (aka crop water use) estimation in grapevine, apple, and field crops (mint, alfalfa, potato).</li><br /> <li>Developed and validated tree-row-volume and unit-canopy-ration driven base spray rate estimation approaches for an intelligent orchard sprayer to perform efficient spray applications in tall spindle apple crop canopies.</li><br /> </ul><br /> <p> </p>Publications
<h2>Arizona</h2><br /> <p><strong>Book Chapter</strong></p><br /> <p>Fennimore, S.A. & Siemens, M.C. 2022. Mechanized weed management in vegetable crops. In <em>Encyclopedia of Smart Agricultural </em>Technologies, ed. Qin Zhang. (accepted)</p><br /> <p><strong>Outreach Publications</strong></p><br /> <p>Siemens, M.C. 2022. New Weeding Technologies for the 2022 Growing Season – Article II. 1 July 13. Vol. 14, Issue 14. Tucson, Ariz.: University of Arizona, Yuma Agricultural Center.</p><br /> <p>Siemens, M.C. 2022. New Weeding Technologies for the 2022 Growing Season. 1 June 29. Vol. 13, Issue 13. Tucson, Ariz.: University of Arizona, Yuma Agricultural Center.</p><br /> <p>Siemens, M.C. 2022. 2022 International Robotic Ag Technologies. 1 June. Vol. 13, Issue 11. Tucson, Ariz.: University of Arizona, Yuma Agricultural Center.</p><br /> <p>Siemens, M.C. 2022. 2022 Automated Technology Field Day – Salinas, CA : UC Cooperative Extension. 18 May. Vol. 13, Issue 10. Tucson, Ariz.: University of Arizona, Yuma Agricultural Center.</p><br /> <p>Siemens, M.C. 2022. Control of Palmer Amaranth with Finger Weeders Shows Good Promise in Texas A&M Cotton Studies. 4 May. Vol. 13, Issue 9. Tucson, Ariz.: University of Arizona, Yuma Agricultural Center.</p><br /> <p>Siemens, M.C. 2022. Specialty Crop Agricultural Robotics and Technology Forum. 20 April. Vol. 13, Issue 8. Tucson, Ariz.: University of Arizona, Yuma Agricultural Center.</p><br /> <p>Siemens, M.C. 2022. Finger Weeder Removing Large Palmer Amaranth Plant in Cotton Video. 6 April. Vol. 13, Issue 7. Tucson, Ariz.: University of Arizona, Yuma Agricultural Center.</p><br /> <p>Siemens, M.C. 2022. 2nd Generation Prototype Band-Steam Machine – Initial Testing. 22 March. Vol. 13, Issue 6. Tucson, Ariz.: University of Arizona, Yuma Agricultural Center.</p><br /> <p>Siemens, M.C. 2022. Pre-Plant Injection of Steam for Controlling Soilborne Pathogens and In-Row Weeds: Summary of Trial Results. 9 March. Vol. 13, Issue 5. Tucson, Ariz.: University of Arizona, Yuma Agricultural Center.</p><br /> <p>Siemens, M.C. 2022. “Innovations in Weed Control Technologies” Session and Field Demo at 2022 Southwest Ag Summit. 23 February. Vol. 13, Issue 4. Tucson, Ariz.: University of Arizona, Yuma Agricultural Center.</p><br /> <p>Siemens, M.C. 2022. Band-Steam Applicator Field Demo and Trial Results – 2022 Southwest Ag Summit. 9 February. Vol. 13, Issue 3. Tucson, Ariz.: University of Arizona, Yuma Agricultural Center.</p><br /> <p>Siemens, M.C. 2022. Use of Steam for Post Emergent Weed Control. 26 January. Vol. 13, Issue 2. Tucson, Ariz.: University of Arizona, Yuma Agricultural Center.</p><br /> <p>Siemens, M.C. 2022. Control of Fusarium Wilt with Band-Steam – Trials Show Mixed Results. 12 January. Vol. 13, Issue 1. Tucson, Ariz.: University of Arizona, Yuma Agricultural Center.</p><br /> <p>Siemens, M.C. 2021. Automated Thinning Machine Performance – Vigilance Important. 15 December. Vol. 12, Issue 25. Tucson, Ariz.: University of Arizona, Yuma Agricultural Center.</p><br /> <p>Siemens, M.C. 2021. Camera Guided Shift Hitch and Finger Weeders. 1 December. Vol. 12, Issue 24. Tucson, Ariz.: University of Arizona, Yuma Agricultural Center.</p><br /> <p>Siemens, M.C. 2021. Commercial Autonomous Ag Field Robots - Update. 17 November. Vol. 12, Issue 23. Tucson, Ariz.: University of Arizona, Yuma Agricultural Center.</p><br /> <p>Siemens, M.C. 2021. Automated In-Row Weeders Impress in Weedy Fields. 3 November. Vol. 12, Issue 22. Tucson, Ariz.: University of Arizona, Yuma Agricultural Center.</p><br /> <p>Siemens, M.C. 2021. 2nd Automated Weeding Technologies Field Demo – Update. 06 October. Vol. 12, Issue 20. Tucson, Ariz.: University of Arizona, Yuma Agricultural Center.</p><br /> <p><strong>Invited Presentations</strong></p><br /> <p>Siemens, M.C. 2022. “<em>New” Technologies and Innovations for Improved Weed Control.</em> New Mexico Chile Conference, February 1.</p><br /> <h2>California</h2><br /> <p>Perez-Ruiz, M., Slaughter, DC. 2021. Development of a precision 3-row synchronized transplanter. <em>Biosystems Engineering</em>. Vol 206: 67-78.</p><br /> <p>Anokye-Bempah, L, Phetpan, K, Slaughter, D.C. & Donis-González, I.R. 2022. Design, calibration, and validation of a green coffee inline moisture content estimation system using time-domain reflectometry (TDR). Submitted for publication; <em>Journal of Food Engineering</em>.</p><br /> <p>Peng, C., Vougioukas, S., Slaughter, D., Fei, Z., Arikapudi, R. (2022) A strawberry harvest-aiding system with crop-transport corobots: Design, development, and field evaluation<em>. Journal of Field Robotics</em>.</p><br /> <p>Chou, H-Y., Khorsandi, F., Vougioukas, S.G., Fathallah, F. (2022). Developing and Evaluating an Autonomous Agricultural All-Terrain Vehicle for Field Experimental Rollover Simulations. <em>Computers and Electronics in Agriculture</em> (194) 106735.</p><br /> <p>Avigal, Y., Wong, W., Presten, M., Theis, M., Aeron, S., Deza, A., Sharma, S., Parikh, R., Oehme, S., Carpin, S., Viers, J., Vougioukas, S., Goldberg, K. (2022). Simulating Polyculture Farming to Learn Automation Policies for Plant Diversity and Precision Irrigation. <em>IEEE Transactions on Automation Science and Engineering</em>. 19(3):1352-1364.</p><br /> <p>Fei, Z., Vougioukas, S.G. (2022). Row-sensing Templates: A Generic 3D Sensor-based Approach to Robot Localization with Respect to Orchard Row Centerlines. <em>Journal of Field Robotics</em>, 1-27.</p><br /> <ol><br /> <li>Abdelmoneim, A. Daccache, R. Khadra, M. Bhanot, G. Dragonetti (2021). Internet of Things (IoT) for double ring infiltrometer automation. <em>Computers and Electronics in Agriculture</em>, Volume 188, September 2021, 106324</li><br /> </ol><br /> <h2>Florida</h2><br /> <p>Patel, A., W. S. Lee, N. A. Peres, and C. W. Fraisse. 2021. Strawberry plant wetness detection using computer vision and deep learning. Smart Agricultural Technology 1, 2021, 100013, ISSN 2772-3755, <a href="https://doi.org/10.1016/j.atech.2021.100013">https://doi.org/10.1016/j.atech.2021.100013</a></p><br /> <p>Yun, C., H.-J. Kim, C.-W. Jeon, M. Gang, W. S. Lee, and J. G. Han. 2021. Stereovision-based ridge-furrow detection and tracking for auto-guided cultivator. Computers and Electronics in Agriculture 191, 2021, 106490, ISSN 0168-1699, <a href="https://doi.org/10.1016/j.compag.2021.106490">https://doi.org/10.1016/j.compag.2021.106490</a>.</p><br /> <p>Puranik, P., W. S. Lee, N. Peres, F. Wu, A. Abd-Elrahman, and S. Agehara. 2021. Strawberry flower and fruit detection using deep learning for developing yield prediction models. In the Proceedings of the 13th European Conference on Precision Agriculture (ECPA), July 19-22, 2021, Budapest, Hungary.</p><br /> <p>Zhou, X., W. S. Lee, Y. Ampatzidis, Y. Chen, N. Peres, and C. Fraisse. 2021. Strawberry maturity classification from UAV and near-ground imaging using deep learning. <em>Smart Agricultural Technology</em> 1, 2021, 100001, ISSN 2772-3755, https://doi.org/10.1016/j.atech.2021.100001.</p><br /> <h2>Michigan</h2><br /> <p>Guyer, D.E. Mechanical Harvesting. In: Advanced Automation for Tree Fruit Orchards and Vineyards. Eds: Vougioukas and Zhang. Springer Media. (at publisher)</p><br /> <p>Guyer, D.E. Advances in Image-based Identification and Analysis of Crop Insect Pests. In: Advances in Monitoring of Native and Invasive Insect Crop Pests. Eds: Fountain and Pope. Burleigh Dodds Publishing. (in final review)</p><br /> <h2>Mississippi</h2><br /> <p><strong>Publications</strong></p><br /> <p>He, L., Zhang, X., & Zahid, A. (2022). Chapter 3 – Mechanical management of modern planar fruit tree canopies. In Advanced Automation for Tree Fruit Orchards and Vineyards (Vougioukas, S. G., & Zhang, Q. ed.), <em>Springer Book Series: Agriculture Automation and Control</em>. (In Press)</p><br /> <p>Lu, S., Chen, W., Zhang, X., & Karkee, M. (2022). Canopy-attention-YOLOv4-based immature/mature apple fruit detection on dense-foliage tree architectures for early crop load estimation. <em>Computers and Electronics in Agriculture</em>, 193, 106696.</p><br /> <p>Upadhyaya, P., Karkee, M., Kashetri, S., & Zhang, X. (2022). Automated lag phase detection in wine grapes. In Proceedings of the 15<sup>th</sup> International Conference on Precision Agriculture (unpaginated, online). Monticello, IL: International Society of Precision Agriculture.</p><br /> <p>Peng, H., Zhong, J., Liu, H., Li, J., Yao, M., & Zhang, X. (2022). ResDense-focal-DeepLabv3+ enabled litchi branch semantic segmentation for robotic harvesting. Available at SSRN.</p><br /> <p>Barnes E. M., G. Morgan, K. Hake, J. Devine, R. Kurtz, G. Ibendahl, A. Sharda, G. Rains, J. Snider, J. M. Maja, J. A. Thomasson, Y. Lu, H. Gharakhani, J. Griffin, E. Kimura, R. Hardin, T. Raper, S. Young, K. Fue, M. Pelletier, J. Wanjura, and G. Holt. 2021. Opportunities for robotic systems and automation in cotton production. <em>AgriEngineering </em>3(2):339-362; doi.org/10.3390/agriengineering3020023.</p><br /> <p>Gharakhani, H., J. A. Thomasson, and Y. Lu. 2022. An end-effector for robotic cotton harvesting. <em>Smart Agricultural Technology</em> 2(1):1-11.</p><br /> <p><strong>Presentations</strong></p><br /> <p>Zhang, X. & Regmi, A. MLCAS2021 Crop yield prediction challenge. 3<sup>rd</sup> International Workshop on Machine Learning for Cyber-Agricultural Systems, online (11/2/2021–11/3/2021) (invited talk)</p><br /> <p>Zhang, X. Study of canopy-machine interaction in mass mechanical harvest of fresh market apples. 30<sup>th</sup> Members’ Meeting of the Club of Bologna (Agriculture mechanization vision for the future: The Club of Bologna thirty years of contribution for improve its diffusion and sustainability), Bologna, Italy (10/22/2021–10/23/2021) (invited talk)</p><br /> <p>Zhang, X., Liu, X., Lu, S., & Karkee, M. Deepsort-YOLOv3: Robust on-the-go grape bunch video tracking for yield estimation throughout the growth season. ASABE AIM, Houston, TX (7/17/2022–7/20/2022)</p><br /> <p>Chakraborty, M., Pourreza, A., Zhang, X., Jafarbiglu, H., & Shackel, K. A. Almond bloom mapping at the tree level for early yield forecasting. ASABE AIM, Houston, TX (7/17/2022–7/20/2022)</p><br /> <p>Upadhyaya, P., Zhang, X., Lu, S., & Karkee, M. Smartphone-app for crop load estimation and lag phase detection in wine grapes. 15<sup>th</sup> International Conference on Precision Agriculture (ICPA), Minneapolis, MN (6/26/2022–6/29/2022)</p><br /> <h2>Pennsylvania</h2><br /> <p><strong>Journal Publications:</strong></p><br /> <p>Zhang, H., He, L., Di Gioia, F., Choi, D., Elia, A. and Heinemann, P., 2022. LoRaWAN based internet of things (IoT) system for precision irrigation in plasticulture fresh-market tomato<em>. Smart Agricultural Technology</em>, 2, p.100053.</p><br /> <p>Yuan, W., Choi, D., Bolkas, D., Heinemann, P.H. and He, L., 2022. Sensitivity examination of YOLOv4 regarding test image distortion and training dataset attribute for apple flower bud classification. <em>International Journal of Remote Sensing</em>, 43(8), pp.3106-3130.</p><br /> <p>Zahid, A., Mahmud, M.S., He, L., Schupp, J., Choi, D. and Heinemann, P., 2022. An Apple Tree Branch Pruning Analysis. <em>HortTechnology</em>, 32(2), pp.90-98.</p><br /> <p>Hussain, M., He, L., Schupp, J. and Heinemann, P., 2022. Green Fruit Removal Dynamics for Robotic Green Fruit Thinning End-Effector Development<em>. Journal of the ASABE</em> (in press).</p><br /> <p>Xiao, D., Pan, Y., Feng, J., Yin, J., Liu, Y. and He, L., 2022. Remote sensing detection algorithm for apple fire blight based on UAV multispectral image. <em>Computers and Electronics in Agriculture</em>, 199, p.107137.</p><br /> <p>Mahmud, M.S., Zahid, A., He, L., Choi, D., Krawczyk, G. and Zhu, H., 2021. LiDAR-sensed tree canopy correction in uneven terrain conditions using a sensor fusion approach for precision sprayers. <em>Computers and Electronics in Agriculture</em>, 191, p.106565.</p><br /> <p><strong>Book Chapter:</strong></p><br /> <p>He, L., Zahid, A. and Mahmud, M.S., Robotic Tree Fruit Harvesting: Status, Challenges, and Prosperities. <em>Sensing, Data Managing, and Control Technologies for Agricultural Systems</em>, p.299-332.</p><br /> <p><strong>Extension Publication:</strong></p><br /> <p>He, L., Choi, D., & Pecchia, J. (2021). "Investigation of computer vision system and robotic picking mechanism for button mushroom harvesting." Mushroom News.</p><br /> <p>He, L. (2021). Introduction of automatic irrigation systems for tree fruit orchards. Penn State Extension.</p><br /> <p>He, L., Shannon, T., & Mahmud, M. S. (2021). Unmanned aerial vehicle-based crop scouting in fruit trees. Penn State Extension.</p><br /> <p><strong>Thesis/Dissertations: </strong></p><br /> <p>Md Sultan Mahmud (2022). Study of core technologies in tree canopy parameter measurements for development an advanced precision sprayer. PhD Dissertation. June 2022. Pennsylvania State University.</p><br /> <p>Wenan Yuan (2022). Development of a UAV-based multi-dimensional mapping framework for precise and convenient frost management in apple orchard. PhD Dissertation. May 2022. Pennsylvania State University.</p><br /> <h2>Tennessee</h2><br /> <p>Oleksak, K., Wu, Y., Abella, M., Wang, Z., & Gan, H. (2021). Trajectory Optimization of Unmanned Aerial Vehicles for Wireless Communication with Ground Terminals. In AIAA Scitech 2021 Forum (p. 0709).</p><br /> <p>Daniel, A., Wu, Y., Wang, Z., & Gan, H. (2021). Trajectory Optimization of Unmanned Aerial Vehicles for Wireless Coverage under Time Constraint. In AIAA Scitech 2021 Forum (p. 1581).</p><br /> <p>Rice, C., McDonald, S., Gan, H., Lee, W.S., Chen, Y., Shi, Y., Wang, Z. (2022) Perception, path planning, and flight control for drone-enabled autonomous pollination system. <em>Computers and Electronics in Agriculture</em> (under review)</p><br /> <h2>Texas</h2><br /> <p><strong>Publications:</strong></p><br /> <p>Ahamed M. S., Sultan, M., Monfet, D., Rahman, M.S., Zhang, Y., Zahid, A., Aleem, M., Achour, Y., and Ahsan, T. M. A. 2022. Thermal environment controls and sustainability challenges in indoor vertical farming, <em>Journal of Cleaner Production [Under review]</em></p><br /> <p>Ojo, M., and Zahid, A. 2022. Deep learning and its potential in controlled environment agriculture, <em>Applied Intelligence</em> <em>[Under review]</em></p><br /> <p><strong>Presentations:</strong></p><br /> <p>Ojo, M., Zahid, A. 2022. Automatic crop disease scouting system based on deep neural networks model. In 2022 ASABE Annual International Virtual Meeting Houston TX (Presentation)</p><br /> <p>Zahid, A. 2021. Robotics and intelligent systems for controlled environment agriculture, In 3<sup>rd</sup> Annual Controlled Environment Agriculture Conference, Dallas TX (Presentation)</p><br /> <h2>Washington</h2><br /> <p><strong>Journal Articles</strong></p><br /> <p>Bhattarai, U., & Karkee M. (2022). A Weakly Supervised Approach for Flower/Fruit Counting in Apple Orchards. Computers in Industry, 138, 103635. <a href="https://doi.org/10.1016/j.compind.2022.103635">https://doi.org/10.1016/j.compind.2022.103635</a></p><br /> <p>Bhusal, S., U. Bhattarai, M. Karkee, Y. Majeed, & Q. Zhang. (2022). Automated execution of pest bird deterrence system using a programmable unmanned aerial vehicle (UAV). <em>Computers and Electronics in Agriculture</em>. <a href="https://doi.org/10.1016/j.compag.2022.106972">https://doi.org/10.1016/j.compag.2022.106972</a>.</p><br /> <p>Gao, Z., Y. Zhao, G.-A. Hoheisel, L.R. Khot, and Q. Zhang, 2021. Blueberry bud freeze damage detection using optical sensors: Identification of spectral features through hyperspectral imagery. <em>Journal of Berry Research</em>, <a href="https://doi.org/10.3233/JBR-211506">https://doi.org/10.3233/JBR-211506</a>.</p><br /> <p>Guo, J., Karkee, M., Yang, Z., Fu, H., Li, J., Jiang, Y., ... & Duan, J. (2021). Discrete element modeling and physical experiment research on the biomechanical properties of banana bunch stalk for postharvest machine development. Computers and Electronics in Agriculture, 188, 106308.</p><br /> <p>Guo, J., Karkee, M., Yang, Z., Fu, H., Li, J., Jiang, Y., ... & Duan, J. (2021). Research of simulation analysis and experimental optimization of banana de-handing device with self-adaptive profiling function. Computers and Electronics in Agriculture, 185, 106148.</p><br /> <p>Lohan, S. K., Narang, M. K., Singh, M., Singh, D., Sidhu, H. S., Singh, S., Dixit, A.K, & Karkee, M. (2021). Design and development of remote-control system for two-wheel paddy transplanter. Journal of Field Robotics. <a href="https://doi.org/10.1002/rob.22045">https://doi.org/10.1002/rob.22045</a></p><br /> <p>Lohan, S. K., Narang, M. K., Singh, M., Khadatkar, A., & Karkee, M. (2021). Actuating force required for operating various controls of walk-behind type paddy transplanter leading to development of remotely operated system. Journal of Agricultural Safety and Health, 27(2):87-103. DOI:<a href="http://dx.doi.org/10.13031/jash.14186">10.13031/jash.14186</a></p><br /> <p>Lu, S., Chen, W., Zhang, X., & Karkee, M. (2022). Canopy-attention-YOLOv4-based immature/mature apple fruit detection on dense-foliage tree architectures for early crop load estimation. Computers and Electronics in Agriculture, 193, 106696</p><br /> <p>Rathnayake, A.P., A. Chandel, M. Schrader, G.-A. Hoheisel and L.R. Khot. 2022. Air-assisted velocity profiles and perceptive canopy interactions of commercial airblast sprayers used in Pacific Northwest perennial specialty crop production. <em>CIGR e-journal, </em>24(1): 73-89<em>. </em></p><br /> <p>Worasit, S., A. Marzougui, S. Sankaran, L. R. Khot, A. A. Bates, and B. Schroeder. 2021. Identification of volatile biomarkers for high-throughput sensing of soft rot and Pythium leak diseases in stored potatoes. <em>Food Chemistry, </em><a href="https://doi.org/10.1016/j.foodchem.2021.130910">https://doi.org/10.1016/j.foodchem.2021.130910</a><em>. </em></p><br /> <p>Zhou, Z., G. Diverres, C. Kang, S. Thapa, M. Karkee, Q. Zhang, & M. Keller. 2022. Ground-Based Thermal Imaging for Assessing Crop Water Status in Grapevines over a Growing Season. <em>Agronomy</em>, 12(2), 322.</p><br /> <p><strong>US Patents</strong></p><br /> <p>Zhang, X., C. Mo, M.D. Whiting, and Q. Zhang, 2021. Plant-based compositions for the protection of plants from cold damage. Publication No. US 2021/0029896 A1 (Feb 4, 2021).</p>Impact Statements
- WASHINGTON - Created an innovative method for crop cold damage prevention. WSU team has developed a novel synthesizes solution of Cellulose NanoCrystals (CNC) based dispersion which could be applied as a spray agent on tree fruit buds to prevent the frost damage. Test results obtained from laboratory indicated that CNC covered cheery buds could lower ice nucleation temperature to below -5 °C. Such results have been validated from large acreage of field tests for preventing cherry buds been damaged in a few frost events with lowest temperature close to that level in multi-year field trials. A licensing negotiation between WSU and an interested commercial partner has completed and an agreement is expected to be signed soon.
Date of Annual Report: 07/31/2023
Report Information
Period the Report Covers: 10/01/2022 - 09/30/2023
Participants
In-personYu Jiang (Cornell University)
Katie Gold (Cornell University)
Chang Chen (Cornell University)
Yuzhen Lu (Michigan State University)
Ning Wang (Oklahoma State University)
Paul Heinemann (Pennsylvania State University)
Long He (Pennsylvania State University)
Pennsylvania State University (Pennsylvania State University)
Zahid Aslan (Texas A&M University)
Mark Siemens (University of Arizona)
Qin Zhang (Washington State University)
Virtual
Alex Thomasson (Mississippi State University)
Vivian Voung (UC Davis)
Stavros Vougioukas (UC Davis)
Pedro Andrade Sanchez (University of Arizona)
Won Suk “Daniel” Lee (University of Florida)
Changying Li (University of Florida)
Davie M. Kadyampakeni (University of Florida)
Steven Thompson (USDA-NIFA)
Manjo Karkee (Washington State University)
Brief Summary of Minutes
Brief Summary of Minutes of Annual Meeting
Meeting Summary:
2023-06-20
8:30 AM – Meeting called to order (Yu Jiang)
8:30 AM to 8:45 AM – Attendee introduction
8:45 AM to 9:15 AM – Welcome address by Dr. Olga I. Padilla-Zakour, interim director of Cornell AgriTech
9:15 to noon – Business meeting
- Summary of 2022 meeting/reports approved.
- New secretary nominated and approved: Yuzhen Lu
- W4009 renewal update
- Proposal development led by Dr. Stavros Vougioukas and submitted the initial proposal on 2023-01-23. Proposal duration is from 2023-10-01 to 2028-09-30.
- Positive comments received on 2023-04-17, and major suggestions were to improve participation across the US.
- Revised proposal submitted on 2023-05-24, with 27 participants from 13 states.
- Waiting for the final approval from USDA NIFA.
- Meeting location and time in 2024
- Potential locations: Michigan, Florida, Texas, Hawaii (preferred due to its tropical fruit industry), and Alaska.
- The coming W4009 chair (Hao Gan) will work with a team to finalize the schedule.
- Announcements
- NIFA report by Dr. Steven Thomson
- Expand AI program, small- to medium-scale with focus on connection to MSI
- New USDA/NSF FRR program to replace the previous NRI
- NIFA/NSF CPS program
- AFRI A1521/A1541 have been very competitive.
- Special Issue from Computer and Electronics in Agriculture (IF 8.3)
- A special topic on specialty crop engineering. Application deadline: 2023-12-31, minimum 15 papers per special issue.
- Add acknowledgement to the W3009 and other programs supporting specialty crop engineering.
- FIRA conference: https://fira-usa.com/
- Cornell CIDA Hackathon: https://digitalaghackathon.com/
- NIFA report by Dr. Steven Thomson
- Collaboration opportunity
- A conference on specialty crop engineering
12:00 PM to 1 PM – Lunch
1:00 PM to 5:00 PM – Institute report presentations
5:05 PM – Adjourned the meeting.
Accomplishments
<p><span style="text-decoration: underline;">Arizona</span></p><br /> <ul><br /> <li>A new, energy-saving steam applicator effectively kills weed seeds before planting. Trials in spinach yielded excellent broadleaf weed control (>80%) and drastically cut manual weeding time (>80%), performing better than untreated controls. The tool's work rates and fuel costs were reasonable, even at a slow 0.15 mph speed, achieving 0.10 ac/hr and $800/ac.</li><br /> <li>A novel, low-cost electronic monitoring system of high temporal resolution in-situ soil respiration was developed, and an invention disclosure was filed on the device.</li><br /> </ul><br /> <p><span style="text-decoration: underline;">California</span></p><br /> <ul><br /> <li>A prototype was built to measure the yield of individual almond trees during mechanical harvesting with off-ground harvesters. The system does not require the harvester to stop; instead, it measures the volume of the almonds inline, as they are transported on the conveyor belts of a commercial harvested (TOL company). Thus, the system is compatible with commercial harvesting machines and procedures that implement high-speed harvesting at commercial scale.</li><br /> <li>Developed a fully automated almond yield mapping prototype system. The system comprised a 2D Lidar and an encoder to measure the almond pile profile and the speed of the belt respectively; an RTK-GPS to record harvester location; a vibration sensor to record the tree shaking time instants; an inertial sensor to record harvester motion. Software was developed to pre-process the data and combine the filtered and cleaned data from all the sensors to generate yield maps of the harvested almonds.</li><br /> <li>Initiated the research and outreach program related to safety for robotics and emerging technologies in agriculture.</li><br /> <li>Presented and co-organized the “Labor & Ergonomics for Emerging Technologies” session in the “Addressing Grand Challenges in the Produce Industry” workshop.</li><br /> <li>Hosted a hybrid (virtual and in-person) applied extension workshop titled: “Addressing Grand Challenges in the Produce Industry”.</li><br /> <li>Co-PI and Co-organizer of a workshop funded by USDA, AFRI ($50,000), titled “SAfety For Emerging Robotics and Autonomous aGriculture (SAFER AG)” that was held November 9-10, 2022, in Urbana, IL.</li><br /> <li>Co-host a workshop titled “Emerging Technology in Agriculture: Keeping Health and Safety at the Forefront” on May 11, 2023, at UC Davis in California, to discuss the safety and health concerns for farmworkers related to robotics and new technologies in agriculture.</li><br /> <li>Support ongoing development of a unique sensor system for in-field 3D plant phenotype measurements by introducing computer algorithms for automated, high-throughput phenotyping of Solanaceae crops like tomato and pepper. These machine learning and image processing algorithms successfully measure critical traits including the time to first open flower and first green fruit, requiring automatic detection in farm settings.</li><br /> <li>Practical learning modules were created to train engineering graduate students in designing autonomous robots and sensors using machine learning to automate laborious tasks in specialty crop production. The modules cover edge computing, IoT, network dashboards, servo motor control for navigation, optical and acoustic sensors for object detection, machine vision for crop detection and fruit localization, path optimization, automated harvest, transport, and machine learning.</li><br /> <li>A remote sensing model prototype was created to estimate grapevine leaf and canopy nitrogen levels using hyperspectral data, utilizing diverse data analytics methods like linear regression, machine learning, and physics-based modeling. A new hybrid method was developed, combining mechanistic trait retrieval outputs with advanced machine learning to improve model prediction accuracy and consistency.</li><br /> <li>Machine learning techniques were used to i) map global irrigated area at high temporal and spatial resolution using satellite imagery, ii) evaluate irrigation water demand at county level and iii) to evaluate feature importance and to forecast reference evapotranspiration.</li><br /> <li>Finalize editorial revisions for booklets, and extension type publication in topics related to fresh fruit and vegetable quality factors, their evaluation, and sensing of environmental conditions during the transport of refrigerated produce (Telematics).</li><br /> <li>Initiated the development of non-invasive visual assessment tools, including cell-phone applications, to infer quality attributes of dry coffee beans. This is beneficial to both the consumer and producers of coffee in California as a specialty crop.</li><br /> </ul><br /> <p><span style="text-decoration: underline;">Florida</span></p><br /> <ul><br /> <li>Kadyampakeni: We also had two presentations for in-service training for extension agents and crop advisors. Two Ph.D. students completed their doctoral programs.</li><br /> <li>Ampatzidis: Several disease detection and monitoring systems were developed for vegetable crops utilizing UAV-based hyperspectral imaging and machine learning. These technologies were able to classify several severity stages of the diseases.</li><br /> <li>Ampatzidis: An AI-enabled yield prediction system was developed for citrus using aerial and ground sensing systems.</li><br /> <li>Ampatzidis: An AI-enhanced technique was developed to determine citrus leaf nutrient concentration utilizing UAV multispectral imaging.</li><br /> <li>Ampatzidis: A smart tree crop sprayer was developed using sensor fusion and artificial intelligence.</li><br /> <li>Choi: This year, we successfully developed a computer vision-based and comprehensive strawberry crop load prediction system for yield forecasting. This system incorporated machine learning algorithms, sensor technology, and data analysis to predict harvest amounts throughout the growing season.</li><br /> <li>Choi: We have made significant progress in a project aimed at modernizing the manual mite release methods in strawberry farming. We have successfully built the first prototype of this mechanism, enhancing the precision of mite deployment in pest control. We have identified areas that require improvement to increase the overall system efficiency.</li><br /> <li>Lee: A smartphone app and a portable imaging device were developed to detect two-spotted spider mites using artificial intelligence. An autonomous vehicle navigation method was developed to improve the accuracy and robustness of existing fusion algorithms by taking information from a camera, IMU, and GPS receiver and fusing them through nonlinear batch optimization.</li><br /> </ul><br /> <p><span style="text-decoration: underline;">Kentucky</span></p><br /> <ul><br /> <li>We used data collected from Aquaculture projects to determine the appropriate sizing for solar systems to ensure continuous, remote power for various daily system electrical loads. Various configuration options were also considered.</li><br /> </ul><br /> <p><span style="text-decoration: underline;">Michigan</span></p><br /> <ul><br /> <li>Multi-class weed detection datasets were created and made publicly available to the research community to accelerate developing robust machine vision-based weed recognition systems. Accompanied with the open datasets were large suites of weed detection benchmarking models that are publicly accessible.</li><br /> <li>Open-source graphical software was developed for weed imagery and real-time weed detection.</li><br /> <li>Blueberry fruit detection models were built to assist in harvest decision making.</li><br /> </ul><br /> <p><span style="text-decoration: underline;">Mississippi</span></p><br /> <ul><br /> <li>The team evaluated a newly designed real-time moisture sensor system for cotton gin. Results showed that the system was sufficiently robust for real-time application with sufficient repeatability. Additional experiments are planned for the 2023 season to determine alternate configurations and to improve sensitivity.</li><br /> <li>The team developed a computer vision system to detect blackberries accurately and efficiently in the orchards, which will be utilized for soft robotic harvesting tasks in real-time. A dual-camera system is under development for better detection and localization purposes due to the small size of the target fruit. The graduate student working on this project won the Best Student Paper Award at SPIE conference in Orlando, FL.</li><br /> <li>The team developed an automated image processing pipeline to map the bloom of almond trees to early forecast the yield of almond trees using UAV-based approach. The proposed approach was compared against the previously developed method by other researchers, enhanced bloom index (EBI), and satellite-based method. Results showed our approach is promising to estimate the almond yield during bloom stage.</li><br /> <li>The team developed a computer vision system to detect wine grape bunches accurately, which will be utilized for crop load estimation for the growers in the vineyards. The proposed model was the combination of YOLOv5 and Swin-transformer to perform more efficiently.</li><br /> </ul><br /> <p><span style="text-decoration: underline;">New York</span></p><br /> <ul><br /> <li>Developed and deployed PhytoPatholoBot (PPB) in multiple grape production regions and breeding programs in the US to demonstrate autonomous disease scouting for genetics studies, breeding programs, and precision disease management.</li><br /> <li>Developed and deployed an attachable implement of UV treatment to trellised crops such as grapes, apples, and strawberries, which has attracted attention worldwide.</li><br /> <li>The team has utilized outcomes from the W3009 and collaborated with other state researchers to successfully secure additional funding support (NASA Acres and USDA SCRI VitisGen 3) for specialty crop engineering.</li><br /> </ul><br /> <p><span style="text-decoration: underline;">Pennsylvania</span></p><br /> <ul><br /> <li>A robotic apple blossom thinning system was develop with integration of an unmanned ground vehicle, a 3D machine vision system, a solenoid valve controlled- nozzle control system, and a RTK-GPS based localization system. A series of field test was conducted in the spring of 2023, and the results showed that the robotic blossom thinning system achieved targeted blossom thinning with more than 50% of chemical reduction and similar thinning performance by comparing to conventional air-blast sprayer.</li><br /> <li>An integrated robotic green fruit thinning system was developed and tested in apple orchards. The system includes a 3D machine vision system (detection and localization), a UR5 robotic manipulator, a stem cutting end-effector, and a collision-free path planning algorithm. Field test showed that the robotic system successfully removed 87.5% of targeted fruits in the clusters.</li><br /> <li>Continued to develop deep learning-based models for early apple bud detection and localization, and achieved over 90% accuracy for bud detection in orchard environment. Two types of end-effectors were developed and tested in the field for excessive bud removal. The test showed that the two end-effectors can remove flower buds at an early stage, while the scissor-type performed better in terms of efficiency.</li><br /> <li>A computational fluid dynamic model was developed to determine the effects of wind speed and wind direction on static and mobile heating of the orchard. Utilizing the concept of “percentage of protected canopy (PPC)”, the simulation model was used to assess which heater scenarios would provide the maximum PPC. A mobile heater was mounted on the UGV, and field tests showed the advantage of using wind adapted heater angle control can improve heating efficiency significantly.</li><br /> <li>Fully integrated, autonomous multi-agent frost protection system communications were in development. This provides the real-time capture of thermal images, transfer to a base station, execution of the path planning algorithm, and signal to the UGV to move to the destination for heating using the optimal path, without collisions and within acceptable navigation error. The calculated deviation of the UGV from the proposed path was 3.44 cm RMS error latitudinally and 8.28 cm longitudinally. The mean computational time for creating the paths was 7.85 seconds.</li><br /> <li>Continued the field tests with the developed precision irrigation and precision spraying systems in the apple orchards and vegetable fields for various purposes. Especially, both precision irrigation and spraying technologies have been attracting attention from tree fruit and vegetable growers. We have worked with more than 10 growers for irrigation sensor setup, and there are about 5 growers in the area have been or are about to adopt the precision spraying system.</li><br /> </ul><br /> <p><span style="text-decoration: underline;">Tennessee</span></p><br /> <ul><br /> <li>A mobile robot platform was built for Tennessee pot-in-pot nursery production. The chassis and motor control system was completed for 15-gallon pots. Currently, the robot can be remote controlled using a joystick. It includes two driving motors, two mechanical grippers to grab the 15-gallon pot, and two linear actuator to lift the pot.</li><br /> <li>A robotic farm built in previous years has been used to grow tomato plants and for developing flower mapping algorithms. Top-view image data was collected automatically by the robot. Yolo models were applied to detect flowers and Pix4D was used to create a 3D map of the entire plant bed. This is a preliminary study with an end goal of creating a drone-based autonomous pollination system.</li><br /> </ul><br /> <p><span style="text-decoration: underline;">Texas</span></p><br /> <ul><br /> <li>A deep learning model was developed to detect bacterial wilt disease in greenhouse tomato crops. Over-amplification of the contrast due to uneven illumination is a major concern for color image-based disease detection systems. We used image enhancement algorithms to adjust the light and contrast in the images. A new deep-learning approach was used to train the features on the plant leaves in the images to develop the disease prediction algorithm. The system achieved more than 90% classification accuracy, advocating its implementation for site-specific crop management systems, including robotic spraying and disease watchdog.</li><br /> <li>An end-to-end deep learning algorithm is developed for non-destructive measurements of hydroponic lettuce phenotypic traits. Different deep-learning approaches were tested to develop a lightweight model for deployment on edge devices. The results indicate that biomass, leaf area, dry weight, and plant height can be measured non-destructively with high accuracy (up to 95%). The developed algorithm can be deployed on edge devices for real-time crop growth monitoring.</li><br /> <li>A review of AI and automation applications in CEA and the nursery industry was conducted, and the results were published in a scientific journal. The survey highlighted the status of technology development for CEA and the nursery industry. Also, it summarized the pros and cons of different AI models and automation techniques implemented for various tasks in CEA production.</li><br /> </ul><br /> <p><span style="text-decoration: underline;">Washington</span></p><br /> <ul><br /> <li>Integrated an automated green shoot thinning system with a commercial thinning machine for precision green shoot thinning in vineyards.</li><br /> <li>Further improved a 12-armed robotic apple harvester and continued field evaluation of the harvester in commercial orchards.</li><br /> <li>Further developed a machine-vision and robotic system for pruning apple and cherry trees. Vision system was advanced to accurately detect secondary branches in trees and recreate 3D structure of trees for effective pruning decisions.</li><br /> <li>Integrated end-effector and vision system with a soft-robotic manipulator for apple picking and flower thinning.</li><br /> <li>Evaluated cell-phone-based models for lag-phase detection and crop-estimation in vineyards.</li><br /> <li>Developed a canopy density estimation method, a decision support system, and a precision application system for individual plant level nitrogen application in apple orchards.</li><br /> <li>Developed and evaluated various methods for improved water, nitrogen, and phosphorus status assessment in wine grapes using ground-based hyperspectral imaging.</li><br /> <li>Developed and evaluated a robotic system for pollination and green fruit thinning. Pollination. Robotic pollination system focused on identifying king flower and applying pollens precisely onto king flowers for targeted pollination. Robotic green fruit thinning has been investigated with various end-effectors including scissor cutter, claw-like mechanism, and vacuum suctioning.</li><br /> <li>Optimized, automated fixed spray system (i.e., solid set chemical delivery system) configurations were tested to validate the biological efficacy in apple orchard orchards. System configurations were revised for their applicability in crop protection and heat stress mitigation in grapevines.</li><br /> <li>Optimized intelligent sprayer spray rates to efficiently apply chemicals in modern apple orchards. The optimized systems are being tested for precision chemical blossom thinning in smart orchard blocks in the 2023 field season.</li><br /> <li>The IoT-enabled Crop Physiology Sensing System (CPSS 3.0) was automated to mitigate heat stress in apple orchards, evaluating the effectiveness of different cooling and netting techniques. It also adapted for heat stress mitigation in VSP trained grapevines.</li><br /> <li>Continual public-private partnership to establish smart orchard framework in commercial settings and develop data driven decision support for on-farm production management. WSU researched crop water use mapping solution has been translated to use for understanding crop water use (evapotranspiration) in smart apple orchard. The neutrality of the model was tested using manned aircraft collected arial imagery data.</li><br /> <li>Developed and validated grapevine canopy vigor variation mapping solutions using aerial imagery data for in season decision support on canopy, irrigation management, precision crop protection, etc.</li><br /> </ul>Publications
<p><span style="text-decoration: underline;">Arizona</span></p><br /> <p><strong>Peer-reviewed Publications</strong></p><br /> <ul><br /> <li>Raja, R., Slaughter, D.C., Fennimore, S.A. & Siemens, M.C. 2023. Real-time control of high-resolution micro-jet sprayer integrated with machine vision for precision weed control. <em>Biosystems Eng</em>. 228: 31-48.</li><br /> <li>Guerra, N., Fennimore, S.A., Siemens, M.C., & Goodhue, R.E. 2022. Band steaming for weed and disease control in leafy greens and carrots. <em> Sci</em>. 57(11): 1453-1459.</li><br /> </ul><br /> <p><strong>Patents</strong></p><br /> <ul><br /> <li>Heun J.T., Andrade-Sanchez P., & Sanyal D. 2023. Low-Cost Electronic Monitoring System of High Temporal Resolution In-Situ Soil Respiration. TLA Invention Disclosure UA23-221. Tucson, Ariz: Tech Launch Arizona, University of Arizona.</li><br /> <li>Bahr, N. A., Siemens, M.C., Godinez, Jr., & Fennimore, S.A. 2022. Method and apparatus for applying steam for soil disinfestation. TLA Invention Disclosure UA22-190. Tucson, Ariz: Tech Launch Arizona, University of Arizona.</li><br /> </ul><br /> <p><strong>Book Chapters</strong></p><br /> <ul><br /> <li>Fennimore, S.A. & Siemens, M.C. 2023. Mechanized weed management in vegetable crops. In <em>Encyclopedia of Smart Agricultural </em>Technologies, ed. Q. Zhang. Cham, Switzerland: Springer, Cham. https://doi.org/10.1007/978-3-030-89123-7_244-2.</li><br /> </ul><br /> <p><span style="text-decoration: underline;">California</span></p><br /> <p><strong>Peer-reviewed Publications</strong></p><br /> <ul><br /> <li>Tang M, Sadowski DL, Peng C, Vougioukas SG, Klever B, Khasla SDS, Brown PH and Jin Y. (2023). Tree-level almond yield estimation from high resolution aerial imagery with convolutional neural network. Front. Plant Sci. 14:1070699. <a href="https://doi.org/10.3389/fpls.2023.1070699">https://doi.org/10.3389/fpls.2023.1070699</a></li><br /> <li>Peng, C., Fei, Z., Vougioukas, SG. (2023). GNSS-Free End-of-Row Detection and Headland Manoeuvring for Orchard Navigation Using a Depth Camera. Machines 11(1), 84. <a href="https://doi.org/10.3390/machines11010084">https://doi.org/10.3390/machines11010084</a>.</li><br /> <li>Ghafoor, A., F. A. Khan, F. Khorsandi, M. A. Khan, H. M. Nauman, and M. U. Farid. Development and Evaluation of a Prototype Self-Propelled Crop Sprayer for Agricultural Sustainability in Small Farms. Sustainability, (2022), 14: 9204.</li><br /> <li>Khan, F. A., A. Ghafoor, M. A. Khan, M. U. Chattha, and F. Khorsandi. Parameter Optimization of Newly Developed Self-Propelled Variable Height Crop Sprayer Using Response Surface Methodology (RSM) Approach. Agriculture, (2022), 12(3):408.</li><br /> <li>Chou, H. Y., F. Khorsandi, S. G.Vougioukas, F. A.Fathallah . Developing and evaluating an autonomous agricultural all-terrain vehicle for field. experimental rollover simulations. Computers and Electronics in Agriculture, (2022), 194: 106735.</li><br /> <li>Khorsandi, F., G. D. M. Araujo, and F. Fathallah. A systematic review of youth and all-terrain vehicles safety in agriculture. Journal of Agromedicine, (2022), 1-23.</li><br /> <li>Araujo, G. D. M., F. Khorsandi, and F.A. Fathallah. Forces Required to Operate Controls on Agricultural All-Terrain Vehicles: Implications for Youth. Journal of Ergonomics, (2022), 1-15.</li><br /> <li>Dos Santos FFL, de Queiroz DM, Valente DSM, Khorsandi F, de Moura Araújo G. Analysis of Different Electric Current Frequencies in Soil Apparent Conductivity. Journal of Biosystems Engineering, (2023), 1-14.</li><br /> <li>Gibbs, J., C. Sheridan, F. Khorsandi*, A. Yoder. Emphasizing Safe Engineering Design Features of Quad Bikes in Agricultural Safety Programs. JASH, (2023), 29(2): 121-127.</li><br /> <li>Araujo, G. D. M., F. Khorsandi, F. A. Fathallah. Reach Evaluation to Operate Controls on Agricultural All-Terrain Vehicles: Implications for Young Operators. Journal of Safety Research, (2023), 84: 353-363.</li><br /> <li>Sirapoom Peanusaha, Alireza Pourreza, Yuto Kamya, Matthew Fidelibus. Grape Nitrogen retrieval by hyperspectral sensing–Part I: leaf level. Under review in the Journal of Remote Sensing of Environment.</li><br /> <li>Yuto Kamya, Alireza Pourreza, Sirapoom Peanusaha, Hamid Jafarbiglu, Matthew Fidelibus. Grape Nitrogen retrieval by hyperspectral sensing–Part II: canopy level. In-preparation.</li><br /> <li>Ahmadi A., Daccache A., Snyder R., Suvočarev K. (2022). Meteorological driving forces of reference evapotranspiration and their trends in California, Science of The Total Environment, 157823.</li><br /> <li>Emami, M.; Ahmadi, A.; Daccache, A.; Nazif, S.; Mousavi, S.-F.; Karami, H. County-Level Irrigation Water Demand Estimation Using Machine Learning: Case Study of California. Water 2022, 14, 1937.</li><br /> </ul><br /> <p><span style="text-decoration: underline;">Florida</span></p><br /> <p><strong>Peer-reviewed Publications</strong></p><br /> <ul><br /> <li><span style="text-decoration: underline;">Ghoveisi, H., </span><strong>M. Kadyampakeni</strong>, J. Qureshi, and L. Diepenbrock. 2023. Water use efficiency in young citrus trees on metalized UV reflective mulch compared to bare ground. Water 2023, 15, 2098. <a href="https://doi.org/10.3390/w15112098">https://doi.org/10.3390/w15112098</a></li><br /> <li><span style="text-decoration: underline;">Kwakye, S.</span> and <strong>M. Kadyampakeni</strong>. 2023. Impact of deficit irrigation on growth and water relations of HLB-affected citrus trees under greenhouse conditions. Water 15, 2085. <a href="https://doi.org/10.3390/w15112085">https://doi.org/10.3390/w15112085</a></li><br /> <li>Brewer, M. 2023. Citrus row-middle management using cover crops for suppressing weeds and improving soil. Ph.D. Dissertation, University of Florida, Gainesville, FL.</li><br /> <li>Teshome F.T., <span style="text-decoration: underline;">Bayabil H.K.</span>, Hoogenboom G., Schaffer B., Singh A., <strong>Ampatzidis Y.</strong>, 2023. Unmanned Aerial Vehicle (UAV) Imaging and Machine Learning Applications for Plant Phenotyping. Computers and Electronics in Agriculture, 212, 108064, <a href="https://doi.org/10.1016/j.compag.2023.108064">https://doi.org/10.1016/j.compag.2023.108064</a>.</li><br /> <li>Zhou C., <span style="text-decoration: underline;">Lee W.S.</span>, Liburd O.E., Aygun I., Zhou X., Pourreza A., Schueller J.K., <strong>Ampatzidis Y.</strong>, 2023. Detecting Two-spotted Spider Mites and Predatory Mites in Strawberry Using Deep Learning. Smart Agricultural Technology, 100229, <a href="https://doi.org/10.1016/j.atech.2023.100229">https://doi.org/10.1016/j.atech.2023.100229</a>.</li><br /> <li>Javidan S.M., Banakar A., Vakilian K.A., <strong>Ampatzidis Y.</strong>, 2023. Tomato leaf diseases classification using image processing and weighted ensemble learning. Agronomy Journal, <a href="http://doi.org/10.1002/agj2.21293">http://doi.org/10.1002/agj2.21293</a>.</li><br /> <li>Hariharan J., <strong><span style="text-decoration: underline;">Ampatzidis Y.</span></strong>, Abdulridha J., Batuman O., 2023. An AI-based Spectral Data Analysis Process for Recognizing Unique Plant Biomarkers and Disease Features. Computers and Electronics in Agriculture, 204, 107574, <a href="https://doi.org/10.1016/j.compag.2022.107574">https://doi.org/10.1016/j.compag.2022.107574</a>.</li><br /> <li>Momeny M., Neshat A.A., Jahanbakhshi A., Bakhtoor M.., <strong>Ampatzidis Y.</strong>, Radeva P., 2023. Grading and fraud detection of Saffron via learning-to-augment incorporated inception-v4 CNN. Food Control, 109554, <a href="https://doi.org/10.1016/j.foodcont.2022.109554">https://doi.org/10.1016/j.foodcont.2022.109554</a>.</li><br /> <li>Panta S., Zhou B., Zhu L., Maness N., Rohla C., Costa L., <strong>Ampatzidis Y.</strong>, Fontainer C., Kaur A., Zhang, L., 2023. Selecting non-linear mixed effect model for growth and development of pecan nut. Scientia Horticulturae, 309, 111614, <a href="https://doi.org/10.1016/j.scienta.2022.111614">https://doi.org/10.1016/j.scienta.2022.111614</a>.</li><br /> <li>Poudyal C., Sandhu H., <strong><span style="text-decoration: underline;">Ampatzidis Y.</span></strong>, Odero D.C., Arbelo O.C., Cherry R.H., Costa L., 2023. Prediction of morho-physiological traits in sugarcane using aerial imagery and machine learning. Smart Agricultural Technology, 100104, <a href="https://doi.org/10.1016/j.atech.2022.100104">https://doi.org/10.1016/j.atech.2022.100104</a>.</li><br /> <li>Vijayakumar V., <strong><span style="text-decoration: underline;">Ampatzidis Y.</span></strong>, Costa L., 2023. Tree-level Citrus Yield Prediction Utilizing Ground and Aerial Machine Vision and Machine Learning. Smart Agricultural Technology, 100077, <a href="https://doi.org/10.1016/j.atech.2022.100077">https://doi.org/10.1016/j.atech.2022.100077</a>.</li><br /> <li>Javidan S.M., Banakar A., Vakilian K.A., <strong>Ampatzidis Y.</strong>, 2023. Diagnosis of grape leaf diseases using automatic K-means clustering and machine learning. Smart Agricultural Technology, 100081, <a href="https://doi.org/10.1016/j.atech.2022.100081">https://doi.org/10.1016/j.atech.2022.100081</a>.</li><br /> <li>Momeny M., Jahanbakhshi A., Neshat A.A., Hadipour-Rokni R., Zhang Y-D., <strong>Ampatzidis Y.</strong>, 2022. Detection of citrus black spot disease and ripeness level in orange fruit using robust and generalized deep CNN based on learning-to-augment strategy. Ecological Informatics, 101829, <a href="https://doi.org/10.1016/j.ecoinf.2022.101829">https://doi.org/10.1016/j.ecoinf.2022.101829</a>.</li><br /> <li>Zhou X., <strong><span style="text-decoration: underline;">Ampatzidis Y.</span></strong>, Lee W.S., Zhou C., Agehara S., Schueller J.K., 2022. Deep learning-based postharvest strawberry bruise detection under UV and incandescent light. Computers and Electronics in Agriculture, 22, 107389, <a href="https://doi.org/10.1016/j.compag.2022.107389">https://doi.org/10.1016/j.compag.2022.107389</a>.</li><br /> <li>Longchamps L., Tisseyre B., Taylor J., Sagoo L, Momin Md.A., Fountas S., Manfrini L., <strong>Ampatzidis Y.</strong>, Schueller K.J., Khosla R., 2022. Yield sensing technologies for perennial and annual horticultural crops: a review. Precision Agriculture, https://doi.org/10.1007/s11119-022-09906-2.</li><br /> <li>Poudyal C., Costa L., Sandhu H., <strong><span style="text-decoration: underline;">Ampatzidis Y</span>.</strong>, Odero D.C., Arbelo O.C., Cherry R.H., 2022. Sugarcane yield prediction and genotype selection using UAV-based hyperspectral imaging and machine learning. Agronomy Journal, doi.org/10.1002/agj2.21133.</li><br /> <li>Abdulridha J., <strong><span style="text-decoration: underline;">Ampatzidis Y.</span></strong>, Qureshi J., Roberts P., 2022. Identification and classification of downy mildew development stages in watermelon utilizing aerial and ground remote sensing and machine learning. Frontiers in Plant Science, 13, 791018, <a href="https://doi.org/10.3389/fpls.2022.791018">https://doi.org/10.3389/fpls.2022.791018</a>.</li><br /> <li>Costa L., McBreen J., <strong><span style="text-decoration: underline;">Ampatzidis Y.</span></strong>, Guo J., Reisi Gahrooei M., Babar A., 2022. Using UAV-based hyperspectral imaging and functional regression to assist in predicting grain yield and related traits in wheat under heat-related stress environments for the purpose of stable yielding genotypes. Precision Agriculture, 23(2), 622-642, <a href="https://doi.org/10.1007/s11119-021-09852-5">https://doi.org/10.1007/s11119-021-09852-5</a>.</li><br /> <li>Costa L., Kunwar S., <strong><span style="text-decoration: underline;">Ampatzidis Y.</span></strong>, Albrecht U., 2022. Determining leaf nutrient concentrations in citrus trees using UAV imagery and machine learning. Precision Agriculture, 23(3), 854-875, <a href="https://doi.org/10.1007/s11119-021-09864-1">https://doi.org/10.1007/s11119-021-09864-1</a>.</li><br /> <li>Mirbod, O., <strong>Choi, D</strong>., Heinemann, P. H., Marini, R. P., & He, L. (2023). On-tree apple fruit size estimation using stereo vision with deep learning-based occlusion handling. Biosystems Engineering, 226, 27-42. (open access)</li><br /> <li>Yuan, W., <strong>Choi, D</strong>., Bolkas, D., Heinemann, P. H., & He, L. (2022). Sensitivity examination of YOLOv4 regarding test image distortion and training dataset attribute for apple flower bud classification. International Journal of Remote Sensing, 43(8), 3106-3130.</li><br /> <li>Yuan, W., <strong>Choi, D</strong>., & Bolkas, D. (2022). GNSS-IMU-assisted colored ICP for UAV-LiDAR point cloud registration of peach trees. Computers and Electronics in Agriculture, 197, 106966.</li><br /> <li>Zhang, H., He, L., Di Gioia, F., <strong>Choi, D</strong>., Elia, A., & Heinemann, P. (2022). LoRaWAN based Internet of Things (IoT) System for Precision Irrigation in Plasticulture Fresh-market Tomato. Smart Agricultural Technology, 100053.</li><br /> <li>Zhou, X., Y. Ampatzidis, W. S. Lee, C. Zhou, S. Agehara, and J. K. Schueller. 2022. Deep learning-based postharvest strawberry bruise detection under UV and incandescent light. Computers and Electronics in Agriculture 202 (2022) 107389. <a href="https://doi.org/10.1016/j.compag.2022.107389">https://doi.org/10.1016/j.compag.2022.107389</a>.</li><br /> <li>Patel, A.M., W. S. Lee, and N. A. Peres. 2022. Imaging and deep learning based approach to leaf wetness detection in strawberry. Sensors 2022, 22, 8558. <a href="https://doi.org/10.3390/s22218558">https://doi.org/10.3390/s22218558</a>.</li><br /> </ul><br /> <p><strong>Thesis/Dissertation</strong></p><br /> <ul><br /> <li>Uthman, Q.O. 2023. Management of huanglongbing-affected “Valencia” sweet oranges in sandy soils of central Florida: sorption kinetics and equilibria, uptake, and leaching of nutrients and imidacloprid in a Florida sandy soil. D. Dissertation, University of Florida, Gainesville, FL.</li><br /> </ul><br /> <p><span style="text-decoration: underline;">Michigan</span></p><br /> <p><strong>Peer-reviewed Publications</strong></p><br /> <ul><br /> <li>Dang, F., Chen, D., Lu, Y., Li, Z., 2023. YOLOWeeds: a novel benchmark of YOLO object detectors for multi-class weed detection in cotton production systems. Computers and Electronics in Agriculture 205, 107655.</li><br /> <li>Rahman, A., Lu, Y., Wang, H., 2023. Performance evaluation of deep learning object detectors for weed detection for cotton. Smart Agricultural Technology 3, 100126.</li><br /> </ul><br /> <p><span style="text-decoration: underline;">Mississippi</span></p><br /> <p><strong>Peer-reviewed Publications</strong></p><br /> <ul><br /> <li>Lucas Gay, <strong>Filip To</strong>, Joe Thomas, Sean Donohoe, “Inline Real-Time Moisture Sensing System for Gin Cotton”, Proceedings of National Cotton Council Belt-wide Cotton Conferences, New Orleans, January 10-12, 2023.</li><br /> <li><strong>Zhang, X.</strong>, Thayananthan, T., Usman, M., Liu, W., & Chen, Y. (2023, June). Multi-ripeness level blackberry detection using YOLOv7 for soft robotic harvesting. In Autonomous Air and Ground Sensing Systems for Agricultural Optimization and Phenotyping VIII (Vol. 12539, pp. 85-96). SPIE. <a href="https://doi.org/10.1117/12.2663367">https://doi.org/10.1117/12.2663367</a></li><br /> <li>Chakraborty, M., Pourreza, A., <strong>Zhang, X.</strong>, Jafarbiglu, H., Shackel, K. A., & DeJong, T. (2023). Early almond yield forecasting by bloom mapping using aerial imagery and deep learning. Computers and Electronics in Agriculture. (Accepted)</li><br /> <li>Peng, H., Zhong, J., Liu, H., Li, J., Yao, M., & <strong>Zhang, X.</strong> (2023). ResDense-focal-DeepLabV3+ enabled litchi branch semantic segmentation for robotic harvesting. Computers and Electronics in Agriculture, 206, 107691. <a href="https://doi.org/10.1016/j.compag.2023.107691">https://doi.org/10.1016/j.compag.2023.107691</a></li><br /> <li>Lu, S., Liu, X., He, Z., <strong>Zhang, X.</strong>, Liu, W., & Karkee, M. (2022). Swin-transformer-YOLOv5 for real-time wine grape bunch detection. Remote Sensing, 14(22), 5853. <a href="https://doi.org/10.3390/rs14225853">https://doi.org/10.3390/rs14225853</a></li><br /> </ul><br /> <p><strong>Book Chapters:</strong></p><br /> <ul><br /> <li><strong>Zhang, X.</strong> (2023). Robotics and Automation Technologies: Plant-machine interface. In Encyclopedia of Smart Agriculture Technologies (Zhang, Q. ed.), Springer. <a href="https://doi.org/10.1007/978-3-030-89123-7_124-1">https://doi.org/10.1007/978-3-030-89123-7_124-1</a></li><br /> <li>He, L., <strong>Zhang, X.</strong>, & Zahid, A. (2023). Chapter 2 – Mechanical management of modern planar fruit tree canopies. In Advanced Automation for Tree Fruit Orchards and Vineyards (Vougioukas, S. G., & Zhang, Q. ed.), Springer Book Series: Agriculture Automation and Control. <a href="https://doi.org/10.1007/978-3-031-26941-7_2">https://doi.org/10.1007/978-3-031-26941-7_2</a></li><br /> </ul><br /> <p><span style="text-decoration: underline;">New York</span></p><br /> <p><strong>Peer-reviewed Publications</strong></p><br /> <ul><br /> <li>Liu, E., Gold, K., Cadle-Davidson, L., Combs, D., & Jiang, Y. (2022, October). Near Real-Time Vineyard Downy Mildew Detection and Severity Estimation. In 2022 IEEE/RSJ International Conference on Intelligent Robots and Systems (IROS) (pp. 9187-9194). IEEE.</li><br /> <li>Kanaley, K., Paul, A., Combs, D., Liu, E., Jiang, Y., & Gold, K. (2022, December). Mapping Winegrape Disease Epidemics with SkySat and PlanetScope Imagery. In AGU Fall Meeting Abstracts (Vol. 2022, pp. IN43A-05).</li><br /> </ul><br /> <p><span style="text-decoration: underline;">Pennsylvania</span></p><br /> <p><strong>Peer-reviewed Publications</strong></p><br /> <ul><br /> <li>Hussain, M., He, L., Heinemann, P., & Schupp, J. (2022). Green fruit removal dynamics for robotic green fruit thinning end-effector development. <em>Journal of ASABE 65</em>(4), 779-788.</li><br /> <li>Mahmud, M. S., Zahid, A., He, L., Zhu, H., Heinemann, P., Choi, D., & Krawczyk, G. (2022). Development of an automatic airflow control system for precision sprayers based on tree canopy density. <em>Journal of ASABE 65</em>(6), 1225-1240.</li><br /> <li>Mu, X., He, L., Heinemann, P., Schupp, J., & Karkee, M. (2023). Mask R-CNN based king flowers identification for precise apple pollination. <em>Smart Agricultural Technology 4</em>, 100151.</li><br /> <li>Mahmud, M. S., He, L., Heinemann, P., Choi, D., & Zhu, H. (2023). Unmanned aerial vehicle based tree canopy characteristics measurement for precision spray applications. <em>Smart Agricultural Technology 4</em>(100153).</li><br /> <li>Hussain, M., He, L., Schupp, J., Lyons, D., & Heinemann, P. (2023). Green fruit segmentation and orientation estimation for robotic green fruit thinning of apples. <em>Computers and Electronics in Agriculture</em> 207, 107734.</li><br /> <li>Yuan, W., Hua, W., Heinemann, P.H. & He, L. (2023). UAV photogrammetry-based apple orchard blossom density estimation and mapping. <em>Horticulturae</em>, <em>9</em>(2), 266.</li><br /> <li>Mahmud, M.S., He, L., Zahid, A., Heinemann, P., Choi, D., Krawczyk, G. & Zhu, H., 2023. Detection and infected area segmentation of apple fire blight using image processing and deep transfer learning for site-specific management. Computers and Electronics in Agriculture, 209, 107862.</li><br /> </ul><br /> <p><strong>Book Chapter:</strong></p><br /> <ul><br /> <li>He, L., Zhang, X., & Zahid, A. (2023). Mechanical Management of Modern Planar Fruit Tree Canopies. In Book: Advanced Automation for Tree Fruit Orchards and Vineyards. Cham: Springer International Publishing.</li><br /> <li>He, L. (2022). Variable rate Technologies for Precision Agriculture. In Zhang, Q. (eds) Encyclopedia of Smart Agriculture Technologies. Springer, Cham. ISBN/ISSN: 978-3-030-89123-7</li><br /> <li>Gohil, H & He, L. (2023). Precision Irrigation for Orchards. In: Zhang, Q. (eds) Encyclopedia of Smart Agriculture Technologies. Springer, Cham. <a href="https://nam10.safelinks.protection.outlook.com/?url=https%3A%2F%2Fdoi.org%2F10.1007%2F978-3-030-89123-7_193-1&data=05%7C01%7Cluh378%40psu.edu%7C1ace4f06ded3414a3dc608db16bbf9f7%7C7cf48d453ddb4389a9c1c115526eb52e%7C0%7C0%7C638128768831093499%7CUnknown%7CTWFpbGZsb3d8eyJWIjoiMC4wLjAwMDAiLCJQIjoiV2luMzIiLCJBTiI6Ik1haWwiLCJXVCI6Mn0%3D%7C3000%7C%7C%7C&sdata=EIjDcA2ay4KqDF9CKGcXf9v7GxsTfM5j2QHWxzFKU4s%3D&reserved=0">ISBN/ISSN: 978-3-030-89123-7_193-1</a></li><br /> </ul><br /> <p><strong>Thesis and Dissertation: </strong></p><br /> <ul><br /> <li>Kittiphum Pawikhum (2022). Design of end-effectors for thinning apples in the green fruit stage. MS Thesis. The Pennsylvania State University.</li><br /> <li>Rashmi Sahu (2023). Development of vision system and end-effector for automatic bud thinning of apple tree: early crop load management. MS Thesis. The Pennsylvania State University.</li><br /> </ul><br /> <p><span style="text-decoration: underline;">Tennessee</span></p><br /> <p><strong>Peer-reviewed Publications</strong></p><br /> <ul><br /> <li>Rice, C. R., McDonald, S. T., Shi, Y., Gan, H., Lee, W. S., Chen, Y., & Wang, Z. (2022). Perception, Path Planning, and Flight Control for a Drone-Enabled Autonomous Pollination System. Robotics, 11(6), 144.</li><br /> <li>Rice, C.R., Gan, H., Wang, Z. (2023). Real-Time Path Planning and Collision-Free Flight</li><br /> </ul><br /> <p>Control for Drone-Assisted Autonomous Pollination Systems. Information Processing in Agriculture. (under review).</p><br /> <p><span style="text-decoration: underline;">Texas</span></p><br /> <p><strong>Peer-reviewed Publications</strong></p><br /> <ul><br /> <li>Ojo, M. O., and Zahid, A. 2023. Improving deep learning classifiers performance via preprocessing and class imbalance approaches in a plant disease detection pipeline. Agronomy, 13, 887.</li><br /> <li>Mahmud, M.S., Zahid, A., and Das, A.K. 2023. Sensing and Automation Technologies for Ornamental Nursery Crop Production: Current Status and Future Prospects. Sensors, 23, 1818. https://doi.org/10.3390/s23041818</li><br /> <li>Ojo, M. O., and Zahid, A. 2022. Deep learning in controlled environment agriculture: A review of recent advancements, challenges, and prospects, Sensors, 22(20), 7965</li><br /> </ul><br /> <p><strong>Book Chapters</strong></p><br /> <ul><br /> <li>Zahid, A., and Mahmud, M.S. 2023. LiDAR Sensing and its Applications in Agriculture. Q. Zhang (ed.), Encyclopedia of Smart Agriculture Technologies, Springer Nature Switzerland</li><br /> </ul><br /> <p><span style="text-decoration: underline;">Washington</span></p><br /> <p><strong>Peer-reviewed Publications</strong></p><br /> <ul><br /> <li>Molaei, B., A. K. Chandel, R. Troy Peters, L. R. Khot, A. Khan, F. Maureira, and C. Stockle. 2023. Investigating the application of artificial hot and cold reference surfaces for improved ETc estimation using the UAS-METRIC energy balance model. <em>Agricultural Water Management</em>, 284, 108346. <a href="https://doi.org/10.1016/j.agwat.2023.108346">https://doi.org/10.1016/j.agwat.2023.108346</a>.</li><br /> <li>Chandel<sup>l</sup>, A.K., M.M. Moyer, M. Keller, L.R. Khot, and G-A. Hoheisel. 2022. Soil and climate geographic information system data-derived risk mapping for grape phylloxera in Washington state. <em>Frontiers in Plant Science,</em> <em>13, 827393-827393</em> <a href="https://doi.org/10.3389/fpls.2022.827393">https://doi.org/10.3389/fpls.2022.827393</a></li><br /> <li>Chandel, A.K., A.P. Rathnayake, and L.R. Khot. 2022. Mapping apple canopy attributes using aerial multispectral imagery for precision crop inputs management. <em>Acta Horticulturae</em>. 1346, 537-546, <a href="https://10.17660/ActaHortic.2022.1346.68">https://10.17660/ActaHortic.2022.1346.68</a></li><br /> <li>Chandel, A. K., Amogi, B., Khot, L., Stockle, C. O., and R. T. Peters. 2022. Digitizing Crop Water Use with Data-Driven Approaches. <em>Resource Magazine, 29</em>(4), 14--16.</li><br /> <li>Kalyanaraman*, A., M. Burnett, A. Fern, L. Khot, and J. Viers. 2022. Special report: The AgAID AI institute for transforming workforce and decision support in agriculture. <em>Computers and Electronics in Agriculture,</em> 197, 106944</li><br /> <li>McCoy, M. L., G.-A. Hoheisel, L. R. Khot, and M. M. Moyer*. 2022. Adjusting air-assistance and nozzle style for optimized airblast sprayer use in eastern Washington vineyards. <em>Catalyst: Discovery into Practice</em>, 6(1): 9-19 <a href="https://doi:10.5344">https://doi:10.5344/catalyst.2021.21001</a> <em>(Featured on cover page)</em></li><br /> <li>Molaei, B., A. Chandel<sup>l</sup>, R.T. Peters*, L.R. Khot, and J.Q. Vargas<sup>l</sup>. Investigating lodging in Spearmint with overhead sprinklers compared to drag hoses using the texture feature from low altitude RGB imagery. <em>Information Processing in Agriculture,</em> 9(2): 335-341 <a href="https://doi.org/10.1016/j.inpa.2021.02.003">https://doi.org/10.1016/j.inpa.2021.02.003</a> </li><br /> <li>Molaei, B., R.T. Peters*, L.R. Khot, and C. Stockle. 2022. Assessing suitability of auto-selection of hot and cold anchor pixels of the UAS-metric model for developing crop water use maps. <em>Remote Sensing</em>, 14(18), 4454; <a href="https://doi.org/10.3390/rs14184454">https://doi.org/10.3390/rs14184454</a></li><br /> <li>Ranjan<sup>l</sup>, R., R. Sinha<sup>l</sup>, L.R. Khot*, and M. Whiting. 2022. Thermal-RGB imagery and in-field weather sensing derived sweet cherry wetness prediction model. <em>Scientia Horticulturae</em>, 294, 110782 <a href="https://doi.org/10.1016/j.scienta.2021.110782">https://doi.org/10.1016/j.scienta.2021.110782</a></li><br /> <li>Rathnayake<sup>l</sup>, A. P., A. Chandel<sup>l</sup>, M. Schrader<sup>l</sup>, G.-A. Hoheisel and L. R. Khot*. 2022. Air-assisted velocity profiles and perceptive canopy interactions of commercial airblast sprayers used in Pacific Northwest perennial specialty crop production. <em>CIGR e-journal, 24 (1) 7039.</em></li><br /> <li>Rathnayake<sup>l</sup>, R. K Sahni<sup>l</sup>, L. R. Khot*, G.-A. Hoheisel and H. Zhu. 2022. Intelligent sprayer spray rates optimization to efficiently apply chemicals in modern apple orchards. <em>Journal of the ASABE</em>, 65 (6): 1-10. <a href="https://doi.org/10.13031/ja.14654">https://doi.org/10.13031/ja.14654</a></li><br /> <li>Sahni<sup>l</sup>, R. K, R. Ranjan<sup>l</sup>, L. R. Khot*, G.-A. Hoheisel and M. Grieshop. 2022. Reservoir units optimization in pneumatic spray delivery-based fixed spray system for large-scale commercial adaptation. <em>Sustainability</em>, 14, 10843. <a href="https://doi.org/10.3390/su141710843">https://doi.org/10.3390/su141710843</a></li><br /> <li>Schrader<sup>l</sup>, M.J., A.P. Rathnayake<sup>l</sup>, and L. R. Khot. 2022. Horticultural oil thermotherapy delivery system for perennial specialty crops: a-proof-of-concept and preliminary results. <em>Applied Engineering in Agriculture,</em> 38(2), 461-468.</li><br /> <li>Schrader<sup>l</sup>, M.J., P. Smytheman, E.H. Beers, and L.R. Khot*. 2022. An open-source low-cost imaging system plug-in for pheromone traps aiding remote insect pest population monitoring in fruit crops. <em>Machines</em>, <em>10</em>(1), 52. <a href="https://doi.org/10.3390/machines10010052">https://doi.org/10.3390/machines10010052</a></li><br /> <li>Sinha<sup>l</sup>, R., J. Quiros Vargas<sup>l</sup>, S. Sankaran and L. R. Khot*. 2022. High resolution aerial photogrammetry based 3D mapping of fruit crop canopies for precision inputs management. <em>Information Processing in Agriculture,9(1): 11-23 </em><a href="https://doi.org/10.1016/j.inpa.2021.01.006">https://doi.org/10.1016/j.inpa.2021.01.006</a>.</li><br /> <li>Worasit, S., A. Marzougui, A. A. Bates, B. Schroeder, L. R. Khot and S. Sankaran*. 2022. Identification of volatile biomarkers for high-throughput sensing of soft rot and Pythium leak diseases in stored potatoes. <em>Food Chemistry, </em>370, 130910 <a href="https://doi.org/10.1016/j.foodchem.2021.130910">https://doi.org/10.1016/j.foodchem.2021.130910</a></li><br /> <li>Kang, C., Diverres, G., Karkee, M., Zhang, Q., & Keller, M. (2023). Decision-support system for precision regulated deficit irrigation management for wine grapes. <em>Computers and Electronics in Agriculture</em>, <em>208</em>, 107777.</li><br /> <li>Borrenpohl, D., & Karkee, M. (2023). Automated pruning decisions in dormant sweet cherry canopies using instance segmentation. <em>Computers and Electronics in Agriculture</em>, <em>207</em>, 107716.</li><br /> <li>Bayano-Tejero, S., Karkee, M., Rodríguez-Lizana, A., & Sola-Guirado, R. R. (2023). Estimation of harvested fruit weight using volume measurements with distance sensors: A case study with olives in a big box. <em>Computers and Electronics in Agriculture</em>, <em>205</em>, 107620.</li><br /> <li>Mu, X., He, L., Heinemann, P., Schupp, J., & Karkee, M. (2023). Mask R-CNN based apple flower detection and king flower identification for precision pollination. <em>Smart Agricultural Technology</em>, <em>4</em>, 100151.</li><br /> <li>Bayano-Tejero, S., Karkee, M., Rodríguez-Lizana, A., & Sola-Guirado, R. R. (2023). Estimation of harvested fruit weight using volume measurements with distance sensors: A case study with olives in a big box. <em>Computers and Electronics in Agriculture</em>, <em>205</em>, 107620</li><br /> <li>Lu, S., Liu, X., He, Z., Zhang, X., Liu, W., & Karkee, M. (2022). Swin-Transformer-YOLOv5 for Real-Time Wine Grape Bunch Detection. <em>Remote Sensing</em>, <em>14</em>(22), 5853.</li><br /> <li>Guo, J., Duan, J., Yang, Z., & Karkee, M. (2022). De-Handing Technologies for Banana Postharvest Operations—Updates and Challenges. <em>Agriculture</em>, <em>12</em>(11), 1821.</li><br /> </ul><br /> <p><strong>Thesis/Dissertations</strong></p><br /> <ul><br /> <li>Borrenpohl, D. (2023). Automated Pruning Decisions in Dormant Sweet Cherry Canopies using Instance Segmentation. <em>MS Thesis, Washington State University.</em></li><br /> <li>Bhattarai, U. (2023). Robotic Blossom Thinning System for Tree Fruit Crops. <em>PhD Dissertation,</em> Washington State University.</li><br /> <li>Kang, C. (2023). Decision-Support System for Water Stress Assessment and Deficit Irrigation Management in Wine Grapes. <em>PhD Dissertation,</em> Washington State University.</li><br /> </ul><br /> <p><strong>Books and Book Chapters</strong></p><br /> <ul><br /> <li>Sahni, R.K., and <strong>R. Khot*</strong> 2023. Fixed spray systems for perennial specialty crops. In: Zhang, Q. (eds) Encyclopedia of Smart Agriculture Technologies. Springer, Cham. <a href="https://doi.org/10.1007/978-3-030-89123-7_195-1">https://doi.org/10.1007/978-3-030-89123-7_195-1</a></li><br /> <li>Karkee, M., Majeed, Y., & Zhang, Q. (2023). Advanced Technologies for Crop-Load Management. In <em>Advanced Automation for Tree Fruit Orchards and Vineyards</em>(pp. 119-149) (Editors Stavros Vougioukas and Qin Zhang). Cham: Springer International Publishing.</li><br /> <li><strong>Karkee</strong>, M., &Silwal, A. (2023). Robotic Fruit Harvesting. <em>In: Zhang, Q. (eds) Encyclopedia of Smart Agriculture Technologies</em>. Springer, Cham. <a href="https://doi.org/10.1007/978-3-030-89123-7_139-1">https://doi.org/10.1007/978-3-030-89123-7_139-1</a></li><br /> </ul>Impact Statements
- Washington - Labor shortage and work-induced safety are two of major challenges in Washington State agriculture. Washington State University (WSU) team has focused on developing mechanization and automation solutions for mechanical harvest of apples for fresh market, and also worked closely with equipment manufacturers to support technology transfer from research to products. For example, WSU team has collaborated with FFRobotics Inc. (Israel) in developing and testing a full scale (12-armed) robotic machine for apple picking. In the test with planner apple trees in WA, it achieved a 100% accuracy in detecting fruit in canopies with a machine vision system and about 70% success rate in picking. Growers witnessed field tests have shown their willingness to adopt a robotic picking system like this one as it can perform the majority of picking job to allow growers maintain a small proportion of current workforce necessary for apple harvesting. The result is promising for commercial adoption of such a robotic picking system in near future, which will make a huge positive impact to apple industry to minimize the need and cost associated with farm labor and improve long term sustainability of the industry. In addition, the WSU team has partner with VineTech (Prosser, WA) to integrate the automated grape green shoot thinning system with existing commercial shoot thinning machine and evaluate in commercial vineyards so that the machine could be commercialized with automated thinning capability. In terms of precision orchard management, crop physiology sensing system-based apple fruit surface temperature outputs are being integrated into commercial hardware (Wise Conn, Spokane, WA) for automated heat stress mitigation along with under tree irrigation.