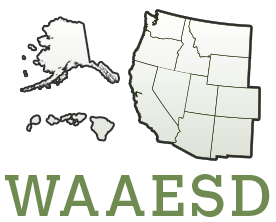
W4009: Integrated Systems Research and Development in Automation and Sensors for Sustainability of Specialty Crops
(Multistate Research Project)
Status: Active
W4009: Integrated Systems Research and Development in Automation and Sensors for Sustainability of Specialty Crops
Duration: 10/01/2023 to 09/30/2028
Administrative Advisor(s):
NIFA Reps:
Non-Technical Summary
Statement of Issues and Justification
The Need:
Increased demand for safe and high-quality food, pressure from global competition, and the need to preserve sustainability in natural resources and the environment represent formidable challenges for agricultural production in the U.S. Additionally, the decline in the availability of skilled and unskilled farm labor is a continuing trend with a very significant negative impact on crop production. Specialty crops, in particular, are major sources of nutrients that are paramount to achieving the nutrition security that USDA has highlighted. USDA recommendations suggest two servings per day of fresh fruits and 2 to 4 servings per day of fresh vegetables. Nuts also provide significant health benefits. However, much of the population in the U.S. cannot meet the USDA standards due to limited access to and high prices of these crops. Over the past 3 to 4 decades, the U.S. kept increasing the percentage of imported fruits and vegetables, resulting in over 40% of total fruits and vegetables in 2021. Considering the fragility of global agrifood supply chains, especially during unexpected global pandemics, such a high importing ratio introduces extreme uncertainty. We should develop productive, resilient, and sustainable specialty crop production systems to avoid potential consequences.
Toward this goal, producers and processors are urgently seeking new advancements in tools, methodologies, and systems that will aid them during production, harvesting, sorting, storing, processing, packaging, marketing, and transportation while maintaining competitiveness in production costs. There is a continuing need for sufficient and effective sensors, optimized assistive mechanization, and automation systems for specialty crops (fruits, vegetables, tree nuts, dried fruits, and nurseries). This is because many of the underlying biological processes related to the quality and condition of fruits and vegetables are not readily transferred into engineering concepts. Biological variability, coupled with various environmental factors, creates challenges and opportunities for developing sensors and automation systems for effective implementation at various stages of the production, harvest, and postharvest handling chain. Additionally, obtaining measurements of internal biological factors by using external, non-destructive sensors is not simple. Such devices or processes must adapt to a wide variation in shape, size, maturity, and other parameters of the commodity being processed.
Although modern crop architectures, systems, and growing practices are slowly being upgraded to accommodate mechanized and automated production, a large portion of the production system, such as orchards, is still based on conventional, labor-intensive production practices. In contrast to grain crop production, it is a challenge for any single specialty crop sector to afford the cost of research, development, and commercialization of this complex level of automation due to its relatively small scale. Thus, public entities must assist this economically vital agricultural sector with integrated research and development in sensing, mechanization, and automation using an integrated approach.
Importance of the Work:
The steady increase in global competition and the recent decrease in available labor has amplified the need for new technologies in specialty crop production. The specialty crop industry in the United States faces significant challenges to remain competitive; improving production efficiency is critical to keeping the specialty crop industry thriving. A system-wide approach to developing automation for the specialty crop industry is critically needed to address economic and environmental sustainability challenges. The proposed five-year project will address research and outreach needs for the specialty crop industry in these areas, working with researchers, manufacturers, and producers. Approval of this project will allow researchers and industrial partners to share their results and to plan future efforts in a coordinated manner to help develop new technologies to improve efficiency, reduce redundancies, and help promote better use of expertise.
Related, Current and Previous Work
Specialty crop mechanization history
In the 1800s, U.S. agriculture was powered by human and animal labor. With the introduction of the steam engine and internal combustion engine, mechanical devices rapidly became the power source for agricultural operations. By the early 1900s, mechanical power had a strong economic case (Reynoldson, 1933). The need for mechanization of crops (primarily grain and fiber crops) intensified after World War II (Cooper et al., 1947). By the later half of the 20th century, grain and fiber agriculture employed very few farm laborers (National Rural Center 1980).
In contrast, specialty crops (fruits, nuts, vegetables, berries, nurseries, dried fruits, and herbs) continued to rely on human labor for most operations. Production operations were diverse, difficult, and required judgment and dexterity- attributes well suited for human labor and poorly suited for mechanization (Grise and Johnson 1973). There were a few examples of successful mechanization in production systems, such as tree shakers (Pellerin et al., 1978), fruit conveyors (Rehkugler et al., 1976), and orchard sprayers (Giles et al., 1987; Byers 1989). But most of the automation effort was in postharvest handling and processing. There were many research and development efforts in quality inspection of fruits such as apples (Cavalieri et al., 1998, Guyer et al., 1991), berries (e.g., Timm et al., 1998) and other fruits and vegetables.
U.S. automation and mechanization research for specialty crop production all but ceased in the late 1980s and early 1990s. Research and development continued in Europe and Japan, outpacing the U.S. in production operations such as pruning, harvesting, and handling specialty crops. In the late 1990s and continuing to the present, the farm labor supply for specialty crops has continued to shrink, and there emerged an urgent and ongoing need for automation to replace human labor. The introduction of the USDA Specialty Crop Research Initiative (SCRI) program in 2008 and support from NIFA for research in agricultural robotics (Vougioukas, 2019) and cyberphysical systems (Schueller, 2021a) through the AFRI and National Robotics Initiative and Cyberphysical Systems programs have revitalized engineering-related research in the specialty crop area. Many projects related to this multi-state project have been funded, and research results have been published extensively, while some projects have led to the adoption of investigated technologies. For example, mechanized thinning of fruit has been proven to increase fruit size and quality in peaches, with significant reductions in labor required (Baugher et al., 2010, Miller et al., 2011, Schupp et al., 2011). By 2012, 60 "Darwin" and "PT-250" string thinners had been commercially sold in North America due to these studies. Also, the research knowledge and ecosystem created through such projects have contributed to a boom in agricultural robotics and automation start-up companies.
Assessment of plant and crop properties in the field
Substantial advancements have been made for infield plant and crop property assessment in the past decade to support not only digital agriculture but also high throughput plant phenotyping (HTP) for genetics studies and breeding programs (Yang et al., 2017; Guo et al., 2021; Li et al., 2021; Li et al., 2014; Sankaran et al., 2015; Sishodia et al., 2020; Xu & Li, 2022; Zhou et al., 2022). These efforts have resulted in various novel sensing systems that can be categorized by system sensing range, modality, and purpose.
Based on the sensing range, the systems are classified into two types: proximal and remote. Proximal systems include handheld devices (Siddiqua et al., 2022), internet of thing (IoT) sensor network (Navarro et al., 2020), ground mobile systems (e.g., unmanned ground vehicles, UGVs) (Xu & Li, 2022), gantry- and tower-based systems (Shafiekhani et al., 2017), and low-altitude unmanned aircraft systems (UAS) (Sankaran et al., 2015; Zheng et al., 2021), and remote systems include high-altitude UAS and satellite-based systems (Silva et al., 2021). The proximal systems usually cover a small to medium sized area (e.g., up to several acres) with a high spatial resolution (e.g., organ level details) and temporal resolution (e.g., hourly to daily), whereas the remote systems scan large areas (e.g., regional to global level) with a moderate spatiotemporal resolution. These two types of system provide complementary features to enable data-driven approaches for both precision management operations in the field (e.g., selective harvesting and precision pesticide application) and strategic planning of the specialty crop industry (e.g., the estimation of regional disease presence and influence on yield).
Based on the sensing modality, the systems are grouped as four types: optical, acoustic, olfactory, and haptic. Optical sensors are the most widely used sensors for plant and crop property measurement in the field because of their non-destructive sensing manner and rich information (i.e., imaging) (Li et al., 2014). Extensive studies have demonstrated the usefulness of a wide range of optical sensors (e.g., RGB color, stereo, depth, multi-/hyper-spectral, and thermal imaging) for characterizing plant morphology (e.g., plant height), physiology, and development in the field (Li et al., 2014; Xie & Yang, 2020). These studies also lay a solid foundation to enable the translation of plant and crop measurements into actionable items by executing units such as sprayers and robots. Acoustic and olfactory sensors are also investigated to accommodate situations where optical sensing may not be effective such as underground insect detection (Roberts & Wickings, 2022) and mapping and asymptomatic disease detection (Cellini et al., 2017). While certain successes were achieved, confounding environmental factors (e.g., wind turbulence and background noises) are widely acknowledged to be key challenges for accurate and reliable measurements. Haptic sensing recently receives additional attentions primarily because of the advances in soft robotic grippers for agrifood applications such as fruit picking (Gunderman et al., 2022). When a gripper touches an object, the forces applied to the object surface is measured and fed back to the control system for improved handling (e.g., avoidance of extra external impacts causing bruises). This allow assessing some properties (e.g., optimal picking force for delicate fruits like caneberries) that cannot be measured or are not well defined using other sensing modalities.
Based on the sensing purposes, the systems are developed for three major aspects: yield estimation, quality assessment, and stress detection. Yield estimation and quality assessment are crucial to specialty crop production to determine logistics and business strategies for profitability of growers. Several products have been commercialized in recent years for trellised crops such as apples (Gao et al., 2022; Mirbod et al., 2023) and grapes (Nellithimaru & Kantor, 2019) as well as other tree fruit crops like citrus (Zhang et al., 2022), showing research efforts have resulted in technology translation and adoption to generate impacts for production systems. Major constraints still remain in challenging situations where sensing is the major barrier. For example, crop load is underestimated due to occlusions. Stress detection is another key aspect related to in-season management. Stresses can be further broken down into abiotic (e.g., nutrient deficiency) and biotic (e.g., disease). While symptomatic stress detection and quantification can be accurately achieved (Liu, Gold, Cadle-Davidson, et al., 2022; Liu, Gold, Combs, et al., 2022), early- and pre-symptomatic stress characterization is recognized as more valuable for production systems yet understudied (Terentev et al., 2022). Current focuses are to use advanced sensing technologies (e.g., multi-/hyper-spectral imaging) to reveal stress signals and have demonstrated promising results.
In addition to sensing system advances, data analytics of large, complex sensing data for plant and crop characterization has been improved dramatically because of recent breakthroughs in deep learning (DL) and artificial intelligence (AI) (Jiang & Li, 2020). Many DL models have been utilized or tailored to provide accurate analysis results in agricultural applications such as fruit detection and counting (Gao et al., 2022; Zhang et al., 2022; Olenskyi et al., 2022), stress identification and severity estimation (Liu, Gold, Cadle-Davidson, et al., 2022; Liu, Gold, Combs, et al., 2022), and 3D reconstruction (Smitt et al., 2022). Key challenges remain in computational efficiency on edge devices (e.g., embedded systems) for field operations, creating, managing (Huang et al., 2018) and standardizing datasets (Joshi et al., 2022), and data annotation requiring particular knowledge expertise (such as disease differentiation).
Assessment of crop attributes during postharvest operations
Postharvest needs to measure quality and inspect for damage have directed specialty crops' physiological and metabolic study. Image analysis has formed the basis of many sensor designs. Machine vision systems have been used to detect defects in prunes (Delwiche et al., 1990), peaches (Miller and Delwiche, 1991), and pistachio nuts (Pearson and Slaughter, 1996). Vision systems have also been used to grade various fruits and vegetables; potatoes (Tao et al., 1995), apples (Rehkugler and Throop, 1986), mushrooms (Heinemann et al., 1994), and other crops. Vision systems coupled with light energy transmitted through fruit have been used to detect watercore (Throop et al., 1994) and the internal quality of mango fruit (Reyes et al., 2000). Durand-Petiteville et al. (2017) developed image-processing algorithms to segment strawberry flesh and calyx. The parameters of firmness, color, and bruise damage are recurring themes in reported work.
Firmness is associated with mechanical properties, and many researchers looked for connections between mechanical properties and sensory firmness. Delwiche et al. (1987) tested devices to measure peach firmness and Timm et al. (1996) developed portable devices to measure cherry firmness. Non-destructive assessment of firmness is highly desirable, and multiple approaches have been studied to measure the mechanical properties in a non-destructive manner, such as impact loading (Lu and Abbott, 1997) and vibration modal response (Lu and Abbott (1996). A few indirect measures of firmness have been determined (e.g., near-infrared reflectance (Lu et al., (2000)). Investigators developed more universal fruit firmness sensors (Delwiche et al., 1987, Delwiche et al., 1996).
Bruising is a common defect in fruits. Bruise detection is an important quality attribute for fruit and vegetables in terms of consumer acceptance and storing the produce. Understanding the type and severity of mechanical loads that would cause bruising is important to designing fruit and vegetable handling systems. Upchurch et al. (1987) developed a detection device based on ultrasound reflection. Schulte et al. (1994) measured threshold levels of peach impact damage. Slaughter et al. (1993) quantified vibration injury to pears. Rehkugler et al. (1971) and Upchurch (1991) used optical devices to locate bruises on fruit. Upchurch et al. (1990) developed a method to measure bruise volume in situ using spectrophotometers. Li et al. (2018) used hyperspectral imaging with an improved watershed segmentation algorithm to detect early bruises on peaches.
Color is one of fruits and vegetables most important consumer quality attributes. Color is also a maturity and harvest indicator. Color, both visible and infrared, has been the basis for numerous fruit and vegetable quality sensors. Delwiche et al. (1987) quantified color and maturity in peaches. Thai et al. (1990) monitored changes to tomatoes in storage. Infrared light has been used to measure firmness and sugar content (Lu, 2001), internal quality in peaches, nectarines, and kiwifruit (Slaughter and Crisosto, 1998).
Some researchers have used novel methods to measure fruit quality. Cho and Krutz (1989) and Ray et al. (1993) used NMR techniques. Timm et al. (1991) evaluated various technologies to detect pits in tart cherries. Marrazzo et al. (2007) adapted an electronic nose design to differentiate between apple cultivars. Li et al. (2007) used sensor data fusion techniques to distinguish between damaged and healthy apples utilizing an electronic nose and a "zNose". Lefcourt and Siemens (2017) determined specifications for low-cost imaging systems to detect fecal contamination in produce fields. Donis-González used computer tomography to characterize chestnut internal structure (Donis-González et al., 2012a; 2012b; Donis-González et al., 2014; 2015).
Many other biological attributes have been studied. Allison et al. (1987) measured internal pressures in maturing pecans. Pearson et al. (1996) studied hull adhesion forces in pistachio nuts. Ikediala et al. (2000) investigated the dielectric properties of apple and codling moth larvae to develop a method to eradicate the insect from apples. Constante et al. (2016) used a deep learning architecture with noise-compensated learning for strawberry classification in the food processing industry.
Developing machine-friendly crop systems
Specialty crop mechanization is very challenging due to the differences between individual plants, so model-based approaches (such as those used in factories) will fail (Fisher, 1992). Complex architectures result in the absence of consistent patterns that can be followed in finding limbs of trees or fruits/nuts on a limb and thus poses technological challenges to orchard mechanization. A conventional engineering solution to the problem is to develop special machines for each operation; however, the specialized equipment and small markets discourage innovation and commercialization by private sector technology providers. Therefore, mechanization solutions for tree fruit/nut production will be more successful if cropping systems are modified to accommodate the machinery rather than designing the machinery to fit all situations. Robinson et al. (1990) discussed the potential benefits of pruning trees to ease automated harvest operations. Schupp and Baugher (2011) and Miller et al. (2011) showed that narrowing canopy widths to make them more two-dimensional has increased the viability of mechanized fruit thinning. Baugher et al. (2003) and Crassweller and Smith (2013) described new training system designs. Castillo-Ruiz et al. (2017) analyzed different tree structures in three pruning treatments to compare the performance of two mechanical olive harvesters. Schupp et al. (2017) developed a pruning severity index (limb-to-trunk ratio - LTR) for apple trees calculated from the sum of the cross-sectional area of all branches on a tree at 2.5 cm from their union to the central leader divided by the cross-sectional area of its central leader at 30 cm from the graft union. The LTR provides a measurable way to define and create different levels of pruning severity and achieve consistent outcomes, allowing greater accuracy and precision to dormant pruning of tall spindle apple trees. This metric is also a necessary step in developing autonomous pruning systems. Recent studies aimed at providing data and methods to enable the model-based design of fruit-picking robotic arms. Arikapudi et al. (2016) developed technology to measure the spatial distributions of fruits in tree canopies, and Vougioukas et al. (2016) calculated fruit linear reachability in high-density orchards. Bloch, Degani, and Bechar (2018) used tree growth modeling software to co-optimize robotic manipulators and tree structure (Y-trellis branch angles) to maximize fruit-picking efficiency.
Design of automated and mechanized systems
Major mechanization and automation research areas include harvesting, weeding, thinning, pruning, fertilizing, planting and transplanting, robot mobility and robot-crop interaction, pesticide application, and irrigation.
Mechanized harvesting is a major goal for specialty crop production. Harvesting is one of the most labor-intensive operations and has a high timeliness cost associated with delay (Oliveira et al., 1993). One approach is harvest-assist technology, bridging the gap between fully manual and automated harvest. Such units for apple harvesting were developed by researchers (Schupp et al., 2011, Lewis et al., 2012, Heinemann et al., 2012). These units mount on a platform and utilize vacuums to transport apples from the picker through tubes to the apple bin. Results have shown improved picker efficiency and reduced potential for injury. Ye et al. (2017) developed bin-carrying harvest aids for orchard operations. Robotic harvest aids can reduce non-productive walking times during berry harvesting. Khosro Anjom et al. (2018) developed algorithms to predict the picking time of strawberry pickers so that robotic harvest aids can be proactively dispatched to them. Peng et al. (2022) developed and field-tested such strawberry transport robots acting as strawberry harvest aids. Zhang et al. (2016) developed an economical harvest-assist device that mounts on a two-person picking platform, increasing harvest efficiency by 78% by eliminating ladders and collection buckets. Fei et al. (2021) developed a collaborative robotic platform that increased efficiency by up to 25%, compared to a manually controlled platform. Recent work has re-visited the catch-and-shake concept. Larbi et al. (2015) improved a two-unit sweet cherry harvester using a continuous shaking mechanism in commercial operation. He et al. (2017) and Zhang et al. (2020) developed a new shake-and-catch harvester (shaking targeted tree limbs and catching the detached fruits) for fresh market apples in trellis-trained apple trees. Robotic selective harvesting has been a research goal for many years. Bac et al. (2014) and Kootstra et al. (2021) provided state-of-art reviews. Several participants of this proposal are active in this area. For example, Silwal et al. (2017) developed a low-cost robotic apple harvester for modern apple orchards with planar canopies, and Hohimer et al. (2019) field-tested soft robotic grippers for fruit harvesting.
Automated weed control applications for Western cropping systems were reviewed by Fennimore et al. (2013), and field applications were reported. Fennimore et al. (2016) reviewed technologies for automated weed control and concluded that robotic weeding systems show great promise for use in specialty crops. Fennimore and Siemens (2022) informed on the advances and current state of robotic weeding systems for vegetable crops. They reported that the latest commercially available technologies reduced weed densities by more than 70% and lowered hand weeding times by 30-49%. Raja et al. (2019) developed a novel crop signaling technique that showed great promise for reliable crop/weed differentiation in fields with heavy weed infestation. Siemens et al. (2021) developed a high precision sprayer for automated weeding in specialty crops. The device was designed to spot apply herbicides at the 1-cm scale level of resolution while traveling at speeds that are viable for commercial farming operations (2 mph). In laboratory testing, they reported weed control efficacy was greater than 95% (3 species), percentage of off-target spray was less than 3% and no crop injury was observed.
Mechanized thinning of fruit has been proven to increase fruit size and quality in peaches, with significant reductions in labor required (Baugher et al., 2010, Miller et al., 2011, Schupp et al., 2012). Kon et al., (2013) determined optimum string thinner spindle speeds for mechanical thinning of apple blossoms based on thinning response and minimized injury to spur leaves. They determined that mechanical string thinning might be a viable treatment in organic apple production, where chemical thinners are limited. Lyons et al. (2015) developed robotic peach blossom thinning algorithms based on heuristics.
Several researchers have studied planting and transplanting operations. Especially in nursery operations, reducing labor used for transplanting is a critical need. Kutz et al. (1987) described a method for transplanting bedding plants. Gautz and Wong (1993) described a system to open flower pollen vessels during micropropagation. Kondo et al. (1996) described a vision system for cutting chrysanthemums. Sakaute (1996) developed an automated transplanter in Japan. Wang et al. (1997) developed a vision-guided robotic system for separating and transplanting sugarcane shoots from tissue culture.
Autonomous mobility is required to deploy automated systems efficiently and safely in orchards. Guidance is a critical part of vehicle mobility (Vougioukas, 2019). GPS-based guidance in open fields is commercial, off-the-shelf technology. In orchards and vineyards, guidance is more challenging due to unreliable or unavailable precision GPS signals. Bergerman et al. (2012) developed autonomous orchard platforms to perform multiple tasks, which relied on artificial landmarks for turning between rows. More recent autonomous vehicles have relied primarily on machine vision to move (Radcliffe et al., 2018; Fei & Vougioukas, 2022). At a mission-planning level, Bochtis et al. (2015) developed route-planning algorithms for autonomous vehicles in orchards, and Seyyedhasani and Dvorak (2017) developed a method that optimizes vehicle fleets' routing in specialty crop production fields. Regarding safety in the presence of humans, Vougioukas et al. (2016) developed a safety system for detecting and localizing workers in orchards to enable the safe operation of autonomous vehicles. Dvorak, Stone, and Self (2016) developed sensors to detect human presence from agricultural machinery, which is always a concern when humans work near machinery, as is common in specialty crop production. The topic of safety for autonomous machines is extremely important, as the lack of research and standards presents regulatory obstacles for the deployment of such equipment.
Physical robot-crop interaction, such as grasping, cutting, etc., is essential to many production operations, and developing optimized manipulation units is very important. Edan et al. (1992) developed a finite element model of a gripper. There have been many designs for grippers of fruit in general (Zhang et al., 2020b), and for soft grippers, in particular (Navas et al., 2021) but there is not wide acceptance of any particular design. Gripper design guidelines would greatly advance the development of harvesting equipment. In terms of robotic manipulators for specialty crops operations, Cho et al. (2002b) adapted three-degree-of-freedom robotic arms for cutting lettuce heads. Sivaraman and Burks (2006) developed performance indices for such manipulators, and Molaei et al. (2022) optimized arms for grapevine pruning. Clearly, more work needs to be done in the areas of manipulator and end-effector design and optimization.
Precision pesticide application has also been a topic of active research. Khot et al. (2012a, b) retrofitted an axial-fan airblast sprayer for use in citrus with adjustable air assistance and liquid flow rates. Spray patterns evaluation and resulting spray decision rules formulated to operate the retrofitted sprayer were effectively tested under field conditions in varied-sized citrus canopies. The results revealed that variable rate spray applications would result in 50% or less chemical usage while having comparable spray deposition to control. Several researchers (Oberti et al., 2016; Hezajipoor et al., 2021; Meshram et al., 2022) have implemented selective targeting of pesticide applications in orchards and vineyards for disease control.
Precision irrigation is an area of automation that has received much attention. Dwindling water supplies, prolonged droughts, and restrictive regulations on water abstraction are pushing growers to improve their water use efficiency (Monaghan et al., 2012). In this context, advancement in mapping, remote/proximal sensing and robotics automation technologies provide an opportunity for growers to reduce water usage without affecting yield or quality (Maes and Steppe, 2019); Abioye et al., 2020; Chandel et al., 2021). Precision irrigation, defined as site-specific irrigation management requires substantial investment in variable rate irrigation infrastructure, sensors, telemetry and in decision support tools to facilitate the management of such a complex system (Daccache et al., 2015). Despite the slow adoption of precision irrigation, the infrastructure for precision agriculture is building up in various countries. In California, labor shortage and the restrictive environmental constraints (i.e., SGMA) are pushing growers to embrace more precision irrigation technology. This explains the growing number of precision irrigation service providers in the private sector. However, the progress in this area depends on further collaborations between policy makers, research communities, private sector and the farmers. Using qualitative interviewing and analysis, Caplan et al. (2014) used the diffusion of innovations framework to gain insight into what channels of communications impacted planned adoption rates and what aspects of technology influenced the decision-making process. Interviews of participants emphasized the need to adopt new technologies while considering the capital investment of more complex technology, changes in labor management to integrate technology, the applicability of technology to current practices, and trust in technology providers.
A systems approach is needed to solve specialty crop labor-intensive and sensing challenges. A key theme in this project is investigating the whole system, ranging from identifying key biological parameters of specialty crops to commercializing new products. Our long-term goals are increased production efficiency, profitability, environmental stewardship, and social responsibility in specialty crop systems.
Objectives
-
Connect biological mechanisms and attributes relevant to specialty crop production, harvest, and postharvest handling with quantifiable parameters that can be sensed
-
Develop sensors and sensing systems that can measure and interpret biological parameters
-
Study interactions between machinery and crop to provide a basis for creating optimal mechanical and automated solutions for specialty crop production
-
Develop specialty crop architectures and production systems that are more amenable to mechanization
-
Develop, integrate and evaluate automated and autonomous systems with varying degrees of mechanization, sensing and AI to assist specialty crop industries with labor, management decisions, and reduction of production costs
-
Develop collaborations with equipment and technology manufacturers to commercialize and implement the outcomes of this project
Methods
Multiple Crop and Cross-Platform Integration: A key and continuing theme in this project is a multi-dimensional integration of research activities, integration from the adaptation of biological concepts to measurable parameters through the commercialization of new products, and integration within each objective among different specialty crops. Although the objectives seem sequential in execution, we anticipate a swirl of concurrent activities centered on and driven by the needs of specialty crop growers to automate growing, harvesting, and postharvest operations. A major task for the project leadership is to facilitate communication and collaboration among the members of this project and between project members and other stakeholders in specialty crop agriculture. Also, the participants will select early on a number of "typical" specialty crops, which will serve as “case-studies” for the integration of activities. Possible candidates include apple trees, grape vines and strawberries, crops that represent large segments of the specialty crop market, span large geographical areas and present different challenges to mechanization, automation, sensing and precision management.
Objective 1: Connect biological mechanisms and attributes relevant to specialty crop production, harvest, and postharvest handling with quantifiable parameters that can be sensed.
Participants: To, Zhang, Ghatrehsamani, Donis-Gonzalez, Khorsandi
Every automation system for specialty crops will depend on sensing both the immediate environment and the "biological state" (the combination of genetics, growth history including pesticide pressures, and current environmental conditions which influence future growth and quality factors) of the plant and produce. Developing the knowledge relating the biological state to parameters that can be externally measured is central to sensor design (Schueller, 2021).
Relating the biological state to measurable parameters is a direct continuation of the research conducted under W1009, W2009, and W3009, the predecessors of the proposed project. There is a deep level of expertise among the members of this proposed project in the areas of physical properties, enzymatic reactions, internal quality, and plant response to insect or disease attacks and environmental stress.
Defining how external loads are transferred to cells and under what conditions those loads will result in mechanical failure and/or injury response from the plant has been studied in almost every experiment station represented in this project. Continuing and future research will define the cellular mechanical properties and how these cell-scale properties integrate to form tissue-scale mechanical properties linked to quality parameters such as firmness and crispness.
Optical and electrical properties will be applied to assess internal and external quality factors, such as the amount of soluble sugar and the maturity level of many fruits and vegetables. Optical, NMR, X-ray, infrared, and other electromagnetic techniques will be applied to determine the relationship between internal quality factors and consumer acceptance of various produce using, with a focus on the development of rapid, cost-effective, and more accurate methods and techniques for quantification of the electromagnetic properties and their relationship with internal quality parameters of fruits and vegetables.
The biological concepts described above do not act in isolation from each other. Causal relationships such as enzyme activity and cell mechanical properties exist. Abnormal "biological states," such as water-deficient stress, often cause multiple responses among the concepts described above. Investigations will address using combinations of these concepts to gain a more precise and insightful understanding of the "biological state." For example, research into plant response to insect/disease attacks and environmental stress will provide a rapid and strong indicator that intervention is required.
Objective 2: Develop sensors and sensing systems that can measure and interpret biological parameters
Participants: Pourreza, Vougioukas, Lee, Dvorak, Lu, To, Zhang, Ghatrehsamani, Heinemann, He, Zahid, Ampatzidis, Pourreza, Gan, Donis-Gonzalez, Earles, Daccache, Khorsandi, Andrade-Sanchez, Jiang, Kadyampakeni
There is a long and rich history of sensor research and development aiming toward sensing the "biological state." New applications of established sensor types and novel sensors will be applied for phenotyping and assessing quality parameters. Most of the sensors developed to date are used in more typical controlled environments, so these sensors must be rugged and adaptable for field and packinghouse use. Three human senses can roughly classify the multitude of sensor designs; touch, sight, and smell.
Image and vision-based sensors will provide the bulk of the information to an automation system. Vision systems (including cameras, NMR devices, I.R. devices, and other devices based on radiated energy) will be focused on various targets, work non-invasively, provide information in visible and non-visible spectra, and provide a large amount of data. The challenge in using vision systems is to extract the required information from the collected data. Moving to a cost-effective, portable system in an outdoor environment will be challenging for some imaging devices, such as NMR and X-ray. New concepts, such as multi-view images, machine learning and an ever-increasing amount of portable computing power, will be applied to extract the information. Still, there is a significant challenge in designing effective vision-based sensing systems at an acceptable cost. New tools from deep learning neural networks will also be utilized to address this challenge.
The elevated cost of sensors needed to assess plant stress and map, monitor and control homogeneous management zones represents the main hurdle for mass adoption of precision irrigation. Therefore, this project will focus on i) developing low-cost sensing solutions (Abdelmoneim et al., 2021; Dhillon et al., 2017), ii) harness the potential of freely available satellite imagery (Mokhtari et al., 2021) and iii) explore the potential of machine learning in predicting physiological and climatic parameters with limited number of sensors (Ahmadi et al., 2022). This approach is based on the concept of Ghandian engineering where more (Sensors), for more (people) for less (money) is encouraged to promote mass adoption of precision irrigation.
Objective 3: Study interactions between machinery and crop to provide a basis for creating optimal mechanical and automated solutions for specialty crop production
Participants: Vougioukas, Dvorak, To, Zhang, Ghatrehsamani, Heinemann, He, Karkee, Earles, Fathallah, Andrade-Sanchez
Physical contact will involve many interactions between the crop and machinery (such as pruning, thinning, harvesting, sorting, storage, and transportation). Most of these interactions must be non-destructive to the plant. Sensing of mechanical properties to establish maximum forces that may be applied without damage will be investigated and determined. Research and design are required to develop sensors that can nondestructively, rapidly, and accurately determine the inherent strength of plant tissue.
Mechanical or automated harvesting of fruit crops is a critical issue in specialty crop production. Experiments will be carried out in mechanical impact and vibration energy transmission through canopies in different crop architectures. This will provide valuable information for developing efficient and effective interfaces for existing mechanical harvesters and new mechanical and automated harvesting technologies. The accessibility of machines to fruits, flowers, and branches in different types of fruit trees and bushes will be studied, which will be beneficial for improving or developing new technologies for harvesting and pruning, and thinning. Accessibility affects the efficiency of the above mentioned operations, and efficiency is a key factor for cost effectiveness.
Chemical application by fixed and mobile spraying systems that move in various types of crop canopies will be investigated to improve technologies for better coverage and reduced drift and off-site movement. Multi-disciplinary work to simultaneously improve machines and crop architectures will be the key to success in these areas.
In addition to 'traditional' methods based on field testing, model-based approaches will also be pursued. Tree canopies will be digitized using high-resolution scanners, fruit distributions will be assessed using computer vision and canopy-fruit-machine interactions (e.g., ease of canopy penetration, fruit reachability) will be investigated using software and computational geometry methods. The working speed of robots with many actuators/arms will be also investigated because it affects the cost-effectiveness of operations.
Objective 4: Develop specialty crop architectures and production systems that are more amenable to mechanization
Participants: Dvorak, Ghatrehsamani
For mechanization and automation to be successful, the crop architecture must enable machinery to easily access the plants. Conventional orchard layouts with large, wide (i.e., three-dimensional) trees make mechanized tasks very difficult. Research into novel architectures will continue, focusing on changing the structures to a more two-dimensional configuration, making many production tasks simpler (such as thinning, pruning, scouting, and harvesting). The orchard architecture developments will be addressed through commercially viable approaches. New and innovative technologies have been proven more successful with the change in structures. An example is mechanical blossom thinning using a string thinner. Proper training and pruning helped to optimize the thinning levels, resulting in reduced labor and higher quality yields. Crop architectures will continue to be investigated, and training systems will be further refined to achieve high productivity, low canopy training/management costs, and improved interaction with machinery. This objective focuses on integrating biology, horticulture, and production economics. The study of machine-canopy interaction constitutes an objective of its own.
Objective 5: Develop, integrate and evaluate automated and autonomous systems with varying degrees of mechanization, sensing, and A.I. to assist specialty crop industries with labor, management decisions, and reduction of production costs
Participants: Siemens, Li, Vougioukas, Lee, Dvorak, Lu, To, Zhang, Ghatrehsamani, Heinemann, He, Zahid, Karkee, Ampatzidis, Gan, Donis-Gonzalez, Earles, Daccache, Fathallah, Jiang, Kadyampakeni
Field operations' wide variation and semi-structured nature provide many research challenges. A key challenge is adaptability to multiple crops and cropping systems. Applications of new automation technologies and autonomous controls will be investigated to improve the efficacy of automated systems. These will be developed with cost constraints in mind, as growers can not necessarily afford high-cost solutions. The capital investment in automated equipment is high, and the potential market is small. It is critical to spread research and development costs by developing automated systems that can easily adapt to different crops and cropping systems.
An automated system for specialty crops is much more than an autonomous robot moving along a row of trees or plants. A cost-effective system will require information from many sources, both on-site and off-site, and autonomous robots or vehicles will require direction and coordination. Hence the efficient gathering of data and control of devices will be an integral part of an automated system. New autonomous orchard vehicles have been extensively tested and proven in the field. Further work can investigate the integration of tasks with these vehicles and platforms and fully automate certain operations that have not yet been successfully addressed, such as autonomous fresh fruit harvesting, precision thinning, pruning and pollination.
It is not feasible to replace human labor with automation in all operations. In some operations, the cost of replacing human dexterity and complex decision-making capability with equipment is not justified. A semi-automated device assisting human labor in these operations may be a better, more cost-effective solution. Developing a man-machine system requires careful attention to the ergonomic (safety, productivity, comfort, and intellectual engagement) needs of the human in the system. Very little research has been done on the ergonomic design of specialty crop equipment, and additional research is required to design an optimum man-machine system for specialty crops.
Furthermore, although there has been extensive research and development of farm robotics, very little specifically addresses the safety of farmworkers working with robots. Thus, research must be undertaken to establish safety and health standards and best practices for incorporating robots into farms. Such research needs to do the following: monitor trends in worker injuries; identify risk factors for farmworkers' injuries when working with robots; research interactions between humans and robots and optimal designs for the human-robot interface; and develop and evaluate interventions to prevent farmworkers' injuries.
Decisions regarding an automated system's overall plan and control will remain with a human operator. The quantity of operator decision-making information needed for various equipment's autonomous or semi-autonomous operations may be unprecedented and unmanageable. There is a need to develop computer-based data systems that collect, verify, and organize raw data to present information to the operator, such as crop maturity, crop stress, and spatial variation. There is also a need to organize predictive models for crop needs such as pesticide application, pruning, thinning, and harvesting. Finally, there is also a need to help the operator visualize the most effective use of the automated equipment.
Objective 6: Develop collaborations with equipment and technology manufacturers to commercialize and implement the outcomes of this project
Participants: Siemens, Vougioukas, Dvorak, To, Ghatrehsamani, He, Karkee, Ampatzidis, Donis-Gonzalez, Earles, Jiang, Kadyampakeni
The selection of a particular crop and operation covered by this project will be based on growers' needs to automate that crop and operation. It is important to take an integrated approach and for researchers working on different aspects of specialty crop production to come together and collaborate to develop real-world solutions. The research areas may include horticulture, agricultural and biological engineering, plant and soil science, plant pathology, food quality and safety, postharvest biology and technologies, water resources, agricultural economics, environmental science, and mechanical engineering, and not limited to those mentioned above. With that as a premise, we cannot consider the research complete until a piece of equipment or device is commercialized and in use. Immediately following acceptance of this project, we will initiate meetings between project participants, specialty crop growers, and manufacturers to target specific crops and operations for which we will develop automation.
Commercializing many critical automation needs of specialty crop growers will be difficult to justify using traditional business plans. The main reason is that the small size of the market, coupled with potentially high development costs, results in a low return on investment (ROI). Manufacturers will need help to reduce the cost of development and risk assumed to market a new product. Frequent communication between growers, manufacturers, and project participants will help to mitigate costs and risks to the manufacturers. We will create opportunities for our partnerships with specialty crop growers and manufacturers (currently, companies like Deere & Company, Oxbo International Corp., Durand-Wayland, Inc., Trimble Navigation Ltd., DBR Conveyance Concepts, COE Orchard Eq., Orchard Machinery Corporation, Atlas Pacific Engineering, Future Acres, FF Robotics and others have partnered with project participants in various projects and/or programs).
Defining industry standards for the test, operation, and components of automated equipment is necessary. Standards will enable the interchangeability of parts and software, decrease design time, and encourage manufacturers to build parts such as articulating arms and end effectors. We will work with the standards committees of ASABE and SAE to propose standards related to automation in specialty crops.
The design of automation systems is a continually evolving engineering area, and the application of automation design to specialty crops is very new. Scientific findings and engineering concepts and techniques learned during this project should be shared among practicing scientists, engineers, and students. During the project, we will periodically collect concepts and techniques learned from among the participants and disseminate this knowledge through classroom and continuing education venues.
Measurement of Progress and Results
Outputs
- Production structures and systems that fit mechanization and automation
- Sensors capable of measuring the "biological state" adapted for outdoor use on automated equipment
- Sensors used in industrial and military automation adapted for use in specialty crop environments
- Sensors can measure and monitor product quality and food safety during harvest and postharvest operations.
- Specialty crop automated and semi-automated equipment available
- Wide-area specialty crop data communication systems available
- Decision-making software for use in aiding the management of automated equipment
- Engineering models relating mechanical and/or physical properties, enzymatic reactions, internal quality, and plant external stress indicators to the "biological state" of selected specialty crops
- Engineering models which estimate "biological state" based on the interaction of multiple indicators
- Integrated set of design, test, and manufacturing standards
- Design, manufacture, and use education modules for university and continuing education learning.
Outcomes or Projected Impacts
- Specialty crop technology development
- Modern, mechanization-compatible crop production designs
- Research publications in the design of specialty crop technologies
- Training of graduate and undergraduate students in the design and concepts of specialty crop automated equipment
- Knowledge dissemination through workshops and other continuing education opportunities for practicing scientists and engineers
- Competitive advantage for domestic specialty crop producers by increasing labor efficiency with automated equipment and systems
- Healthier and safer working environment for the workforce in specialty crop production and handling
- Manufacturing and agriculture workforce (design engineers, mechanics, operators, and technicians) better prepared to manufacture, use, and maintain automated equipment for specialty crop production, postharvest storage, processing, handling, and sorting
- Reduction of the impact of specialty crop production on the environment through more precise field, postharvest facilities, and packinghouse operations
- Enhanced safety, quality, and shelf life of fruits and vegetables for fresh market via interdisciplinary innovations from field management to postharvest handling
- Resilient and diverse specialty crop production systems for a strengthened supply chain of fruits and vegetables across the U.S.
Milestones
(1):Year 1: Identify emerging technologies in specialty crops and forecast future research needs. (2024): Spring 2024: Obtaining industrial and federal grants to support team research(2):Year 2: Organize systems-driven research and extension teams on each focus area and develop technology elements (2025): Spring 2025: Developing prototypes and conducting field research
(3):Year 3: Create testbeds to transfer new technologies developed by the teams (2026): Spring 2026: Filing patent application for developed technologies
(4):Year 4: Develop strong commercialization plans and support industry in adopting developed technologies (2027): Spring 2027: Organizing workshops and helping industry to commercialize and apply the technologies.
(5):Fall 2028: Project ends (renewal in place)
(0):We list the above set of milestones that we expect to achieve collectively. The activities included in the milestones are already taking place in different institutions, albeit not necessarily in a synergistic or coordinated fashion. Each individual station may achieve some of these milestones earlier or later than the anticipated year, but the activities are being performed. Our goal is to increase the number and the synergy of joint activities among collaborating partners. Hence, the milestones serve as targets for coordination, and therefore we see no need to identify backup plans in case the milestones are not met.
Projected Participation
View Appendix E: ParticipationOutreach Plan
From the user-centered design nature of this project, outreach to our partners is continuous and integral to the project. The intended users will be a part of the initial meetings to select targeted crops/operations, in the prototype design and testing of equipment, in developing a business plan and marketing tools, and in the commercialization of the target equipment. Indicators of the project outreach will include the expected scientific papers, patents, and publications in the trade and popular press. In addition, there will be frequent communication with manufacturers, growers, and the people who will be using the automated equipment, sets of standards to convey the design concepts learned to a wide audience of engineers and technicians, and educational modules which can be used in the classroom and continuing education venues.
Organization/Governance
The technical committee will consist of project leaders for the contributing states, the administrative advisor, and CSREES representatives. Voting membership includes all persons with contributing projects.
A Chairperson, Vice Chairperson, and Secretary will be elected from the voting membership at the first authorized committee meeting after the project has been approved. The Chair, Vice-Chair, and Secretary will serve two years if so desired by the membership. They will be responsible for meeting arrangements, annual reports, implementation of the Outreach Plan, and preparation of the renewal proposal.
Due to the number and diversity of the membership and the user-based nature of this project, a working group and coordinator for each target crop/operation will be selected by the Chairperson from among the members. The coordinator will be responsible for communication within the working group, developing a timeline for the targeted crop/operation, and coordinating activities among the four project objectives (as applied to the particular targeted crop/operation).
Literature Cited
Abdelmoneim, A., Daccache, A., Khadra, R., Bhanot, M., Dragonetti, G. (2021). Internet of Things (IoT) for doublering infiltrometer automation. Computers and Electronics in Agriculture, Volume 188, September 2021, 106324
Abioye, E. A., Abidin, M. S. Z., Mahmud, M. S. A., Buyamin, S., Ishak, M. H. I., Abd Rahman, M. K. I., ... & Ramli, M. S. A. (2020). A review on monitoring and advanced control strategies for precision irrigation. Computers and Electronics in Agriculture, 173, 105441.
Ahmadi A., Daccache A., Snaryder R., Suvočarev K. (2022). Meteorological driving forces of reference evapotranspiration and their trends in California, Science of The Total Environment, 157823.
Allison, J. M., JR. Prussia, S. E. Daniell, J. W. & Tollner, E. W. (1987). Measuring the internal pressure of maturing pecans. In Transactions of the ASAE - American Society of Agricultural Engineers pp. 1869-1872.
Arikapudi, R., Vougioukas, S.G., Jiménez- Jiménez, F., Farangis Khosro Anjom, F. (2016). Estimation of Fruit Locations in Orchard Tree Canopies Using Radio Signal Ranging and Trilateration. Computers and Electronics in Agriculture (125):160-172. http://dx.doi.org/10.1016/j.compag.2016.05.004
Bac, C. W., Van Henten, E. J., Hemming, J., & Edan, Y. (2014). Harvesting robots for high‐value crops: State‐of‐the‐art review and challenges ahead. Journal of Field Robotics, 31(6), 888-911.
Bao, Y., L. Tang. 2016. Field-based Robotic Phenotyping for Sorghum Biomass Yield Component Traits Characterization Using Stereo Vision. IFAC-PapersOnLine. 49(16):265-270. https://doi.org/10.1016/j.ifacol.2016.10.049
Baugher, T. Auxt. 2003. Training Systems. In: Baugher, T. Auxt and S. Singha (editors). 2003. Concise Encyclopedia of Temperate Tree Fruit. Haworth Press, Inc., NY. 387 pp.
Bergerman, M., S. Singh, and B. Hamner. 2012. Results with autonomous vehicles operating in specialty crops. IEEE International Conference on Robotics and Automation. Saint Paul, Minnesota. May 14-15. Pp. 1829-1835.
Bloch, V., Degani, A., Bechar, A. 2018. A methodology of orchard architecture design for an optimal harvesting robot, Biosystems Engineering (166):126-137.
Byers, R. E. Hogmire, H. W. Ferree, D. C. Hall, F. R. & Donahue, S. J. (1989). Spray chemical deposits in high-density and trellis apple orchards. In HortScience pp. 918-920.
Caplan, S., B. Tilt, G. Hoheisel, T. Auxt Baugher. 2014. Specialty crop growers' perspectives on adopting new technologies. HortTechnology 24:81-87.
Castillo-Ruiz, F. J., Sola-Guirado, R. R., Castro-Garcia, S., Gonzalez-Sanchez, E. J., Colmenero-Martinez, J. T. & Blanco-Roldan, G. L. 2017. Pruning systems to adapt traditional olive orchards to new integral harvesters. Scientia Horticulturae, 220: 122-129.
Cavalieri R. P. Hyde, G. M. & Macquirre, P. R. (1998). Hydraulic sorting of watercore apples. In Acta horticulturae pp. 103-108
Cellini, A., Blasioli, S., Biondi, E., Bertaccini, A., Braschi, I., & Spinelli, F. (2017). Potential Applications and Limitations of Electronic Nose Devices for Plant Disease Diagnosis. Sensors, 17(11). https://doi.org/10.3390/s17112596 Chandel, A. K., Khot, L. R., Molaei, B., Peters, R. T., Stöckle, C. O., & Jacoby, P. W. (2021). High-resolution spatiotemporal water use mapping of surface and direct-root-zone drip-irrigated grapevines using uas-based thermal and multispectral remote sensing. Remote Sensing, 13(5), 954.
Chen, S. W., Shivakumar, S. S., Dcunha, S., Das, J., Okon, E., Qu, C., Taylor, C. J. & Kumar, V. 2017. Counting Apples and Oranges with Deep Learning: A Data-Driven Approach. Ieee Robotics and Automation Letters, 2(2): 781-788.
Cho, S. I. & Krutz, G. W. (1989). Fruit ripeness detection by using 1H-NMR. In Paper - American Society of Agricultural Engineers p. 11 p.
Cho, Y. J. You, B. J. Lim, J. H. & Oh, S. R. (2000). Real-time visual tracking insensitive to three-dimensional rotation of objects. In Biologically Motivated Computer Vision, Proceeding pp. 160-167.
Cho, S. I. Chang, S. J. Kim, Y. Y. & An, K. J. (2002). Development of a three-degrees-of-freedom robot for harvesting lettuce using machine vision and fuzzy logic control. In Biosystems engineering pp. 143-149.
Choi, D., Lee, W. S., Schueller, J. K., Ehsani, R., Roka, F., & Diamond, J. 2017. A performance comparison of RGB, NIR, and depth images in immature citrus detection using deep learning algorithms for yield prediction. 2017 ASABE Annual International Meeting, Paper No. 1700076, pages 1-6, July 16 – 19, 2017.
Choi, D., Lee, W. S., Schueller, J. K., Ehsani, R., Roka, F., & Ritenour, M. 2016. A precise fruit inspection system for Huanglongbing and other common citrus defects using GPU and deep learning technologies. 13th International Conference on Precision Agriculture, Paper No. 1835, pages 1-6, July 31 - August 4, 2016.
Constante, P., Gordon, A., Chang, O., Pruna, E., Escobar, I. & Acuna, F. 2016. Artificial Vision Techniques for Strawberry's Industrial Classification. IEEE Latin America Transactions, 14(6): 2576-2581.
Cortes, V., Blanes, C., Blasco, J., Ortiz, C., Aleixos, N., Mellado, M., Cubero, S. & Talens, P. 2017. Integration of simultaneous tactile sensing and visible and near-infrared reflectance spectroscopy in a robot gripper for mango quality assessment. Biosystems Engineering, 162: 112-123.
Cooper, M. R. Barton, G. T. & Brodell, A. P. (1947). Progress of farm mechanization. p. 101. Washington, D.C.: U.S. Dept. of Agriculture.
Crassweller, R.M. and D.E. Smith. 2013. Second generation apple systems trials. P.A. Fruit News 93 (1):67-71.
Cubero, S., Aleixos, N., Moltó, E., Gómez-Sanchis, J., Blasco, J., 2010. Advances in Machine Vision Applications for Automatic Inspection and Quality Evaluation of Fruits and Vegetables. Food and Bioprocess Technology 4:487-504.
Daccache, A, Knox, J.W., Weatherhead, E.K., Daneshkhah, A., and Hess, T. (2015). Implementing precision irrigation in a humid climate - recent experiences and ongoing challenges. Agricultural Water management. Volume 147 Pages 135–143
Delwiche, M. J. (1987). Grader performance using a peach ground color maturity chart. In HortScience pp. 87-89.
Delwiche, M. J. Arevalo, H. & Mehlschau, J. (1996). Second generation impact force response fruit firmness sorter. In Transactions of the ASAE pp. 1025-1033.
Delwiche, M. J. & Baumgardner, R. A. (1986). Conversion between southeastern and California peach sizes. In HortScience pp. 1238-1239.
Delwiche, M. J. Mcdonald, T. & Bowers, S. V. (1987). Determination of peach firmness by analysis of impact forces. In Transactions of the ASAE - American Society of Agricultural Engineers pp. 249-254.
Delwiche, M. J. Tang, S. & Rumsey, J. W. (1987). Color and optical properties of Clingstone peaches related to maturity. In Transactions of the ASAE - American Society of Agricultural Engineers pp. 1873-1879.Dhillon, R. S., Upadhaya, S. K., Rojo, F., Roach, J., Coates, R. W., & Delwiche, M. J. (2017). Development of a Continuous Leaf Monitoring System to Predict Plant Water Status. Transactions of the ASABE, 60(5), 1445-1455.
Donis-González, I.R., Guyer, D.E., Pease, A., 2012a. Application of Response Surface Methodology to systematically optimize image quality in computer tomography: A case study using fresh chestnuts (Castanea spp.). Computers and Electronics in Agriculture 87:94-107.
Donis-González, I.R., Guyer, D.E., Pease, A., Fulbright, D.W., 2012b. Relation of computerized tomography Hounsfield unit measurements and internal components of fresh chestnuts (Castanea spp.). Postharvest Biology and Technology 64:74-82.
Donis-González, I.R., Guyer, D.E., Fulbright, D.W., Pease, A., 2014. Postharvest noninvasive assessment of fresh chestnut (Castanea spp.) internal decay using computer tomography images. Postharvest Biology and Technology 94: 14-25.
Donis-González, I.R., Guyer, D.E., Chen, R., Pease, A., 2015. Evaluation of undesirable fibrous tissue in processing carrots using Computed Tomography (C.T.) and structural fiber biochemistry. Journal of Food Engineering 153:108-116.
Dvorak, J., Stone, M., & Self, K. (2016) Object Detection for Agricultural and Construction Environments using an Ultrasonic Sensor. Journal of Agricultural Safety and Health. 22(2): 107-119. doi: 10.13031/jash.22.11260.
Durand-Petiteville, A., Vougioukas, S. G., Slaughter, D.C. (2017). Real-time segmentation of strawberry flesh and calyx from images of singulated strawberries during postharvest processing. Computers and Electronics in Agriculture, (142A): 298-313. https://doi.org/10.1016/j.compag.2017.09.011
Edan, Y. Haghighi, K. Stroshine, R. & Cardenas-Weber, M. (1992). Robot gripper analysis: finite element modeling and optimization. In Applied engineering in agriculture pp. 563-570.
Espinoza, C. Z., Khot, L. R., Sankaran, S. & Jacoby, P. W. 2017. High Resolution Multispectral and Thermal Remote Sensing-Based Water Stress Assessment in Subsurface Irrigated Grapevines. Remote Sensing, 9(9): 961, pp 1-15.
Everard, C., M. Kim, M.C. Siemens, H. Cho, H., A. LeCourt, and C. O'Donnell. 2017. A multispectral imaging system using solar illumination to distinguish fecal matter on leafy greens and soils. Biosystems Eng. (submitted)
Fan, S. X., Li, C. Y., Huang, W. Q. & Chen, L. P. 2017. Detection of blueberry internal bruising over time using NIR hyperspectral reflectance imaging with optimum wavelengths. Postharvest Biology and Technology, 134:55-66.
Fei, Z., Vougioukas, S.G. (2021). Co-Robotic Harvest-aid Platforms: Real-time Control of Picker Lift Heights to Maximize Harvesting Efficiency. Computers and Electronics in Agriculture. (180): 105894. https://doi.org/10.1016/j.compag.2020.105894
Fei, Z., Vougioukas, S.G. (2022). Row-sensing Templates: A Generic 3D Sensor-based Approach to Robot Localization with Respect to Orchard Row Centerlines. Journal of Field Robotics, 39(6):712-738. https://doi.org/10.1002/rob.22072
Fennimore, S.A., B. D. Hanson, L. M. Sosnoskie, J. B. Samtani, A. Datta, S. Z. Knezevic, and M. C. Siemens. 2013. Chapter 9: Field Applications of Automated Weed Control: Western Hemisphere. In Automation: The Future of Weed Control in Cropping Systems, 151-169. S.L. Young and F.J. Pierce, eds. Dordrecht: Springer Science+Business Media.
Fennimore, S.F., D.C. Slaughter, M.C. Siemens, R.G. Leon, and M.N. Saber. 2016. Technology for Automation of Weed Control in Specialty Crops. Weed Technology, 30(4): 823-837.
Fennimore, S.A. & Siemens, M.C. 2022. Mechanized weed management in vegetable crops. In Encyclopedia of Smart Agricultural Technologies, ed. Qin Zhang. (accepted)
Fisher, J.B., 1992. How predictive are computer simulations of tree architecture? International Journal Plant Science, 153(3): 137-146
Gan, H., Lee, W. S., & Alchanatis, V. (2017). A Prototype of an Immature Citrus Fruit Yield Mapping System. In 2017 ASABE Annual International Meeting (p. 1). American Society of Agricultural and Biological Engineers.
Gao, F. F., Fang, W. T., Sun, X. M., Wu, Z. C., Zhao, G. A., Li, G., Li, R., Fu, L. S., & Zhang, Q. (2022). A novel apple fruit detection and counting methodology based on deep learning and trunk tracking in modern orchard. Computers and Electronics in Agriculture, 197. https://doi.org/10.1016/j.compag.2022.107000
Giles, D. K. Delwiche, M. J. & Dodd, R. B. (1987). Control of orchard spraying based on electronic sensing of target characteristics. In Transactions of the ASAE - American Society of Agricultural Engineers pp. 1624-1630, 1636.
Gongal, A., Silwal, A., Amatya, S., Karkee, M., Zhang, Q., & Lewis, K. (2016). Apple crop-load estimation with over-the-row machine vision system. Computers and Electronics in Agriculture, 120, 26-35.
Grise, V. N. & Johnson, S. S. (1973). An economic analysis of cling peach production with emphasis on harvest mechanization. pp. vi, 38. Washington, D.C.: U.S. Dept. of Agriculture.
Gunderman, A., Collins, J., Myers, A., Threlfall, R., & Chen, Y. (2022). Tendon-Driven Soft Robotic Gripper for Blackberry Harvesting. Ieee Robotics and Automation Letters, 7(2), 2652-2659. https://doi.org/10.1109/Lra.2022.3143891
Guo, W., Carroll, M. E., Singh, A., Swetnam, T. L., Merchant, N., Sarkar, S., Singh, A. K., & Ganapathysubramanian, B. (2021). UAS-Based Plant Phenotyping for Research and Breeding Applications. Plant Phenomics, 2021. https://doi.org/10.34133/2021/9840192
Guyer, D. E. Schulte, N. L. Timm, E. J. & Brown, G. K. (1991). Minimizing apple bruising in the packingline. In Tree fruit postharvest journal pp. 14-20
He, L., Fu, H., Sun, D., Karkee, M. & Zhang, Q. 2017. Shake-and-Catch Harvesting for Fresh Market Apples in Trellis-Trained Trees. Transactions of the ASABE, 60(2): 353-360.
Heinemann, P. H. Hughes, R. Morrow, C. T. Sommer, H. J., III Beelman, R. B. & Wuest, P. J. (1994). Grading of mushrooms using a machine vision system. Transactions of the ASAE 37(5), 1671-1677.
Hejazipoor, H., Massah, J., Soryani, M., Vakilian, K. A., & Chegini, G. (2021). An intelligent spraying robot based on plant bulk volume. Computers and Electronics in Agriculture, 180, 105859.
Hohimer, C. J., Wang, H., Bhusal, S., Miller, J., Mo, C., & Karkee, M. (2019). Design and field evaluation of a robotic apple harvesting system with a 3D-printed soft-robotic end-effector. Transactions of the ASABE, 62(2), 405-414.
Huang, Y., Chen, Z. X., Tao, Y. U., Huang, X. Z., & Gu, X. F. (2018). Agricultural remote sensing big data: Management and applications. Journal of Integrative Agriculture, 17(9), 1915-1931.
Hung, C., Underwood, J., Nieto, J., & Sukkarieh, S. (2015). A feature learning based approach for automated fruit yield estimation. In Field and Service Robotics (pp. 485-498). Springer International Publishing.
Ikediala, J. N. Tang, J. Drake, S. R. & Neven, L. G. (2000). Dielectric properties of apple cultivars and codling moth larvae. In Transactions of the ASAE pp. 1175-1184.
Jiang, Y., & Li, C. Y. (2020). Convolutional Neural Networks for Image-Based High-Throughput Plant Phenotyping: A Review. Plant Phenomics, 2020. https://doi.org/10.34133/2020/4152816
Joshi, A., Guevara, D., & Earles, M. (2022). Standardizing and Centralizing Datasets to Enable Efficient Training of Agricultural Deep Learning Models. arXiv preprint arXiv:2208.02707.
Khosro Anjom, F., Vougioukas, S. G., Slaughter, D.C. (2018). Development of a Linear Mixed Model to Predict the Picking Time in Strawberry Harvesting Processes. Biosystems Engineering. (166): 76-89. https://doi.org/10.1016/j.biosystemseng.2017.10.006
Khot, L. R., Ehsani, R., Albrigo, G., Larbi, P. A., Landers, A., Campoy, J., & Wellington, C. (2012a). Air-assisted
sprayer adapted for precision horticulture: Spray patterns and deposition assessments in small-sized citrus canopies. Biosystems Engineering, 113, pp. 76-85.
Khot, L. R., Ehsani, R., Albrigo, G., Larbi, P. A., & Landers, A., (2012b). Spray pattern investigation of an axial-fan airblast precision sprayer using a modified vertical patternator. Applied Engineering in Agriculture, 28(5), pp. 647-654.
Kon, T. M., J. R. Schupp, H. E. Winzeler and R. P. Marini. 2013. Influence of mechanical string thinning treatments on vegetative and reproductive tissues, fruit set, yield and fruit quality of apple. HortScience 48:40-46.
Kootstra, G., Wang, X., Blok, P. M., Hemming, J., & Van Henten, E. (2021). Selective harvesting robotics: current research, trends, and future directions. Current Robotics Reports, 2(1), 95-104.
Larbi, P. A., Karkee, M., Amatya, S., Zhang, Q. & Whiting, M. D. 2015. Modification and field evaluation of an experimental mechanical sweet cherry harvester. Applied Engineering in Agriculture, 31(3): 387-397.
Lefcourt, A.M. and M.C. Siemens. 2017. Interactions of insolation and shading on ability to use fluorescence imaging to detect fecal contaminated spinach. Appl. Sci., 7, 1041.
Li, J. B., Chen, L. P. & Huang, W. Q. 2018. Detection of early bruises on peaches (Amygdalus persica L.) using hyperspectral imaging coupled with improved watershed segmentation algorithm. Postharvest Biology and Technology, 135: 104-113.
Li, J., L. Tang. 2017. Crop recognition under weedy conditions based on 3D imaging for robotic weed control. Journal of Field Robotics. 2017:1-16
Li, J., L. Tang. 2017. Developing a low-cost 3D plant morphological traits characterization system. Computers and Electronics in Agriculture 143:1-13.
Li, D. L., Quan, C. Q., Song, Z. Y., Li, X., Yu, G. H., Li, C., & Muhammad, A. (2021). High-Throughput Plant Phenotyping Platform (HT3P) as a Novel Tool for Estimating Agronomic Traits From the Lab to the Field. Frontiers in Bioengineering and Biotechnology, 8. https://doi.org/10.3389/fbioe.2020.623705
Li, L., Zhang, Q., & Huang, D. F. (2014). A Review of Imaging Techniques for Plant Phenotyping. Sensors, 14(11), 20078-20111. https://doi.org/10.3390/s141120078
Liu, E., Gold, K., Cadle-Davidson, L., Combs, D., & Jiang, Y. (2022, 23-27 Oct. 2022). Near Real-Time Vineyard Downy Mildew Detection and Severity Estimation. 2022 IEEE/RSJ International Conference on Intelligent Robots and Systems (IROS),
Liu, E., Gold, K. M., Combs, D., Cadle-Davidson, L., & Jiang, Y. (2022). Deep semantic segmentation for the quantification of grape foliar diseases in the vineyard. Frontiers in Plant Science, 13. https://doi.org/10.3389/fpls.2022.978761
Lu, R. & Abbott, J. A. (1997). Finite element modeling of transient responses of apples to impulse excitation. In Transactions of the ASAE pp. 1395-1406.
Lu, R. & Abbott, J. A. (1996). Finite element analysis of models of vibration in apples. In Journal of texture studies pp. 265-286.
Lu, R. Guyer, D. E. & Beaudry, R. M. (2000). Determination of firmness and sugar content of apples using near- infrared diffuse reflectance. In Journal of texture studies pp. 615-630.
Lu, H., L. Tang, S.A. Whitham, Y. Mei. (2017). A Robotic Platform for Corn Seedling Morphological Traits Characterization. Sensors. 17(9), 2082.
Lyons, D.J., P.H. Heinemann, J. Liu, J., J.R. Schupp, and T.A. Baugher. 2015. Development of a selective automated blossom thinning system for peaches. Transactions of ASABE. 58(6):1447-1457.)
Maes, W. H., & Steppe, K. (2019). Perspectives for remote sensing with unmanned aerial vehicles in precision agriculture. Trends in plant science, 24(2), 152-164.
Marrazzo, W. N. Heinemann, P. H. Crassweller, R. E. & Leblanc, E. 2007. Electronic nose chemical sensor feasibility study for the differentiation of apple cultivars. Transactions of the ASAE 48(5), 1995-2002.
Meshram, A. T., Vanalkar, A. V., Kalambe, K. B., & Badar, A. M. (2022). Pesticide spraying robot for precision agriculture: A categorical literature review and future trends. Journal of Field Robotics, 39(2), 153-171.
Miller, B. K. & Delwiche, M. J. (1989). Automatic grading of fresh peaches using color computer vision. In Acta horticulturae pp. 161-166.
Miller, B. K. & Delwiche, M. J. (1991). Peach defect detection with machine vision. In Transactions of the ASAE pp. 2588-2597.
Miller, S., J. Schupp, T. Baugher, and S. Wolford. 2011. Performance of mechanical thinners for bloom or green fruit thinning in peaches. Hort-Science 46:43–51.
Mirbod, O., Choi, D., Heinemann, P. H., Marini, R. P., & He, L. (2023). On-tree apple fruit size estimation using stereo vision with deep learning-based occlusion handling. Biosystems Engineering, 226, 27-42. https://doi.org/https://doi.org/10.1016/j.biosystemseng.2022.12.008
Mokhtari, A.; Ahmadi, A.; Daccache, A.; Drechsler, K. (2021). Actual Evapotranspiration from UAV Images: A Multi-Sensor Data Fusion Approach. Remote Sens. 2021, 13, 2315.
Molaei, F., & Ghatrehsamani, S. (2022). Kinematic-Based Multi-Objective Design Optimization of a Grapevine Pruning Robotic Manipulator. AgriEngineering, 4(3), 606-625.
Monaghan J., Daccache A., Vickers L., Hess T., Grove Iand Knox J. (2012). More' crop per drop' – constraints and opportunities for precision irrigation in European agriculture. Journal of the science of food and agriculture. Volume 93, Issue 5: 977–980.
Munera, S., Amigo, J. M., Blasco, J., Cubero, S., Talens, P. & Aleixos, N. 2017. Ripeness monitoring of two cultivars of nectarine using VIS-NIR hyperspectral reflectance imaging. Journal of Food Engineering, 214: 29-39.
National Rural Center. (1980). Production efficiency and technology for small farms. p. 160 in various pagings. Washington, D.C. (1828 L Street, NW, Washington, D.C. 20036): The National Rural Center
Navarro, E., Costa, N., & Pereira, A. (2020). A Systematic Review of IoT Solutions for Smart Farming. Sensors, 20(15). https://doi.org/10.3390/s20154231
Navas, E., Fernández, R., Sepúlveda, D., Armada, M., & Gonzalez-de-Santos, P. (2021). Soft grippers for automatic crop harvesting: A review. Sensors, 21(8), 2689.
Nellithimaru, A. K., & Kantor, G. A. (2019). ROLS : Robust Object-level SLAM for grape counting. 2019 Ieee/Cvf Conference on Computer Vision and Pattern Recognition Workshops (Cvprw 2019), 2648-2656. https://doi.org/10.1109/Cvprw.2019.00321
Nguyen, T. T., Slaughter, D. C., Max, N., Maloof, J. N., & Sinha, N. (2015). Structured light-based 3D reconstruction system for plants. Sensors, 15(8), 18587-18612.
Nguyen, T. T., Slaughter, D. C., Townsley, B., Carriedo, L., Julin, N. N., & Sinha, N. (2016). Comparison of Structure-from-Motion and Stereo Vision Techniques for Full In-Field 3D Reconstruction and Phenotyping of Plants: An Investigation in Sunflower. In 2016 ASABE Annual International Meeting (p. 1).
Oberti, R., Marchi, M., Tirelli, P., Calcante, A., Iriti, M., Tona, E., Hocevar, M., Baur, J., Pfaff, J., Schutz, C. & Ulbrich, H. (2016). Selective spraying of grapevines for disease control using a modular agricultural robot. Biosystems Engineering, 146: 203-215.
Olenskyj, A. G., Sams, B. S., Fei, Z., Singh, V., Raja, P. V., Bornhorst, G. M., & Earles, J. M. (2022). End-to-end deep learning for directly estimating grape yield from ground-based imagery. Computers and Electronics in Agriculture, 198, 107081.
Pearson, T. C. Slaughter, D. C. & Studer, H. E. (1996). Hull adhesion characteristics of early-split and normal pistachio nuts. In Applied engineering in agriculture pp. 219-221.
Pellerin, R. A. Millier, W. F. Lakso, A. N. Rehkugler, G. E. Throop, J. A. & Allport, T. E. (1978). Apple Harvesting with an Inertial Vs. Impulse Trunk Shaker on Open-Center and Central-Leader Trees. I. In Trans Asae (Am Soc Agric Eng) pp. 407-413
Peng, C., Vougioukas, S., Slaughter, D., Fei, Z., Arikapudi, R. (2022) A strawberry harvest-aiding system with crop-transport co-robots: Design, development, and field evaluation. Journal of Field Robotics 39(8):1231-1257. https://doi.org/10.1002/rob.22106
Radcliffe, J., Cox, J., & Bulanon, D. M. (2018). Machine vision for orchard navigation. Computers in Industry, 98, 165-171.
Raja, R., Slaughter, D.C., Fennimore, S.A., Nguyen, T.T., Vuong, V.L., Sinha, N., Tourte, L., Smith, R.F. & Siemens, M.C. 2019. Crop signaling: A novel crop recognition technique for robotic weed control. Biosystems Eng. 187: 278-291.
Ran N. Lati, M.C. Siemens, J.S. Rachuy, and S.A. Fennimore. (2016). Intrarow Weed Removal in Broccoli and Transplanted Lettuce with an Intelligent Cultivator. Weed Technology, 30(3): 655-663.
Ray, J. A. Stroshine, R. L. Krutz, G. W. & Wai, W. K. (1993). Quality sorting of sweet cherries using magnetic resonance. In Paper p. 11.
Rehkugler, G. E. Millier W. F. Pellerin, R. A. & Throop, J. A. (1976). Analysis of a Reciprocating Panel Fruit Lowering Device. In Trans Asae (Am Soc Agric Eng) pp. 30-34.
Rehkugler, G. E. Stiefvater, T. L. & Throop, J. A. (1971). An Optical Bruise Detection Technique. [Mechanical Harvest,Apples]. In Amer Soc Agr Eng Trans Asae pp. 1189-1194.
Reyes, M. U. Paull, R. E. Williamson, M. R. & Gautz, L. D. (1996). Ripeness determination of "Solo" papaya (Carica papaya L.) by impact force. In Applied engineering in agriculture pp. 703-708.
Reynoldson, L. A. (1933). Utilization and cost of power on corn belt farms. p. 60. Washington: U.S. Dept. of Agriculture
Roberts, L., & Wickings, K. (2022). Biotremology: tapping into the world of substrate-borne waves. Acoust. Today, 18, 49-57.
Robinson, T. L. Millier, W. F. Throop, J. A. Carpenter, S. G. & Lakso, A. N. (1990). Mechanical harvestability of
Y-shaped and pyramid-shaped 'Empire' and 'Delicious' apple trees. In Journal of the American Society for Horticultural Science pp. 368-374.
Romero-Trigueros, C., Nortes, P. A., Alarcon, J. J., Hunink, J. E., Parra, M., Contreras, S., Droogers, P. & Nicolas, E. 2017. Effects of saline reclaimed waters and deficit irrigation on Citrus physiology assessed by UAV remote sensing. Agricultural Water Management, 183: 60-69.
Rounsaville, J., Dvorak, J., Stombaugh, T. (2016). Methods for Calculating Relative Cross-Track Error for ASABE/ISO 12188-2 from Discrete Measurements. Transactions of the ASABE. 59(6): 1609-1616. doi: 10.13031/trans.59.11902
Sa, I., Ge, Z. Y., Dayoub, F., Upcroft, B., Perez, T. & McCool, C. 2016. DeepFruits: A Fruit Detection System Using Deep Neural Networks. Sensors, 16(8): 1222, pp. 1-23.
Sankaran, S., Khot, L. R., Espinoza, C. Z., Jarolmasjed, S., Sathuvalli, V. R., Vandemark, G. J., Miklas, P. N., Carter, A. H., Pumphrey, M. O., Knowles, N. R., & Payek, M. J. (2015). Low-altitude, high-resolution aerial imaging systems for row and field crop phenotyping: A review. European Journal of Agronomy, 70, 112-123. https://doi.org/10.1016/j.eja.2015.07.004
Schueller, John K. "Agricultural cybernetics: Yanbo Huang and Qin Zhang (2021), ISBN: 978-3-030-72102-2." (2022): 107028.
Schueller, J.K., 2021. Opinion: Opportunities and Limitations of Machine Vision for Yield Mapping. Frontiers in Robotics and AI, 8, p.627280.
Schulte, N. L. Timm, E. J. & Brwon, G. K. (1994). 'Redhaven' peach impact damage thresholds. In HortScience : a publication of the American Society for Horticultural Science pp. 1052-1055.
Schupp, J., and T. Baugher. 2011. Peach blossom string thinner performance improved with selective pruning. HortScience. 46:1486-1492.
Schupp, J., H. E. Winzeler, T. M. Kon, R. P. Marini, T. A. Baugher, L. F. Kime, and M. A. Schupp. 2017. A method for quantifying whole-tree pruning severity in mature tall spindle apple plantings. HortScience 52:1233-1240.
Senthilnath, J., Dokania, A., Kandukuri, M., Ramesh, K. N., Anand, G. & Omkar, S. N. 2016. Detection of tomatoes using spectral-spatial methods in remotely sensed RGB images captured by UAV. Biosystems Engineering, 146: 16-32.
Seyyedhasani, H., Dvorak, J. (2017). Using the Vehicle Routing Problem to Reduce Field Completion Times with Multiple Machines. Computers and Electronics in Agriculture. 134. March 2017. 142-150. http://dx.doi.org/10.1016/j.compag.2016.11.010
Shafiekhani, A., Kadam, S., Fritschi, F. B., & DeSouza, G. N. (2017). Vinobot and Vinoculer: Two Robotic Platforms for High-Throughput Field Phenotyping. Sensors, 17(1). https://doi.org/10.3390/s17010214
Shah, D., L. Tang, J. Gai, R. Putta-Venkata. 2016. Development of a mobile robotic phenotyping system for growth chamber-based studies of genotype x environment interactions. IFAC-PapersOnLine. 49(16):248-253. https://doi.org/10.1016/j.ifacol.2016.10.046
Siddiqua, A., Kabir, M. A., Ferdous, T., Ali, I. B., & Weston, L. A. (2022). Evaluating Plant Disease Detection Mobile Applications: Quality and Limitations. Agronomy-Basel, 12(8). https://doi.org/10.3390/agronomy12081869
Siemens, M.C. Robotic weed control. 2014. In Proc. 66th Annual California Weed Science Society 66: 76-80. Salinas, Calif.: California Weed Science Society.
Siemens, M.C., Godinez, Jr., V. & Gayler, R.R. 2021. Centimeter Scale Resolution Spot Sprayer for Precision In-Row Weed Control. In Proc. 73rd Annual California Weed Science Society 73:44. Salinas, Calif.: California Weed Science Society.
Silva, G., Tomlinson, J., Onkokesung, N., Sommer, S., Mrisho, L., Legg, J., Adams, I. P., Gutierrez-Vazquez, Y., Howard, T. P., Laverick, A., Hossain, O., Wei, Q. S., Gold, K. M., & Boonham, N. (2021). Plant pest surveillance: from satellites to molecules. Emerging Topics in Life Sciences, 5(2), 275-287. https://doi.org/10.1042/Etls20200300
Sishodia, R. P., Ray, R. L., & Singh, S. K. (2020). Applications of Remote Sensing in Precision Agriculture: A Review. Remote Sensing, 12(19). https://doi.org/10.3390/rs12193136
Silwal, A., Davidson, J. R., Karkee, M., Mo, C. K., Zhang, Q. & Lewis, K. 2017. Design, integration, and field evaluation of a robotic apple harvester. Journal of Field Robotics, 34(6): 1140-1159.
Sivamaran, B. & Burks, T. F. (2006). Geometric performance indices for analysis and synthesis of manipulators for robotic harvesting. In Transactions of the ASABE pp. 1589-1597.
Slaughter, D. C. Hinsch, R. T. & Thompson, J. F. (1993). Assessment of vibration injury to Bartlett pears. In Transactions of the ASAE pp. 1043-1047.
Slaughter, D. C. & Crisosto, C. H. (1998). Nondestructive internal quality assessment of kiwifruit using near- infrared spectroscopy. In Seminars in food analysis pp. 131-140.
Smitt, C., Halstead, M., Ahmadi, A., & McCool, C. (2022). Explicitly Incorporating Spatial Information to Recurrent Networks for Agriculture. Ieee Robotics and Automation Letters, 7(4), 10017-10024. https://doi.org/10.1109/Lra.2022.3188105
Tang et al. (2015) developed a real-time crop stand analyzer for high-throughput infield crop sensing.
Tang, L., J. Li, Y. Bao, J. Jin, A.D. Nakarmi. 2017. Crop stand analyzer using reflective laser proximity sensors. U.S. Patent 9,804,097
Tao, Y. Morrow, C. T. Heinemann, P. H. & Sommer, H. J., III (1995). Fourier-based separation technique for the shape grading of potatoes using machine vision. In Transactions of the ASAE pp. 949-957.
Terentev, A., Dolzhenko, V., Fedotov, A., & Eremenko, D. (2022). Current State of Hyperspectral Remote Sensing for Early Plant Disease Detection: A Review. Sensors, 22(3). https://doi.org/10.3390/s22030757
Thai, C. N. & Shewfelt, R. L. (1990). Peach quality changes at different constant storage temperatures: empirical models. In Transactions of the ASAE pp. 227-233.
Throop, J. A. Aneshansley, D. J. & Upchurch, B. L. (1994a). Camera system effects on detecting watercore in 'Red Delicious' apples. In Transactions of the ASAE pp. 873-877.
Timm, E. J. Armstrong, P. R. Brown, G. K. & Beaudry, R. M. (1993). A portable instrument for measuring firmness of cherries and berries. In Paper p. 17 p
Upchurch, B. L. Affeldt, H. A. Hruschka, W. R. Norris, K. H. & Throop, J. A. (1990). Spectrophotometric study of bruises on whole, 'Red Delicious' apples. In Transactions of the ASAE pp. 585-589.
Upchurch, B. L. Affeldt, H. A. Hruschka, W. R. & Throop, J. A. (1991). Optical detection of bruises and early frost damage on apples. In Transactions of the ASAE pp. 1004-1009.
Upchurch, B. L. Miles, G. E. Stroshine, R. L. Furgason, E. S. & Emerson, F. H. (1987). Ultrasonic measurement for detecting apple bruises. In Transactions of the ASAE - American Society of Agricultural Engineers pp. 803-809.
Vougioukas, S.G., Arikapudi R., Munic, J. (2016). A Study of Fruit Reachability in Orchard Trees by Linear-Only Motion. Journal: IFAC-PapersOnLine, 49(16), pp.277-280. (From Peer reviewed proceedings of the 5th Agricontrol Conference, Seattle, USA.) https://doi.org/10.1016/j.ifacol.2016.10.051
Vougioukas, S.G. (2019). Agricultural Robotics. Annual Review of Control, Robotics, and Autonomous Systems. 2:365-392. https://doi.org/10.1146/annurev-control-053018-023617
Xie, C. Q., & Yang, C. (2020). A review on plant high-throughput phenotyping traits using UAV-based sensors. Computers and Electronics in Agriculture, 178. https://doi.org/10.1016/j.compag.2020.105731
Xu, R., & Li, C. Y. (2022). A Review of High-Throughput Field Phenotyping Systems: Focusing on Ground Robots. Plant Phenomics, 2022. https://doi.org/10.34133/2022/9760269
Yang, G., Liu, J., Zhao, C., Li, Z., Huang, Y., Yu, H., ... & Yang, H. (2017). Unmanned aerial vehicle remote sensing for field-based crop phenotyping: current status and perspectives. Frontiers in plant science, 8, 1111.
Ye, Y., Wang, Z., Jones, D., He, L., Taylor, M. E., Hollinger, G. A., & Zhang, Q. (2017). Bin-Dog: A Robotic Platform for Bin Management in Orchards. Robotics, 6(2), 12.
Zhang, Z., P.H. Heinemann, J. Liu, J.R. Schupp, and T.A. Baugher. (2016). Design and field test of a low-cost apple harvest-assist unit. Transactions of ASABE. 59(5):1165-1180
Zhang, X., He, L., Karkee, M., Whiting, M. D., & Zhang, Q. (2020). Field evaluation of targeted shake-and-catch harvesting technologies for fresh market apple. Transactions of the ASABE, 63(6), 1759-1771.
Zhang, B., Xie, Y., Zhou, J., Wang, K., & Zhang, Z. (2020b). State-of-the-art robotic grippers, grasping and control strategies, as well as their applications in agricultural robots: A review. Computers and Electronics in Agriculture, 177, 105694
Zheng, C. W., Abd-Elrahman, A., & Whitaker, V. (2021). Remote Sensing and Machine Learning in Crop Phenotyping and Management, with an Emphasis on Applications in Strawberry Farming. Remote Sensing, 13(3). https://doi.org/10.3390/rs13030531
Zhang, W. L., Wang, J. Q., Liu, Y. X., Chen, K. Z., Li, H. B., Duan, Y. L., Wu, W. B., Shi, Y., & Guo, W. (2022). Deep-learning-based in-field citrus fruit detection and tracking. Horticulture Research, 9. https://doi.org/10.1093/hr/uhac003
Zhou, J., Vong, C. N., & Zhou, J. (2022). Imaging technology for high-throughput plant phenotyping. In Sensing, Data Managing, and Control Technologies for Agricultural Systems (pp. 75-99). Springer, Cham.