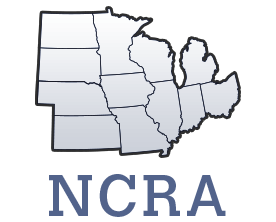
NCERA_old180: Precision Agriculture Technologies for Food, Fiber, and Energy Production
(Multistate Research Coordinating Committee and Information Exchange Group)
Status: Inactive/Terminating
Date of Annual Report: 02/03/2018
Report Information
Period the Report Covers: 08/18/2016 - 02/02/2018
Participants
Brief Summary of Minutes
Meeting called to order at 8:42 a.m. CDT by NCERA 180 Chair Ganesh Bora, Mississippi State University
Ajay Sharda was confirmed via telephone as Vice Chair of the committee for 2017 and Chair/Meeting host for 2018.
2016 meeting minutes were distributed via email prior to the meeting and approved unanimously (m: Fulton/ s: Saraswat).
Update on USDA NIFA (Steve Thompson)
- State of the budget: Level budget
- Sonny Perdue New Secretary
- Engineering Program; National Robotics Initiative; Cyber Physical Systems. Panel submitting top 5 for National Robotics which is 12%-20% funding rate. NIFA AFRI Ag. Engineering 12% funding rate. Cyber physical systems will not be paneled until late August and decisions January 2018.
- Cyber Physical Systems $5M, Robotics $3M,
- Precision ag/remote sensing there must be something different, new, or innovative because of saturation in the past.
- Engineering Deadline is June 8, 2017 and do not wait until the last minute!!!
- Steve attended Ag. Innovations Conference:
- No faculty there
- Interesting to see what crop consultants dealing with
- On-the-Go data collection and management.
- Hybrid evaluation on-the-go with yield, variety, and soil type
- Remote sensing – track insect and disease interpretation of remote sensing data
- Personal weather stations
- Yield monitor calibration is still an issue (extension)
- Speed of moving data and challenges of moving data.
- Possibly pair with other societies or people outside the ag. realm for future proposals.
- Change next year: NIFA AFRI Ag. Eng. RFA is research only which has no benefit/impact to outreach. Do not include extension component this year (2017). Expand NIFA AFRI Ag. Eng. to outreach and teaching components (2018).
- Submit questions to Ganesh and Steve will respond due to abbreviated report.
Updates from upcoming meetings and conferences:
- ICPA – June 24-27, 2018, Le Centre Sheraton, Montreal Quebec, Canada
- 10 Graduate Student Awards, 1 Senior Scientist Award, and 1 Young Scientist Award available.
- Conference is stable and external sponsors are not required.
- ECPA – July 16-20, 2017, Edinburgh, Scotland (http://www.ecpa2017.com/)
- InfoAg
- 25 years in existance and last year changed to an annual event
- Conference is well attended.
- July 25-27, 2017 in St. Louis, MO
- Fulton encourages attendance: good for networking
- ASABE AIM, July 16-19, 2017 in Spokane, WA
- Precision Ag., Machinery Automation, UAV/UAS Standards committees apply to this group.
- Standards being developed: Evaluate Variable Rate Applicators Standard has been accepted, Yield Monitor Data Standard in progress.
- Asian/Australian Precision Ag. Conference; October 16-18, 2017, Hamilton, New Zealand
- Planter Research Group; November 6-8, 2017, Burr Ridge, IL
Big Data Discussions and Efforts
- Need for a working group between professional organizations (ASA, CSSA, SSSA, and ASABE) that aim to consolidate and focus on big data ag issues.
- Joby – Big data conference grant as a collaboration from NCERA 180 group: Write a paper on legal hurdles of collecting data at universities with open data records request. Maybe reach out to law-school for collaboration and something else like social sciences to assess data privacy.
- John N. – focus on data privacy as well as data management
- Fulton: See what Steve says, but put a deliverable in as write a paper.
- Dharmendra: S1069 UAV group divided into 4 and collaborated to submit a grant proposal. NCERA 180 should set collaborations as the same. Making pitch as a USDA previously approved regional project.
- Raj: Bring together diverse groups of researchers that come together to submit multi-state projects. Contribution to the science literature from NCERA directly. @ Nebraska meeting getting priority language in RFA that focuses on precision ag. Have Steve Thompson help get some priority language in RFA. Create a sub-committee from this group (5 people).
Old Business
- 2016 Annual Report not discussed due to Fulton needing to depart early to catch a flight.
New Business
- Future Group Initiatives and Outcomes
- White paper(s): Ag. Big Data Issues
- Special issue of a journal on data issues
- Precision Ag. Journal or Frontiers of plant science (Raj)
- 6-10 papers on different aspects of data privacy/security and an executive summary
- Computer science experts on ideas to remove georeference info. and anonymize ag. data
- What is the difference in the info. I can obtain in pay for service vs. public information
- University public records and data privacy issue
- Data collection on-the-go, how to manage and pass data effectively, and training for data management
- Joby to identify and chair sub-committee to convene sometime in mid-June
- Develop cross-disciplinary conference proposal to NSF and/or USDA for Ag. Big Data issues information gathering, networking, and collaboration development. Brenda and others
- Send a representative group sub-committee to meet with Steve Thompson to inquire about focusing RFA language on precision ag specific concerns. Raj
- Special issue of a journal on data issues
- Incoming Vice Chair
- Dharmendra nominated Brian Luck as Vice Chair for 2018 (s: Ganesh). Nomination was accepted by acclamation.
- 2019 meeting will be held in Madison, WI.
- White paper(s): Ag. Big Data Issues
Announcements
- USDA NIFA Higher Challenge Grant Project for developing precision ag. curriculum for the nation. 20 land grant schools involved. Two text books developed but not published. Engagement of 1890 schools and 1994 schools. Fly them to Colorado State for hands-on training and equipment demonstration. July 31- Aug. 4, 2017. Travel partially or fully funded by the project. Raj asks that this group help recruit 1890 and 1994 faculty.
- Distribute announcement to NCERA 180 group and invite colleagues.
- Next conference call for the project is May 25, 2017.
- Mississippi State University recently implemented 2+2 precision ag program in collaboration with two year technical colleges. Certificate program in undergrad and graduate in precision ag.
- Motion to adjourn (m: B. Luck/ s: Lee): Adjourned 11:25 a.m. CDT.
Accomplishments
<p><strong><span style="text-decoration: underline;">NCERA 180 2017 Accomplishments</span></strong></p><br /> <p>Members made tremendous contribution to knowledge on production machinery, variable rate input decision and application strategies, technology utilization, agronomic practices and enhancing optimal input use to improve yields and economic viability of producers. Research projects conducted and output/outcomes are listed below:</p><br /> <ul><br /> <li><strong>Assess how consistently soil apparent Electrical Conductivity (EC<sub>a</sub>) derived management zones characterize soil water content at the field scale and to determine whether apparent soil electrical conductivity coupled with additional soil properties could further improve the characterization of soil water content.</strong></li><br /> </ul><br /> <p style="padding-left: 30px;"><span style="text-decoration: underline;">Output/Outcomes: Ongoing project</span></p><br /> <ul><br /> <li><strong>Projects on N trials, seeding rate trials, planter technology evaluation, pinch-row compaction, remote sensing, strip-tillage and manure application</strong></li><br /> </ul><br /> <p style="padding-left: 30px;"><span style="text-decoration: underline;">Output/Outcomes</span></p><br /> <ol><br /> <li><em>Nitrogen projects indicated that a reduced rate of applied N would maximize yield and profit over traditional applied N rates within the 180 to 200 lbs acre. These results support the notion that late-season N strategies can be profitable if applied N is withheld through side-dress. There may be years like the 2017 Ohio growing conditions a profitable late-season strategy includes not operating a high-clearance machine between V14 and R corn growth stages resulting in saved N costs.</em></li><br /> <li><em>Soybean seeding rate trials indicated an average of 87% emergence over all trials with optimum seeding rates in the 100k to 120k seeds per acres; a deviation from traditional optimum seeding rates. </em></li><br /> </ol><br /> <ul><br /> <li><strong>Assess potential data layers that farmers can collect with commercial digital tools today. This project was called “terra” determining the level of data that can be collected on 1 corn plant.</strong></li><br /> </ul><br /> <p style="padding-left: 30px;"><span style="text-decoration: underline;">Output/Outcomes</span></p><br /> <p style="padding-left: 30px;"><em>1. Results revealed that 18.4 GB of data could be collected with today’s technologies generating nearly 2500 unique data files.</em></p><br /> <ul><br /> <li><strong>Developing sensors that measure plants in higher resolution, with the capability to independently measure crop stress in individual regions of the plant canopy.</strong></li><br /> </ul><br /> <p style="padding-left: 30px;"><span style="text-decoration: underline;">Output/Outcomes: Ongoing project</span></p><br /> <ul><br /> <li><strong>Understanding effects of different perineal crops planted in headlands instead of grains or legumes.</strong></li><br /> </ul><br /> <p style="padding-left: 30px;"><span style="text-decoration: underline;">Output/Outcomes: Ongoing project</span></p><br /> <ul><br /> <li><strong>Access the carbon life-cycle analysis of different tillage and site-specific management approaches, including residue removal, in an effort to improve the carbon balance of precision agriculture practices.</strong></li><br /> </ul><br /> <p style="padding-left: 30px;"><span style="text-decoration: underline;">Output/Outcomes: Ongoing project</span></p><br /> <ul><br /> <li><strong>Development of an immature green citrus fruit yield mapping, a study conducted to compare the performance of three different cameras (color, near-infrared, and depth cameras).</strong></li><br /> </ul><br /> <p style="padding-left: 30px;"><span style="text-decoration: underline;">Output/outcomes</span></p><br /> <ol><br /> <li><em>A circular object detection method, ‘CHOICE’, was proposed and the near-infrared images yielded the best detection results with a 96% detection accuracy. A prototype yield mapping system for immature citrus fruit was developed with an autonomous navigation and a fruit detection systems. A faster R-CNN network yielded a 77% correct fruit detection accuracy in the validation images. </em></li><br /> <li><em>A low-cost portable soil apparent electrical conductivity sensor was developed for use in mountainous areas and small farms to collect georeferenced data at various user-defined signal frequencies. The sensor was tested in a laboratory and a coffee field, and yielded good results. </em></li><br /> </ol><br /> <ul><br /> <li><strong>Effect of planter downforce on seed spacing, depth and emergence uniformity; and quantify seed singulation using soy-protein based seed lubricant for planters.</strong></li><br /> </ul><br /> <p style="padding-left: 30px;"><span style="text-decoration: underline;">Output/outcomes </span></p><br /> <ol><br /> <li><em>Results indicated that seeding depth was maintained within the 0.25 inch of target when selecting a downforce target of 220 lbs; and spacing COV varied from 18% to 32% for speeds from 4.5mph through 10 mph.</em></li><br /> <li><em>Seed lubrication study showed that there is no significant seed singulation and flowability between the new soy-protein based seed lubricant and other commercially available lubricants and the round shape of soy-based protein might be less abrasive compared to particle shape of other lubricants.</em></li><br /> </ol><br /> <ul><br /> <li><strong>Assess the accuracy of thermal infrared imaging to identify drought and SDS resistant varieties were conducted.</strong></li><br /> </ul><br /> <p style="padding-left: 30px;"><span style="text-decoration: underline;">Output/outcomes </span></p><br /> <ol><br /> <li><em>Preliminary results exhibited that there was strong correlation between elevated canopy temperature and varieties which were not resistant to SDS and drought. Future studies are planned to conduct temporal studies.</em></li><br /> </ol><br /> <ul><br /> <li><strong>Study was conducted to determine the effect of aftermarket closing wheels on corn plant emergence and yield. Replicated studies were conducted at four locations within Wisconsin, representing four soil types and climates.</strong></li><br /> </ul><br /> <p style="padding-left: 30px;"><span style="text-decoration: underline;">Output/outcomes</span></p><br /> <ol><br /> <li><em>Preliminary results indicated the aftermarket wheels resulted in higher final emergence as compared to standard rubber closing wheels. </em></li><br /> <li><em>New data collection systems were developed to better understand work states in the absence of Controller Area Network data on older transport vehicles.</em></li><br /> </ol><br /> <ul><br /> <li><strong>Investigate different sensors aboard UAVs for agricultural crop</strong></li><br /> </ul><br /> <p style="padding-left: 30px;"><span style="text-decoration: underline;">Output/outcomes</span></p><br /> <ol><br /> <li>Invasive and noxious weeds - Researchers use UAS imagery to monitor leafy spurge and purple loosestrife in ditches and on rangeland and other public and private land.</li><br /> <li>Weed identification - UAS imagery detects troublesome weeds such as kochia, waterhemp and redroot pigweed.</li><br /> <li>Herbicide injury - UAS imagery shows where damage occurred if herbicide isn’t applied at the proper rates or it drifted outside the application area.</li><br /> <li>Plant stand - Sensors provide data on a variety of issues, including when seeds germinate and the number of plants growing per acre.</li><br /> <li>Herbicide resistance - Sensors on UAS flying over a field hours after a herbicide application can detect whether weeds are resistant to the chemical. Herbicide-sensitive weeds are about 5 degrees warmer than resistant weeds because healthy plants have enough water in their cells to keep them cooler.</li><br /> </ol><br /> <ul><br /> <li><strong>Teaching, extension and outreach including new courses, online course modules, research /extension talks, website content, videos and extension publications to promote adoption, and expedite applications across cropping systems and regions; and experiential learning opportunities in precision applications for secondary education, community colleges and universities.</strong></li><br /> </ul><br /> <p style="padding-left: 30px;"><span style="text-decoration: underline;">Output/outcomes</span></p><br /> <ol><br /> <li><em>Numerous extension talks; precision ag websites at universities; producer collaborative on-farm research to develop crop input management practices and machine technology implementation; extension publications; focused workshops on specific precision technologies, data management, and UAV</em>.</li><br /> </ol>Publications
<p> </p><br /> <p>Arriaga, F. J., S. P. Conley, B. M. Jensen, C. A.M. Laboski, J. G. Lauer, B. D. Luck, P. D. Mitchell, and D. L. Smith. 2017. Grain Management Considerations in Low-Margin Years. University of Wisconsin Extension Learning Store Article # A4137.</p><br /> <p>Barocco, R., W. S. Lee, and G. Hortman. 2017. Yield mapping hardware components for grains and cotton using on-the-go monitoring systems. UF/IFAS EDIS AE518. <a href="http://edis.ifas.ufl.edu/ae518">http://edis.ifas.ufl.edu/ae518</a>.</p><br /> <p>Choi, D., W. S. Lee, J. K. Schueller, R. Ehsani, F. Roka and J. Diamond. 2017. A performance comparison of RGB, NIR, and depth images in immature citrus detection using deep learning algorithms for yield prediction. ASABE Paper No. 1700076. St. Joseph, Mich.: ASABE.</p><br /> <p>Colley III, R.T. J.P. Fulton, S.S. Virk, and E. Hawkins. 2017. Dry Fertilizer Distribution Uniformity of Two Sources when Applying with VRT Spinner-disc Spreader. In Proceedings of ASABE AIM 2017, Spokane, WA. Paper No. 1700094.</p><br /> <p>Dix, P. D. Flippo and A. Sharda. 2017. An Innovative Approach to Aphid Mitigation. IOSR Journal of Agriculture and Veterinary Science. 10(7): 30-33.</p><br /> <p>Drewry, J. L., C. Y. Choi, J. M. Powell, and B. D. Luck. 2017. Computational model of methane and ammonia emissions from dairy barns: Development and validation. Computers and Electronics in Agriculture. In Press. http://dx.doi.org/10.1016/j.compag.2017.07.012</p><br /> <p>Drewry, J. L., M. R. Mondaca, B. D. Luck, C. Y. Choi. 2017. A computational fluid dynamics model of biological heat and gaseous generation in a dairy holding area. Transactions of the ASABE. https://doi.org/10.13031/trans.12394. Accepted and In Press.</p><br /> <p>Dudenhoeffer, N. E., Luck, B. D., Digman, M. F., & Drewry, J. L. (2017). Simulation of the forage harvest cycle for asset allocation. In press. DOI: 10.13031/aea.12619</p><br /> <p>Ferraretto, L. F., R. D. Shaver, and B. D. Luck. 2017. Silage review: Recent advances and future technologies for whole-plant and fractionated corn silage harvesting. Journal of Dairy Science. https://doi.org/10.3168/jds.2017-13728. Accepted and In Press.</p><br /> <p>Gan, H., W. S. Lee, and V. Alchanatis. 2017. Development of a prototype of an immature citrus fruit yield mapping system. ASABE Paper No. 1700164. St. Joseph, Mich.: ASABE.</p><br /> <p>Geomar, M. Corassa,* T. J. C. Amado, T. Liska, A. Sharda, J. Fulton, and I. A. Ciampitti. 2018. Planter technology to reduce double-planted areas and improve corn and soybean yields. Agronomy Journal. 110(1). doi:10.2134/agronj2017.07.0380</p><br /> <p>Griffin, T.W., Miller, N.J., Bergtold, J., Shanoyan, A., Sharda, A., and Ciampitti, I.A. 2017. Farm’s Sequence of Adoption of Information-Intensive Precision Agricultural Technology. Applied Engineering in Agriculture. 33(4): 521-527. http://doi.org/10.13031/aea.12228.</p><br /> <p>Harmon, J. D. and B. D. Luck. 2017. A case study of a commercial dairy and a custom harvest operation to evaluate forage harvest efficiency. ASABE Annual International Meeting. Spokane, WA.</p><br /> <p>Harmon, J. D., Luck, B. D., Shinners, K. J., Anex, R. P., & Drewry, J. L. (2017). Time–motion analysis of forage harvest: A case study. In press. DOI: 10.13031/trans.12484</p><br /> <p>Hawkins, E., J.P. Fulton, and K. Port. 2017. ANR-8: Tips for Calibrating Grain Yield Monitors—Maximizing Value of Your Yield Data. The Ohio State University Extension, Columbus, Ohio. 8pp. <a href="https://ohioline.osu.edu/factsheet/anr-8">https://ohioline.osu.edu/factsheet/anr-8</a></p><br /> <p>Hawkins, E., J.P. Fulton, and K. Port. 2017. FABE-565-16: Using Soil Electrical Conductivity (EC) to Delineate Field Variation. The Ohio State University Extension, Columbus, Ohio. 9pp. <a href="http://ohioline.osu.edu/factsheet/fabe-565">http://ohioline.osu.edu/factsheet/fabe-565</a>.</p><br /> <p>Khanal, S., J.P. Fulton, and S.A. Shearer. 2017. An overview of current and potential applications of thermal remote sensing in precision agriculture. Computers and Electronics in Agriculture. 139(15June2017): 22-32.</p><br /> <p>Khanal, S., J.P. Fulton, E. Hawkins, K. Port and A. Klopfenstein. 2017. FABE-554.1: Remote Sensing in Precision Agriculture: Best Management Practices for Addressing Challenges with Imagery Quality. The Ohio State University Extension, Columbus, Ohio. 7pp. <a href="http://ohioline.osu.edu/factsheet/fabe-5541">http://ohioline.osu.edu/factsheet/fabe-5541</a></p><br /> <p>Khedher Agha, M. K., R. A. Bucklin, W. S. Lee, R. W. Mankin, and A. R. Blount. 2017. Effect of drying conditions on triticale seed germination and weevil infestation. <em>Trans. ASABE</em> 60(2): 571-575.</p><br /> <p>Khedher Agha, M. K., W. S. Lee, C. Wang, R. W. Mankin, A. R. Blount, R. A. Bucklin, and N. Bliznyuk. 2017. Detection and prediction of <em>Sitophilus oryzae</em> infestations in triticale via visible and near infrared spectral signatures. <em>Journal of Stored Products Research</em> 72: 1-10.</p><br /> <p>Khosla, R. and L. Longchamps. 2017. Precision Maize cultivation. In. Achieving sustainable cultivation of maize Vol 2: Cultivation techniques, pest and disease control. D. Watson (ed) In press</p><br /> <p>Kleinman, P.J.A., A. R. Buda, A. N. Sharpley and R. Khosla. 2017. Elements of Precision Manure Management. In Precision Conservation. J. Delgado (ed) doi:10.2134/agronmonogr59.2013.0023</p><br /> <p>Lal, R., A. Sharda and P. Prabhakar. 2017. Optimal Multi-robot Path Planning for Pesticide Spraying in Agricultural Fields. 56th IEEE Conference on Decision and Control (CDC), 2017.</p><br /> <p>Luck, B. D., J. D. Davis, J. L. Purswell, A. S. Kiess, and S. J. Hoff. 2017. Assessing the effect of house size and design on air velocity distribution in commercial broiler houses. Transactions of the ASABE 60(4): 1313-1323</p><br /> <p>Luck, B. D., J. L. Drewry, J. W. Nelson. 2017. Unmanned Aerial Vehicles (Drones): What you need to know for use in Agriculture. University of Wisconsin Extension Learning Store. In Press.</p><br /> <p>Luck, B. D.. 2017. Calibrate Your Yield Monitor for Greater Accuracy During Harvest. UW Extension Learning Store Publication. University of Wisconsin Extension Learning Store Article #A4146.</p><br /> <p>Mangus, D., A. Sharda, A. Engelhardt, D. Flippo, R. Strasser, J. D. Luck and T. Griffin. 2017. Analyzing the nozzle spray fan pattern on an agricultural sprayer using pulse width modulation technology to generate an on-ground coverage map. Trans. Of ASABE. 60(2):315-325.</p><br /> <p>Mangus, D., A. Sharda, D. Flippo, R. Strasser, and T. Griffin. 2017. Development of high speed camera hardware and software package to evaluate real-time electric seed meter accuracy of a variable rate planter. Computers and Electronics in Agriculture. 142: 314-325. https://doi.org/10.1016/j.compag.2017.09.014</p><br /> <p>Mizuta, K., S. Grunwald, C. M. Clingensmith, G. M. Vasques, W. S. Lee, M. A. Phillips, W. P. Cropper, X. Xiong, B. D. Myers. Novel Pedometrics-Econometrics Modeling Using VNIR spectroscopy: developing soil carbon sequestration capability index. Soil Science Society of America, October</p><br /> <p>Mizuta, K., S. Grunwald, C. M. Clingensmith, W. P. Cropper, W. S. Lee, G. M. Vasques, M. A. Phillips, W. G.Harris, B. M. Myers, and X. Xiong. Modeling pedo-econimetric carbon scores with VNIR spectroscopy. Poster Presentation in Pedometrics 2017, June 26-July 1, 2017, Wageningen, Netherlands.</p><br /> <p>Pengmin, P., T. McDonald, J.P. Fulton, B. Via, and J.Hung. 2017. Simultaneous moisture content and mass flow measurements in wood chip flows using coupled dielectric and impact sensors. Sensors in Agriculture and Forestry. Sensors 2017, 17(1), 20.</p><br /> <p>Pourreza, A., W. S. Lee, E. Czarnecka, L. Verner, and W. Gurley. 2017. Feasibility of using the optical sensing techniques for early detection of Huanglongbing in citrus seedlings. <em>Robotics</em> 6(11). Doi:10.3390/robotics6020011.</p><br /> <p>Qiang Cao, Y. Miao, G Feng, X. Gao, B. Liua, Y. Liu, F. Lie, R. Khosla, D. J. Mulla, F. Zhang. 2017. Improving nitrogen use efficiency with minimal environmental risks using an active canopy sensor in a wheat-maize cropping system. Field Crops Research 214 (2017) 365–372</p><br /> <p>Queiroz, D. M., W. S. Lee, J. K. Schueller, and E. D. T. Santos. Development and test of a low cost portable soil apparent electrical conductivity sensor using a Beaglebone Black. ASABE Paper No. 1700062. St. Joseph, Mich.: ASABE.</p><br /> <p>Sebastian Varela, Y. Assefa, P. V. Vara Prasad, N. R. Peralta, T. W. Griffin, A. Sharda, A. Ferguson, I. A. Ciampitti. 2017. Spatio-temporal evaluation of plant height in corn via unmanned aerial systems. J. Appl. Remote Sens. 11(3), 036013 (2017). http://doi.org/10.1117/1.JRS.11.036013.</p><br /> <p>Seigfried, J, R. Khosla, and L. Longchamps. 2017. Infrared thermometry to quantify in-field soil moisture variability. J. of Crop Improvement Vol. 31 (1) 2017</p><br /> <p>Sharda, A., L. Haag, T.Griffin, J.P. Fulton, S. Badua, and I. Ciampitti. 2017. Planter downforce system for seed depth uniformity. K-State Research and Extension. MF3331. <a href="https://www.bookstore.ksre.ksu.edu/pubs/MF3331.pdf">https://www.bookstore.ksre.ksu.edu/pubs/MF3331.pdf</a></p><br /> <p>Shuaibu, M., W. S. Lee, Y. K. Hong, and S. Kim. 2017. Detection of apple Marssonina blotch disease using particle swarm optimization. <em>Trans. ASABE</em> 60(2): 303-312.</p><br /> <p>Virk, S.S., J.P. Fulton, W.M. Porter and G.L. Pate. 2017. Field Validation of Seed Meter Performance at Varying Seeding Rates and Ground Speeds. In Proceedings of ASABE AIM 2017, Spokane, WA. Pages 1-9.</p>Impact Statements
- Reserach, educational and outreach endeavors will continue to provide crop input management and machine technology implementation recommendations to all stakeholders with a goal to enhance productivity, profitability and promote healthy environment for all
Date of Annual Report: 01/21/2019
Report Information
Period the Report Covers: 05/18/2017 - 05/23/2018
Participants
Campitti, Ignacio (ciampitti@ksu.edu) – Kansas State University; Kovacs, Peter (Peter.Kovacs@sdstate.edu) – South Dakota State University; Tian, Lei (lei-tian@illinois.edu) – University of Illinois; Miao, Yuxin (ymiao@umn.edu) – University of Minnesota; Nowatzski, John (john.nowatzki@ndsu.edu) – North Dakota State University; Griffin, Terry (twgriffin@ksu.edu) – Kansas State University; Franzen, Aaron (Aaron.Franzen@sdstate.edu) – South Dakota State University; Hawkins, Elizabeth (hawkins.301@osu.edu) – Ohio State University; Czarnecki, Joby (joby.czarnecki@msstate.edu) – Mississippi State University; Sharda, Ajay (asharda@k-state.edu) – Kansas State University; Andrade, Pedro (pandrade@ag.arizona.edu) – University of Arizona; McDonald, Timothy (mcdontp@auburn.edu) – Auburn University; and Mesa, Bruce (email) – AgVoice.Brief Summary of Minutes
The 2018 NCERA180 Annual Meeting was held in Manhattan, Kansas on May 23, 2018 and chaired by Dr. Ajay Sharda. Upcoming 2018 meeting notifications were provided regarding the International Conference on Precision Agriculture, InfoAg, American Society of Agricultural and Biological Engineers Annual International Meeting, and the Austral Asian Precision Agriculture Conference. Discussion was had on data issues in agriculture. Needs were identified for the group to consider focusing on in 2018/2019. Dr. Steven Thomson provided an update on USDA NIFA programs the group should be considering for funding. The 2019 NCERA180 Annual Meeting will be held in Madison, Wisconsin under the direction of Dr. Brian Luck. A Vice Chair was not elected at the meeting, but Dr. Jason Ward of North Carolina State University was later identified, nominated, and elected. Action items were identified as follows: 1) Identify new members; 2) Identify a Vice Chair for 2018/2019; and 3) Send comments on alternate meeting date to Dr. Sharda and Dr. B. Luck.
Accomplishments
<p><strong>Accomplishments:</strong> Institutions made tremendous contribution to knowledge on production machinery, variable rate input decision and application strategies, technology utilization, agronomic practices and enhancing optimal input use to improve yields and economic viability of producers. Research projects conducted with output/outcomes are listed below:</p><br /> <p><span style="text-decoration: underline;">Research:</span></p><br /> <ol><br /> <li><span style="text-decoration: underline;">University of California-Davis:</span> Management zone based Precision Irrigation in Almonds and Grapes (Dr. Shrini K Upadhyaya) – Data obtained during the 2015 growing season have been fully analyzed. Both almond and grape crops presented high correlation between the crop water stress index (CWSI) computed from leaf monitor data and the deficit stem water potential measured by the pressure chamber.<br /> Automated Weeding using Crop Signaling Compound (Dr. David Slaughter) – Progress was made on the development of automated, intra-row weed control technologies for vegetable crops that can accurately and rapidly differentiate crops from weeds, determine their spatial location and automatically kill weeds without damage to the crop.<br /> Precision Yield mapping in orchard crops (Dr. Stavros Vougioukas) – Yield monitors are currently not available for tree fruits, as all are harvested manually. A commercial picking bag was instrumented to measure harvested fruit weight. Results showed a mean error of 0.39 kg, standard deviation 0.42 kg, and 95th percentile 1.04 kg. Major error sources included bag acceleration and body reaction force.<br /> A Center for SmartFarm has been established at UC Davis as a part of one of the campus-wide “BIG IDEA” project.</li><br /> <li><span style="text-decoration: underline;">North Dakota State University:</span> Dakota Prairie Grasslands UAS Project 2018. Benjamin Geaumont, NDSU, Hettinger REC – Aerial surveys using manned aircraft have historically been an important tool for agencies to assess wildlife populations but these surveys can be expensive, dangerous, and difficult to accurately repeat. The goal of this project is to evaluate the feasibility of using UAS to locate and monitor leks of sharp-tailed grouse in the Dakota Prairie Grasslands.<br /> Studies Evaluate Precision Herbicide Applications. Rod Lym, NDSU, Plant Science – Herbicide applications with the PWM system on leafy spurge and Canada thistle resulted in control comparable to applications from a standard boom sprayer at all droplet sizes, except 150 microns. The PWM sprayer can be used to apply herbicides in pasture and rangeland at a variety of travel speeds while maintaining medium-sized or larger droplets for reduced drift and more uniform coverage. <br /> Precision Application of Herbicides for Sustainable Crop Systems. Kirk Howatt, NDSU Plant Science – Herbicides applied in pasture and rangeland with the PWM system provided similar leafy spurge and Canada thistle control compared to traditional boom sprayer with 8002 nozzles as long as the average droplet size exceeded 150 microns. Effects demonstrated in these experiments may result in less than optimal control of weeds; however, results also indicate faster development of herbicide-resistant weeds with PWM sprayers than with conventional systems.<br /> Coated Confectionery Sunflower Kernels for Precision Agriculture. Harjot Sidhu, NDSU Agricultural and Biosystems Engineering – This project aims to hull and coat extra-large hybrid confection sunflower kernels for use in precision planting was sponsored by the National Sunflower Association (NSA). Overall, this study showed that coating the hulled kernels substantially increased seed plantability and crop performance<br /> Prediction of Pork Loin Quality using Online Computer Vision System and Artificial Intelligence Mmodel. Xin Suna, Jennifer Younga, Jeng-Hung Liua, David Newman – The objective of this project was to develop a computer vision system (CVS) for objective measurement of pork loin under industry speed requirement. This research shows that the proposed artificial intelligence prediction model with CVS can provide an effective tool for predicting color and marbling in the pork industry at online speeds.</li><br /> <li><span style="text-decoration: underline;">Colorado State University:</span> The technological hardware requisite for precise water delivery methods such as variable rate irrigation is commercially available. Despite that, techniques to formulate a timely, accurate prescription for those systems are inadequate. Research was conducted to assess if vegetation indices derived from multispectral satellite imagery could assist in quantifying soil moisture variability in irrigated maize production. Our study showed that satellite-derived vegetation indices may be useful for creating time-sensitive characterizations of soil moisture variability. Specifically, the Red Edge Normalized Difference (NDRE) Vegetation Index could quantify soil moisture tension variability at V6 (six leaf) (r2 = 0.850, p = 0.009) and V9 (nine leaf) (r2 = 0.913, p = 0.003) crop growth stages.</li><br /> <li><span style="text-decoration: underline;">University of Nebraska-Lincoln:</span> Project SENSE (Sensors for Efficient Nitrogen Use and Stewardship of the Environment) – 2017 represented the third year of Project SENSE. During the year, we reported on significant project findings from the first two years as we conducted year three field activities. In addition to communicating at five field days and one crop management clinic (reaching over 100 individuals), we were able to report significant savings in N applied using the crop canopy sensor-based approach.</li><br /> <li><span style="text-decoration: underline;">University of Minnesota:</span> Variable Rate Nitrogen (VRN) studies for improved production of maize and better water quality (David Mulla) – Nitrogen pollution from suboptimal management of synthetic N fertilizer continues to pose a challenge, especially for drinking water, in rural Minnesota. Variable rate technology based on in-season sensing is becoming more available and affordable, however, a comprehensive evaluation considering the agronomic, environmental and economic impacts is needed to facilitate acceptance of the technology by growers. VRN subfields displayed significantly and consistently higher Nitrogen Use Efficiency (NUE) values for both growing seasons. Lastly, VRN for maize based on in-season sensing was profitable every year, even in the absence of yield increases, because of the N savings from better utilization of soil N.</li><br /> <li><span style="text-decoration: underline;">Texas A&M University:</span> Alex Thomasson – Commercial off-the shelf systems of UAVs and sensors are touted as being able to collect remote-sensing data on crops that include spectral reflectance, plant height, and canopy temperature. Historically a great deal of effort has gone into quantifying and reducing the error levels in the geometry of UAV-based orthomosaics, but little effort has gone into quantifying and reducing the error of the reflectance, plant-height, and canopy-temperature measurements themselves. We have been developing systems and protocols involving multifunctional GCPs (ground-control points) in order to produce crop phenotypic data that are as repeatable as possible. We believe through continued development these error reductions will increase, such that broad-acre phenotypic data can be collected with a high level of repeatability. We are also working on incorporating temperature calibration into the system so that canopy temperature can be measured precisely and accurately.</li><br /> <li><span style="text-decoration: underline;">University of Wisconsin-Madison:</span> Forage harvest is an important process for Wisconsin agriculture, providing feed for dairy cows. This harvest process is very machinery and labor intensive and requires coordination and communication to be done properly. Dr. Brian Luck and the Agriculture Technology Lab have been investigating forage harvest efficiency and developing tools to help producers and custom harvesters efficiently harvest high-quality feed. The focus of this effort has been on two fronts: 1) Time-motion analysis and vehicle movement efficiency, and 2) Image analysis for particle size determination of chopped and processed corn silage. Conversations with producers and custom harvesters have indicated interest in how the forage harvest process could be made more efficient. Research, published in 2018 (Harmon et al., 2018), showed self-propelled forage harvest efficiency to be 65% over three alfalfa crops and corn silage harvest. Transport vehicles showed an average of 20% idle (not working) time during the harvest 2015 and 2016 harvest. From this work a web-based simulator was developed to assess the effect of different transport vehicles on the time to harvest (Dudenhoeffer et al., 2018). Similarly, producers, custom harvesters, and dairy nutritionists were concerned about the assessing the effectiveness of kernel processors in the field during harvest rather than sending samples off to a lab. An image processing app for smart phones was developed to accomplish this task. SilageSnap was released in 2018 and currently has 338 downloads on Apple and Android devices.<br /> Another research focus area is centered on planting technology and how it benefits producers. Variable-rate starter fertilizer, variable row unit downforce, and furrow closing wheels are being investigated across various Wisconsin soils. Results in 2017 showed 2% better emergence with aftermarket closing wheels (α= 0.10). The addition of starter fertilizer in 2018 seemed to negate these results and no differences in closing wheels were observed. Grant funding has been obtained to investigate planter technology and best practices for high residue organic no-till farming.</li><br /> </ol><br /> <p><span style="text-decoration: underline;">Extension and Education:</span></p><br /> <ol><br /> <li><span style="text-decoration: underline;">University of Nebraska-Lincoln:</span> Nebraska Precision Ag Data Management Workshops – From January to March, 2017, 8 day-long workshops were held in in Nebraska and 2 in Kansas. Eight day-long workshops across Nebraska, 120 attendees 179,470 producer acres and 1.1 M acres of consultant/advisor acres were represented; 108 responded to pre- and post-workshop surveys. 88% of respondents indicated the workshop was above average to one of the best extension events they’ve attended.<br /> Site-Specific Crop Management (AGEN/AGRO/MSYM 431) 3 Credit Hour Course – Student enrollment consisted of 75 agronomy, engineering, and mechanized systems management students in 2017. Students were exposed to a variety of topics related to precision agriculture; 15 computer laboratory exercises were conducted throughout the semester to provide hands-on learning experiences with agricultural data applications.</li><br /> <li><span style="text-decoration: underline;">University of Wisconsin-Madison:</span> Multiple state, regional, and national extension events were attended and presentations delivered on Forage Harvest Logistics, Kernel Processing and SilageSnap, Unmanned Aerial Vehicles and Remote Sensing, and General Precision Agriculture Topics and Big Data. Direct contacts from this effort totaled >1,300. Indirect contacts via website hits and social media impressions on precision agriculture topics exceeded 118,000.</li><br /> </ol>Publications
<p>Arriaga, F. J., B. D. Luck, and G. Slemering. 2018. Managing soil compaction at planting and harvest. University of Wisconsin Extension Learning Store. Article # A4158.</p><br /> <p>Barker, J. B., Franz, T. E., Heeren, D. M., Neale, C. M. U., Luck, J. (2017). Soil moisture monitoring for irrigation management: a geostatistical analysis. Agricultural Water Management, 188(2017), 36-49.</p><br /> <p>Coble, K., Ferrell, S.L., Mishra, A., and Griffin, T.W. 2018. Big Data in Agriculture: A Challenge for the Future. Applied Economics Perspectives and Policy, 40(1):79–96.</p><br /> <p>de Lara, A, R. Khosla, L. Longchamps. 2018. Characterizing spatial variability in soil water content for precision irrigation management. J. Agron.</p><br /> <p>Drewry, J. L., B. D. Luck, J. M. Shutske, and D Trechter. 2018. Quantifying agricultural data generation on Wisconsin farms: A case study. Paper # 1800897. ASABE Annual International Meeting. Detroit, MI.</p><br /> <p>Drewry, J. L., B. D. Luck, R. Willett, E. Rocha, and J. D. Harmon. 2018. Assessing kernel processing score of harvested and processed corn silage via image processing techniques. Paper # 1800888. ASABE Annual International Meeting. Detroit, MI.</p><br /> <p>Dudenhoeffer, N. E., B. D. Luck, M. F. Digman, and J. L. Drewry. 2018. Technical Note: Simulation of the forage harvest cycle for asset allocation. Applied Engineering in Agriculture 34(2): 327-334. https://doi.org/10.13031/aea.12619/.</p><br /> <p>Ferguson, R. B., Luck, J., Thompson, L. J., Parrish, J. D., Crowther, J. D., Mieno, T., Glewen, K., Krienke, B., Krull, D., Mueller, N. M., Ingram, T., Shaver, T. M. (2017). Crop caopy Sensor use with irrigated maize: profit and environmental impacts. (pp. 6). Proceedings of the 11th European Conference on Precision Agriculture. Status: Published</p><br /> <p>Ferguson, R., Luck, J., Stevens, R. H. (2017). Chapter 9: Developing prescriptive soil nutrient maps. Madison, WI: Practical Mathematics for Precision Agriculture. ASA/CSSA/SSSA. Status: Published</p><br /> <p>Gan, H., W.S. Lee, and V. Alchanatis. 2018. A photogrammetry-based image registration method for multi-camera systems - with applications in images of a tree crop. Biosystems Engineering 174: 89-106.</p><br /> <p>Gan, H., W.S. Lee, V. Alchanatis, R. Ehsani, and J. K. Schueller. 2018. Immature green citrus fruit detection using color and thermal images. Computers and Electronics in Agriculture 152: 117-125.</p><br /> <p>Griffin, T.W. 2010. The Spatial Analysis of Yield Data. In M. Oliver (Ed.) Geostatistical Applications for Precision Agriculture. Springer. 295p.</p><br /> <p>Harmon, J. D., B. D. Luck, K. J. Shinners, R. P. Anex, and J. L. Drewry. 2018. Time-motion analysis of forage harvest: A case study. Transactions of the ASABE 62(2): 483-491. https://doi.org/10.13031/trans.12484/.</p><br /> <p>Kim, D.-W., H. S. Yun, S.-J. Jeong, Y.-S. Kwon, S.-G. Kim, W. S. Lee, and H.-J. Kim. 2018. Modeling and testing of growth status for Chinese cabbage and white radish with UAV-based RGB imagery. Remote Sens. 10(4): 563. https://doi.org/10.3390/rs10040563.</p><br /> <p>Lo, T. H., Heeren, D. M., Mateos, L., Luck, J., Martin, D. L., Miller, K. A., Barker, J. B., Shaver, T. M. (2017). Field characterization of root zone available water holding capacity for variable rate irrigation. Applied Engineering in Agriculture, 33(4), 559-572.</p><br /> <p>Lu, J., W. S. Lee, H. Gan, and X. Hu. 2018. Immature citrus fruit detection based on local binary pattern feature and hierarchical contour analysis. Biosystems Engineering 171: 78-90.</p><br /> <p>Luck, B. D., J. L. Drewry, E. Chasen, S. Steffan. 2018. Unmanned aerial systems and remote sensing for cranberry production. 14th International Conference on Precision Agriculture. Montreal, Quebec, Ca.</p><br /> <p>Luck, B. D., N. Dudenhoeffer, M. F. Digman, J. L. Drewry. 2018. Simulation of the forage harvest cycle for asset allocation. Paper # 1800523. ASABE Annual International Meeting. Detroit, MI.</p><br /> <p>Miller, N.J., Griffin, T.W., Ciampitti, I., and Sharda, A. forthcoming Farm Adoption of Embodied Knowledge and Information Intensive Precision Agriculture Technology Bundles. Precision Agriculture https://rdcu.be/6LvT</p><br /> <p>Seigfried, J, R. Khosla, L. Longchamps. 2018. Multispectral satellite imagery to quantify in-field soil moisture variability. J. Soil Water Conserv.</p><br /> <p>Shearer, C. A., Luck, J., Evans, J. T. (2017). Development of a sprayer performance diagnostic tool for better management practices of in-field spraying operations. (pp. 6). Proceedings of the 75th International Conference on Agricultural Engineering. Status: Published</p><br /> <p>Shimwela, M. M., Schubert, T. S., Albritton, M., Halbert, S. E., Jones, D. J., Sun, X., Roberts, P. D., Singer, B. H., Lee, W. S., Jones, J. B., Ploetz, R. C., and van Bruggen A. H. C. 2018. Regional spatial-temporal spread of citrus huanglongbing is affected by rain in Florida. Phytopathology. http://dx.doi.org/10.1094/PHYTO-03-18-0088-R.</p><br /> <p>Shuaibu, M., W. S. Lee, J. K. Schueller, P. Gader, Y. K. Hong, and S. Kim. 2018. Unsupervised hyperspectral band selection for apple Marssonina blotch detection. Computers and Electronics in Agriculture 148: 45-53.</p><br /> <p>Shutske, J. M., D. Trechter, B. D. Luck, J. L. Drewry, M. J. DeWitte, L. Pitman, and M. Kluz. 2018. Assessment of digital capacity, needs and access barriers among crop, dairy, and livestock producers. Paper # 1801320. ASABE Annual International Meeting. Detroit, MI.</p><br /> <p>Torquebiau, E., C., Rosenzweig, A.M. Chatrchyan, N. Andrieu, and R. Khosla 2018. Identifying Climate-Smart Agriculture Research Needs. J. Cahiers Agricultures (France).</p><br /> <p>Varela, S., Dhodda, P., Hsu, W.H., Prasad, P.V.V., Assefa, Y., Peralta, N.R., Griffin, T., Sharda, A., Ferguson, A., and Ciampitti, I.A. 2018. Early-Season Stand Count Determination in Corn via Integration of Imagery from Unmanned Aerial Systems (UAS) and Supervised Learning Techniques. Remote Sensing, 10, 343.</p><br /> <p>Wang, C., W. S. Lee, X. Zou, D. Choi, H. Gan, and J. Diamond. 2018. Detection and counting of immature green citrus fruit based on the Local Binary Patterns (LBP) feature using illumination-normalized images. Precision Agriculture Doi: 10.1007/s11118-018-9574-5.</p><br /> <p>Wilson, G., Laacouri, A., Galzki, J. and Mulla, D. 2017. Impacts of Variable Rate Nitrogen (VRN) on Nitrate-N Losses from Tile Drained Maize in Minnesota, USA. Advances in Animal Biosciences, 8(2): 317-321.</p><br /> <p>Zhang, Y., M. Li, L. Zheng, Q. Qin, W. S. Lee. 2018. Spectral features extraction for estimation of soil total nitrogen content based on modified ant colony optimization algorithm. Geoderma 333: 23-34.</p><br /> <p>Zhang, Y., W. S. Lee, M. Li, L. Zheng, M. A. Ritenour. 2018. Non-destructive recognition and classification of citrus fruit blemishes based on ant colony optimized spectral information. Postharvest Biology and Technology, 143: 119-128.</p>Impact Statements
- Increase crop efficiency and improve management decisions by utilizing remote sensing and UAVs.
Date of Annual Report: 10/17/2019
Report Information
Period the Report Covers: 05/31/2018 - 08/14/2019
Participants
Brief Summary of Minutes
See attachment for complete minutes.
Meeting called to order at 8:38am on 14-August-2019. Dr. Thomson delivered an update on the status of the NIFA relocation to Kansas City. Expect delays and changes as only 1/3 of the staff will be making the move. Updates on other Precision Agriculture meetings were shared. It was suggested that NCERA coordinate with S-1069 to create more collaboration opportunities.
NCERA180 will move to a winter meeting date in January or February starting in 2020 as requested by the members. The 2020 meeting will be in Raleigh hosted by Jason Ward at NC State and the 2021 meeting will be hosted at Purdue by John Evans. Registration has been requested at approximately $125. Optimizing cost for the meetings is helpful for many attendees that may or may not have experiment station support.
There are no programs in USDA to support precision ag. National Program Leaders update RFAs every year. Collectively, Dr. Khosla suggests reaching out to program leaders to suggest that a new priority is needed. NCERA 180 discussed the real need for creating priority areas that cover precision ag, spectral signals for pests, other areas. If we were to focus on small areas. A short white paper from the group could create the language from which to draw a new or revised RFA. It was suggested to form a subcommittee for the whitepaper: Newell Kitchen, Van Kelley, Raj Khosla, Ganesh Bora.
NCERA-180 is up for renewal in 2021 and needs paperwork to start in 2020.
The meeting was adjourned at 10:16a.
Accomplishments
<h2><span style="font-weight: 400;">Colorado State University</span></h2><br /> <p><span style="font-weight: 400;">Four Nitrogen management strategies that incorporates soil based and crop canopy based management of N fertilizer were evaluated in terms of grain yield and NUE</span></p><br /> <p><span style="font-weight: 400;">This project accomplished a number of objectives and associated scientific findings. The four N management strategies studied were: a uniform application of N fertilizer, a variable rate N management strategy that consisted of applying variable rate N across management zones referred to as “MZ”; a proximal sensor based variable rate N management strategy referred to as “RS”; and a variable rate N management strategy based on proximal sensing within each management zone referred to as “MZRS”. </span></p><br /> <p><span style="font-weight: 400;">Results from our study comparing N management strategies were encouraging. It indicated that variable rate N management could be undertaken for both, maintaining or increasing grain yield as well as enhancing NUE. Across the three years for which this analysis was performed, the MZ strategy consistently resulted in grain yields that were either the same as or greater than grain yield obtained when applying N uniformly across the field. The RS and MZRS management strategies resulted in yields that were lower than both the uniform and MZ strategies. However, these differences were typically small in each of the three years. This indicates that with further refining, the RS and MZRS can be improved to maintain or increase grain yield relative the uniform application strategy. However, all three variable N management strategies resulted in significantly improved NUE when compared to uniform N application strategy. Specifically, the two management strategies that incorporated proximal sensing strategy consistently resulted in the largest improvements in NUE. </span></p><br /> <p><span style="font-weight: 400;">Based on the findings of this research, farmers could consider employing strategies for variable rate N management that result significant improvements in input use efficiency. While there exists room for growth, improved implementation of these techniques require technical assistance. Farmers are in need of both support systems and personnel they can rely upon to address their concerns about implementing more nuanced approaches to N management. Findings from this research could be coupled with extension services to enhance growers understanding and appreciation of precision N management and promote adoption. </span></p><br /> <h2><span style="font-weight: 400;">NC State University</span></h2><br /> <h3><span style="font-weight: 400;">Extension Activities</span></h3><br /> <p><span style="font-weight: 400;">The modern agricultural landscape is a data-rich environment. Sensors on agricultural equipment, soils data, yield maps, official variety trials, variable-rate prescriptions, animal monitoring, and UAV imagery could all be used on the farm to help make decisions – if the data are collected, managed, and analyzed properly. The range of digital agriculture products and services has increased in order of magnitude over the last five years. Equipment manufacturers and agricultural service providers are the experts in how to operate their products or integrate them into equipment. The real knowledge gap lies in putting the generated data into action. Key issues are emerging which can be addressed with Extension programmatic efforts: producers are not receiving unbiased information on how and why to use digital agriculture tools along with the potential implications of their decisions, Extension agents are not being seen as knowledgeable advisors in implementing digital agriculture, they need focused training to understand modern tools and how to use them, agricultural service providers need to understand both the limitations and potential of their data products so that meaningful insight is being delivered to producers.</span></p><br /> <p><span style="font-weight: 400;">A train-the-trainer event titled Data Science for Ag Extension Agents proposed to educate agriculture and natural resources (ANR) Extension Agents on current digital agriculture technologies and how they could be used in on-farm investigation. The event was funded by NSF and the Sloan Foundation. Data Science for Ag Extension Agents was held on January 15-16, 2019 at the Lake Wheeler Road Field Laboratory in Raleigh, NC. Approximately 40 total people were present for all or part of the content including registered attendees, speakers, and additional attendees from on-campus departments or commodity groups. Speakers were invited from NC State, Kansas State University, The Ohio State University, and industry. Focus was placed on data generated from agricultural production in both crop and animal production systems and how that data could be properly analyzed to inform producer decision making. Best practices in conducting on-farm investigation and free, open-source data analysis tools were introduced. </span></p><br /> <p><span style="font-weight: 400;">A field day event targeted at research station staff and Extension agents was organized on applying next generation planter technology, guidance, and tractor systems. This workshop was organized with industry partners and is expected to continue for future years. Further training was Created for the North Carolina Annual Extension Conference, along with colleagues, to introduce precision agriculture and small UAS applications.</span></p><br /> <p><span style="font-weight: 400;">Trainee self-assessment indicated that degree of understanding in all target knowledge areas increased. While this training did improve understanding, most knowledge areas were below a score of three out of a four point scale. This assessment indicates that additional training or repeated exposure to the content will be needed on increase advanced understanding. The Extension agents who attended have exceptional knowledge in their focus areas, adding functional knowledge of digital agriculture and data science to their day-to-day activities will require additional training avenues and training reinforcement.</span></p><br /> <h2><span style="font-weight: 400;">University of Florida</span></h2><br /> <p><span style="font-weight: 400;">An automated imaging system was developed to count the number of flowers and fruit in the strawberry field using artificial intelligence. Maps for flower distribution and estimated fruit yield were created to help growers for more efficient harvesting operations. Algorithms for detecting strawberry plant wetness were developed using color and thermal imaging. The results will be used for the Strawberry Advisory System which provides real-time fungicide recommendations to strawberry growers. </span></p><br /> <h2><span style="font-weight: 400;">University of Nebraska</span></h2><br /> <h3><span style="font-weight: 400;">Extension Activities:</span></h3><br /> <h4><span style="font-weight: 400;">Nebraska Digital Ag Workshops</span></h4><br /> <p><span style="font-weight: 400;">In previous years, the UNL Precision Ag team had conducted in-person data management workshops to teach attendees methods for working with digital agriculture datasets. In 2018, we began to migrate these workshops to an online format where attendees could enroll in the self-paced course to learn at their convenience. To date, three of the courses have been migrated to the online system. We have currently had around two dozen individuals register and begin learning via the online environment. The courses are designed to collect impact/knowledge gained data after completion of each module. We hope to begin assessing this information in 2020.</span></p><br /> <p><span style="font-weight: 400;">Two day-long Digital Ag workshops were hosted for students at Highland Community College, Kansas with 20 total attendees (students were not surveyed for impact assessment).</span></p><br /> <h4><span style="font-weight: 400;">Project SENSE (Sensors for Efficient Nitrogen Use and Stewardship of the Environment)</span></h4><br /> <p><span style="font-weight: 400;">The 2019 growing season represented the fourth year of Project SENSE. During the year, we reported on significant project findings from the first three years as we conducted additional field activities. The final project grower/cooperator meeting was held in 2018. Feedback from the group indicated that while adoption of the sensing technology had not occurred, 50% of cooperators that had been involved in two or more years of the project had lowered nitrogen application rates in their fields as a result of working with the project. The primary feedback given was that they had observed much improved nitrogen use efficiency metrics via the sensor-based applications and they were trying to reduce nitrogen use in their operations. Interacting with Project SENSE provided confidence to them that reducing nitrogen rates would not negatively impact their crop yield significantly.</span></p><br /> <h3><span style="font-weight: 400;">Education Activities:</span></h3><br /> <h4><span style="font-weight: 400;">Site-Specific Crop Management (AGEN/AGRO/MSYM 431) 3 Credit Hour Course</span></h4><br /> <p><span style="font-weight: 400;">Student enrollment consisted of 82 agronomy, engineering, and mechanized systems management students in 2019. Students were exposed to a variety of topics related to precision agriculture; 15 computer laboratory exercises were conducted throughout the semester to provide hands-on learning experiences with agricultural data applications.</span></p><br /> <h2><span style="font-weight: 400;">University of Wisconsin-Madison</span></h2><br /> <p><span style="font-weight: 400;">The precision agriculture group at the University of Wisconsin-Madison has been focused on planter research, machinery movement and the impact of wheel traffic, and remote sensing. Two federally funded projects of note were awarded in 2018. An USDA-NRCS Conservation Innovation Grant was awarded to investigate planter technology pertaining to organic no-till practices with the goal of improving emergence and weed control (Dr. B. Luck). We recently concluded our first field season associated with this project and collaborations with industry partners providing down-force, depth control, and firming wheel control systems did impact planter performance and plant emergence. Secondly, a USDA-NIFA Alfalfa and Forage Research Program project was awarded to investigate the effect of wheel traffic on alfalfa yield and persistence (Dr. B. Luck). We have completed our first field season with this project as well. Initial results indicate that compaction has an impact on alfalfa yield and this impact can be as great as 1.5 ton/ac depending on the amount of traffic applied to the plants. Soil compaction was measured and remote sensing data was collected via Unmanned Aerial Vehicle. This data is currently being analyzed. Finally, alfalfa yield prediction using UAV-based hyperspectral imagery and machine learning (Dr. Zhou Zhang)-The UAV-based hyperspectral imaging platform has been developed and become fully operational. Hyperspectral data over alfalfa fields have been successfully collected and pre-processed. Yield prediction model is under-development.</span></p><br /> <p><span style="font-weight: 400;">An area of high interest has been assessing crop quality during corn silage harvest. In 2018, the UW-Madison group release a smart phone application (SilageSnap) that utilizes image processing methods to determine kernel processing score during harvest. Typically, farmers send samples to a feed quality laboratory to make this assessment. By the time results return the crop harvest has been completed. To date, SilageSnap has been downloaded nearly 1,000 times and many results from its use have been shared with the research team. This effort has had an impact on feed quality for dairy farms in Wisconsin and across the United States.</span></p><br /> <p><span style="font-weight: 400;">A new research push for the Wisconsin Precision Agriculture Group has, and will be, industrial hemp production. There is considerable interest in this potentially high value crop. In 2019, we grew 10 acres of industrial hemp for grain production. We assessed different row spacing and combine settings for optimal grain harvest. Results from this preliminary work showed that 15 inch row spacing yield was 2300 lb/ac (± 440 lb/ac) and 30 inch row spacing yielded 1600 lb/ac (± 170 lb/ac). At harvest, threshing the material went well, but variations in plant height had an effect on cutting the crop consistently. Losses were realized at the header and through the machine. We intend to continue this research in 2020 to better understand how to manage this crop. Additionally, site-specific management trials and remote sensing data collection will be conducted in 2020 with the goal of optimizing industrial hemp grain production.</span></p><br /> <p><span style="font-weight: 400;">Precision Agriculture extension efforts at the University of Wisconsin-Madison have remained very popular across the state. Multiple county meetings were attended with subject matters ranging from Unmanned Aerial Vehicles and remote sensing, digital agriculture, and planting technology for optimal seeding and emergence. Two international extension opportunities were also attended. Dr. Luck was invited to Temuco, Chile to speak on forage harvest technology and the future of agriculture and Sao Paulo, Brazil for a similar program. This reporting year yielded one peer-reviewed extension publication (see publications section).</span></p><br /> <h2><span style="font-weight: 400;">Washington State University </span></h2><br /> <p><span style="font-weight: 400;">Precision chemical application is one of the major focus of WSU team. An optimized solid set canopy delivery system (SSCDS) was evaluated with different emitters/microsprayers– configurations in a high-density apple orchard and modified vertical shoot position (VSP) trained grapevines. To achieve uniform spray application over longer spray lengths, team has built a reservoir sub-system that integrates into SSCDS. We also have developed and tested the prototype automation sub-system to operate SSCDS. During 2018 season trials, the airblast sprayer and the SSCDS configurations tested in modified VSP had statistically similar within-canopy spray deposition. Drift losses to air were about 900 and 390 times higher for airblast sprayer compared to the studied SSCDS configurations at 6 ft. and 12 ft. downwind, respectively.</span></p><br /> <p><span style="font-weight: 400;"> To reduce chemical use in tree fruit production, WSU team is also exploring alternative pest management technologies. A laboratory–scale application technology unit was developed to apply horticultural oil (HO)–based thermotherapy. Using such unit, experiments were conducted to have spray treatments including four variables namely heat–condition (i.e., heat and no–heat), HO concentration (i.e., 0.5 and 1.0 %), two nozzle types and application pressure. Overall, HO combined with thermotherapy caused a rapid kill of pear psylla (mortality: 74.4±3.1 %).</span></p><br /> <p><span style="font-weight: 400;"> WA team also worked on fruit harvesting and handling technology using a 12-armed robot. A fruit orientation estimation and obstacle detection and avoidance capability have also been developed for the robot. The study is showing promising results in terms of harvesting speed and efficiency for commercial adoption. Another approach, targeted shake-and-catch system, was also evaluated for apple harvesting. Field tests showed that fruit detachment and collection efficiency increased with shorter branch length on similar size branches, and could reach 90% or more in modern, formally trained orchards for a number of varieties like Pink Lady and Scifresh. The technique achieved a fruit damage rate of about 10% for some varieties including Pink Lady, Scifresh and Pacific Rose. This approach showed promise for faster and potentially low cost harvesting of apples for fresh market consumption. However, the method tended to show varietal dependence with Pink Lady, and Jazz showing higher removal efficiency and better quality fruit while varieties like gala and honey crisp suffering from either low removal efficiency, low fruit quality or both. </span></p><br /> <p><span style="font-weight: 400;"> WSU team also worked on a robotic weeding system for vegetable crops with a self levelling system. The system was able to distinguish carrots and onions from different types of weeds with more than 99% accuracy and the weeds could be sprayed with a precision of 2 mm when the robot was travelling on an uneven field terrain. Similarly, the team is working on developing an automated system for green shoot thinning in vineyards. A deep-learning-based machine vision system was developed to detect shoots and cordon of the vines during early growing season and a trajectory fitting model was proposed to represent cordon position and orientation. A trajectory fitting model with a 6th degree polynomial was found to fit about 80% of cordon trajectories with an R-square value of 0.98. WSU team also has started working on a SMART IRRIGATION project, where internet of things is being used to collect various types of data including soil, environment and canopy parameters/maps in space and time and a big data analytics technique is being developed to understand the inherent relationships and patterns in the data, which is expected to improve the assessment of plant water needs and implementation of a decision support system. </span></p><br /> <p><span style="font-weight: 400;"> WSU team is also working on the development of Internet-of-Things enabled Crop Physiology Sensing System (CPSS) for tree fruit crop loss management with initial focus on apple sunburn management. In 2018-19, CPSSS nodes that encompass a thermal-RGB imager integrated with a single board computer for data acquisition and on-board real-time data analytics were developed and tested in commercial apple orchard. Such nodes are capable of apple fruit surface temperature monitoring and actuation of automated SSCDS for evaporative cooling of apple orchard block. </span></p><br /> <h3><span style="font-weight: 400;">WSU Activities</span></h3><br /> <p><span style="font-weight: 400;"> A 12-armed full scale robotic harvesting system (including the desired machine vision system) was developed (in collaboration with FFRobotics) and was evaluated in commercial orchards.</span></p><br /> <p><span style="font-weight: 400;"> A multi-layer self-propelled shake and catch harvesting platform was developed and further evaluated in 2018 harvest season.</span></p><br /> <p><span style="font-weight: 400;"> A robotic prototype was evaluated for automated bin movement in orchards. The prototype with four wheel steering system used RTK GPS and laser sensing systems to navigate in orchards.</span></p><br /> <p><span style="font-weight: 400;"> In another project, WSU team designed and fabricated a research prototype of self-propelled robotic weeding machine for vegetable production.</span></p><br /> <p><span style="font-weight: 400;"> Another activity was the investigation of mechanized red raspberry cane bundling and tying. A cane tying mechanism has been designed, fabricated, and then evaluated in field operation.</span></p><br /> <p><span style="font-weight: 400;"> A SMART IRRIGATION project was initiated to optimize water use in wine grape using big data analytics.</span></p><br /> <p><span style="font-weight: 400;"> A machine vision system was developed for automating green shoot thinning in wine grapes. </span></p><br /> <p><span style="font-weight: 400;"> WSU team also works on sensing and automation technologies with UASs. One specific project we worked on was to use UASs to deter birds from fruit crops such as wine grapes, blueberries and apples. </span></p><br /> <p><span style="font-weight: 400;">Optimization of SSCDS configurations in a high-density apple orchard and modified vertical shoot position trained grapevines.</span></p><br /> <p><span style="font-weight: 400;">Development and evaluation of prototype horticultural oil thermotherapy system for pear psylla management.</span></p><br /> <p><span style="font-weight: 400;">Development of Internet-of-Things enabled Crop Physiology Sensing System for tree fruit crop loss management with initial focus on apple sunburn management.</span></p><br /> <p><span style="font-weight: 400;">Synthesis of a novel Cellulose NanoCrystals (CNC) based dispersion that can be applied as a spray agent on tree fruit buds to prevent the frost damage. </span></p>Publications
<p><span style="font-weight: 400;">Barnes, E.M., Hake, K., Griffin, T.W., Rains, G.C., Maja, J.M.J., Thomasson, J.A., Griffin, J.A., Pelletier, M.G., Kimura, E., Morgan, G., Devine, J., Ibendahl, G. and Ayre, B.G. 2019. Initial Possibilities for Robotic Cotton Harvest. Beltwide Cotton Conference, New Orleans, LA</span></p><br /> <p><span style="font-weight: 400;">Griffin, T.W., Ibendahl, G., Regmi, M., Barnes, E., Devine, J., Cullop, J., Griffin, T.G. 2019. Optimal Robotic Utilization for Cotton Production. Beltwide Cotton Conference, New Orleans, LA. January 8, 2019.</span></p><br /> <p><span style="font-weight: 400;">Barnes, E.M., Ryan Kurtz, Jesse Daystar, John Wanjura, Jason Ward, Bobby Hardin, Kendall Kirk, Wes Porter. (2019). Capturing More Value from Cotton Data. Cotton Cultivated. </span><a href="https://cottoncultivated.cottoninc.com/research_reports/capturing-value-from-cotton-data/"><span style="font-weight: 400;">https://cottoncultivated.cottoninc.com/research_reports/capturing-value-from-cotton-data/</span></a></p><br /> <p><span style="font-weight: 400;">Bhusal, S., K. Khanal, S. Goel, M. Taylor and M. Karkee. 2019. Bird Deterrence in a Vineyard using an Unmanned Aerial System (UAS). Transactions of the ASABE; 62(2): 561-569.</span></p><br /> <p><span style="font-weight: 400;">Chakraborty, M., L. R. Khot, S. Sankaran and P. Jacoby. 2019. Evaluation of mobile 3D light detection and ranging based canopy mapping system for tree fruit crops. Computers and Electronics in Agriculture,158: 284-293. </span><a href="https://doi.org/10.1016/j.compag.2019.02.012"><span style="font-weight: 400;">https://doi.org/10.1016/j.compag.2019.02.012</span></a></p><br /> <p><span style="font-weight: 400;">Chakraborty, M., L. R. Khot and R. T. Peters. 2019. Assessing suitability of modified center pivot irrigation systems in corn production using low altitude aerial imaging techniques. Information Processing in Agriculture, </span><a href="https://doi.org/10.1016/j.inpa.2019.06.001"><span style="font-weight: 400;">https://doi.org/10.1016/j.inpa.2019.06.001</span></a></p><br /> <p><span style="font-weight: 400;">Chandel, A., L. R. Khot, Y. Osroosh and R. T. Peters. 2018. Thermal-RGB imager derived in-field apple surface temperature estimates for sunburn management. Agricultural and Forest Meteorology, 253-254: 132-140. </span><a href="https://doi.org/10.1016/j.agrformet.2018.02.013"><span style="font-weight: 400;">https://doi.org/10.1016/j.agrformet.2018.02.013</span></a></p><br /> <p><span style="font-weight: 400;">Chen, Y., W. S. Lee, H. Gan, N. Peres, C. Fraisse, Y. Zhang, and Y. He. 2019. Strawberry yield prediction based on a deep neural network using high-resolution aerial orthoimages. Remote Sensing, 11: 1584. Doi:10.3390/rs11131584. </span></p><br /> <p><span style="font-weight: 400;">Cordero, E., Longchamps, L., Sacco, D., and Khosla, R. 2019. Spatial management strategies for nitrogen in maize production based on soil and crop data. Science of the Total Environment Journal. STOTEN.</span></p><br /> <p><span style="font-weight: 400;">Cullop, J., Ibendahl, G., Shockley, J., Barnes, E., Devine, J., & Griffin, T.W. 2018. Economics of Swarm Bot Profitability for Cotton Harvesting. In Proceedings of the 14th International Conference on Precision Agriculture (unpaginated, online). Monticello, IL: International Society of Precision Agriculture.</span></p><br /> <p><span style="font-weight: 400;">De Lara, A, R. Khosla, L. Longchamps. 2019. Soil water content and high resolution imagery: Maize yield. J. Agron. </span></p><br /> <p><span style="font-weight: 400;">Drewry, J. L., B. D. Luck, R. M. Willett, E. M. C. Rocha, and J. D. Harmon, 2018. Assessing particle size distribution of harvested and processed corn silage via image processing techniques. Computers and Electronics in Agriculture160: 144-152. https://doi.org/10.1016/j.compag.2019.03.020</span></p><br /> <p><span style="font-weight: 400;">Drewry, J. L., J. M. Shutske, D. Trechter, B. D. Luck, L. Pitman. 2019. Assessment of digital technology adoption and access barriers among crop, dairy and livestock producers in Wisconsin. Computers and Electronics in Agriculture 165: 104960. </span><a href="https://doi.org/10.1016/j.compag.2019.104960"><span style="font-weight: 400;">https://doi.org/10.1016/j.compag.2019.104960</span></a></p><br /> <p><span style="font-weight: 400;">Ellixson, A., Griffin, T.W., Ferrell, S.L., and Goeringer, L.P. 2019. Legal and Economic Implications of Farm Data: Ownership and Possible Protections. Drake Journal of Agricultural Law. 24(2)</span></p><br /> <p><span style="font-weight: 400;">Ferrell, S.L. and Griffin, T.W. 2018. Managing Farm Risk Using Big Data: A guide to understanding the opportunities and challenges of agricultural data for your farm. Handbook sponsored by USDA NIFA RME </span><a href="http://agecon.okstate.edu/farmdata/"><span style="font-weight: 400;">http://agecon.okstate.edu/farmdata/</span></a></p><br /> <p><span style="font-weight: 400;">Finkenbiner, C., T.E. Franz, J. Gibson, D.M. Heeren, and J.D. Luck. 2018. Integration of hydrogeophysical datasets and empirical orthogonal functions for improved irrigation water management. Precision Agric. https://doi.org/10.1007/s11119-018-9582-5 (Contribution 10%)</span></p><br /> <p><span style="font-weight: 400;">Griffin, T.W., and Yeager, E.A. 2019. How quickly do farmers adopt technology? A duration analysis. Precision agriculture ’19. Ed. J.V. Stafford. 12th European Conference on Precision Agriculture. pp 843-849 </span><a href="https://doi.org/10.3920/978-90-8686-888-9_104"><span style="font-weight: 400;">https://doi.org/10.3920/978-90-8686-888-9_104</span></a></p><br /> <p><span style="font-weight: 400;">Griffin, T.W., Yeager, E.A., and Ibendahl, G. Adoption of Precision Agriculture Technology. International Farm Management Congress</span></p><br /> <p><span style="font-weight: 400;">Griffin, T.W., Shanoyan, A., Yeager, B.A., and Ibendahl, G. 2019. Agricultural Technology Adoption Path of Kansas Farms AIM American Society of Agricultural and Biological Engineers Boston, MA July 7-10, 2019</span></p><br /> <p><span style="font-weight: 400;">Griffin, T.W., Ibendahl, G., Barnes, E., and Devine, J. 2019. Optimal utilization of autonomous robotics for cotton harvest: how slow can we go? AIM American Society of Agricultural and Biological Engineers Boston, MA July 7-10, 2019</span></p><br /> <p><span style="font-weight: 400;">Griffin, T.W., Ibendahl, G., Yeager, E.A., and Shanoyan, A. 2019. Digital Agriculture as a Risk Management Strategy. National Farm Business Management Conference aka Triennial aka NAFBAS. Sheboygan, WI. June 9-13, 2019.</span></p><br /> <p><span style="font-weight: 400;">Griffin, T.W., Shockley, J., and Mark, T.B. 2018. Economics of Precision Farming. In D.K. Shannon, D.E. Clay, and N.R. Kitchens (Eds.) Precision Agriculture Basics. USDA. American Society of Agronomy, Crop Science Society of America, and Soil Science Society of America.</span></p><br /> <p><span style="font-weight: 400;">Griffin, T.W. and Yeager, E.A. 2018. Adoption of Precision Agriculture Technology: An Analysis of Kansas Farms. agri benchmark Cash Crop Conference 2018. Beijing China. June 2018.</span></p><br /> <p><span style="font-weight: 400;">Harris, K.D., and Griffin, T.W. 2019. The Old “Block” and Chain: How Farm Data Will Be Used on the Blockchain. Kansas State University Department of Agricultural Economics AgManager. July 18, 2019. <a href="https://www.agmanager.info/machinery/precision-agriculture/old-%E2%80%9Cblock%E2%80%9D-and-chain-how-farm-data-will-be-used-blockchain/"><span style="font-weight: 400;">https://www.agmanager.info/machinery/precision-agriculture/old-%E2%80%9Cblock%E2%80%9D-and-chain-how-farm-data-will-be-used-blockchain/</span></a></span></p><br /> <p><span style="font-weight: 400;">He, L., X. Zhang, Y. Ye, M. Karkee, and Q. Zhang. 2019. Effect of Shaking Location and Duration on Mechanical Harvesting of Fresh Market Apples. Applied Engineering in Agriculture; 35(2): 175-183.</span></p><br /> <p><span style="font-weight: 400;">Hohimer, C. J., H. Wang, S. Bhusal, J. Miller, C. Mo, M. Karkee. 2019. Design and field evaluation of a robot apple harvesting system with 3D printed soft-robotic end-effector. Transactions of the ASABE; 62(2): 405-414.</span></p><br /> <p><span style="font-weight: 400;">Karkee, M., J. Gordón, B. Sallto and M. Whiting, Optimizing fruit production efficiencies via mechanization. 2019. In Achieving sustainable cultivation of temperate zone tree fruits and berries, Volume 1 - Physiology, genetics and cultivation (Editor: Dr Greg Lang). Burleigh Dodds Science Publishing.</span></p><br /> <p><span style="font-weight: 400;">Khanal, K., S. Bhusal, M. Karkee, P. Scharf, and Qin Zhang. 2019. Design of Improved and Semi-Automated Red Raspberry Cane Bundling and Tying Machine Based on the Field Evaluation Results. Transactions of the ASABE. 62(3): 821-829.</span></p><br /> <p><span style="font-weight: 400;">Li, J., Shi, Y., Veeranampalayam-Sivakumar, A. N., & Schachtman, D. P. (2018). Elucidating sorghum biomass, nitrogen and chlorophyll contents with spectral and morphological traits derived from unmanned aircraft system. Frontiers in plant science, 9, 1406.</span></p><br /> <p><span style="font-weight: 400;">Lin, P., W. S. Lee, Y. M. Chen, N. Peres, and C. Fraisse. 2019. A deep-level region-based visual representation architecture for detecting strawberry flowers in an outdoor field. Precision Agriculture. Published online: 07 June 2019. </span><a href="https://doi.org/10.1007/s11119-019-09673-7"><span style="font-weight: 400;">https://doi.org/10.1007/s11119-019-09673-7</span></a><span style="font-weight: 400;">.</span></p><br /> <p><span style="font-weight: 400;">Luck, B. D., R. Willett, J. L. Drewry, L. Ferraretto. Monitoring Kernel Processing During Harvest. University of Wisconsin Division of Extension Publication No. A4174. </span><a href="https://learningstore.extension.wisc.edu/products/kernel-monitoring-processing-during-harvest?_pos=1&_sid=6963f54c3&_ss=r"><span style="font-weight: 400;">https://learningstore.extension.wisc.edu/products/kernel-monitoring-processing-during-harvest?_pos=1&_sid=6963f54c3&_ss=r</span></a></p><br /> <p><span style="font-weight: 400;">Miller, K.A., J.D. Luck, D.M. Heeren, T.H. Lo, D.L. Martin, and J.B. Barker. 2018. A geospatial variable rate irrigation control scenario evaluation methodology based on mining root zone available water capacity. Precision Agric. 19(4): 666-683. (Contribution 40%)</span></p><br /> <p><span style="font-weight: 400;">Miller, N.J., Griffin, T.W., Ciampitti, I., and Sharda, A. 2019. Farm Adoption of Embodied Knowledge and Information Intensive Precision Agriculture Technology Bundles. Precision Agriculture 20(2):348-361 </span><a href="https://rdcu.be/6LvT"><span style="font-weight: 400;">https://rdcu.be/6LvT</span></a></p><br /> <p><span style="font-weight: 400;">Miller, N.J., Griffin, T.W., Goeringer, L.P., Ellixson, A. and Shanoyan, A. 2018. Estimating Value, Damages, and Remedies when Farm Data are Misappropriated. Choices. 4th Quarter 2018 33(4)</span></p><br /> <p><span style="font-weight: 400;">Osroosh, Y., L. R. Khot and R. T. Peters. 2019. Detecting fruit surface wetness using a custom-built low-resolution thermal-RGB imager. Computers and Electronics in Agriculture, 157: 509–517. </span><a href="https://doi.org/10.1016/j.compag.2019.01.023"><span style="font-weight: 400;">https://doi.org/10.1016/j.compag.2019.01.023</span></a></p><br /> <p><span style="font-weight: 400;">Pena Quinones, A. J., M. Keller, M. R. Salazar-Gutierrez, L. R. Khot and G. Hoogenboom. 2019. Comparison between grapevine tissue temperature and air temperature. Scientia Horticulturae, 247: 407–420. </span><a href="https://doi.org/10.1016/j.scienta.2018.12.032"><span style="font-weight: 400;">https://doi.org/10.1016/j.scienta.2018.12.032</span></a></p><br /> <p><span style="font-weight: 400;">Ranjan, R., G. Shi, R. Sinha, L. R. Khot, G.–A. Hoheisel and M. Grieshop. 2019. Automated solid set canopy delivery system for large scale spray applications in perennial tree–fruit crops. Transactions of the ASABE, In Press. https://doi.org/10.13031/trans.13258 </span></p><br /> <p><span style="font-weight: 400;">Ranjan, R., A. Chandel, L. R. Khot, H. Bahlol, J. Zhou, R. Boydston and P. Miklas. 2019. Irrigated pinto bean crop stress and yield assessment using ground based low altitude remote sensing technology. Information Processing in Agriculture, </span><a href="https://doi.org/10.1016/j.inpa.2019.01.005"><span style="font-weight: 400;">https://doi.org/10.1016/j.inpa.2019.01.005</span></a></p><br /> <p><span style="font-weight: 400;">Rohrer, R.A., S.K. Pitla, J.D. Luck, and R.M. Hoy. 2018. Evaluation of the accuracy of machine reported CAN data for engine torque and speed. Trans. ASABE 61(5): 1547-1557 (Contribution 20%)</span></p><br /> <p><span style="font-weight: 400;">Santiago, W. E., N. J. Leite, B. J. Teruel, M. Karkee, C. A.M. Azania. 2019. Evaluation of bag-of-features (BoF) technique for weed management in sugarcane production. Australian Journal Crop of Science. Accepted.</span></p><br /> <p><span style="font-weight: 400;">Sharda, A., G. Hoheisel, M. Karkee, and Q. Zhang. 2019. Design and evaluation of solid set canopy delivery system for spray application in high-density apple orchards. Transactions of the ASABE.</span></p><br /> <p><span style="font-weight: 400;">Siegfried, J, R. Khosla, L. Longchamps. 2018. Multispectral satellite imagery to quantify in-field soil moisture variability. J. Soil Water Conserv. doi:10.2489/jswc.74.1.33</span></p><br /> <p><span style="font-weight: 400;">Sinha, R., L. R. Khot, A. Rathnayake, Z. Gao and N. Rayapati. 2019. Visible−near infrared spectroradiometry−based detection of grapevine leafroll−associated virus 3 in a red−fruited wine grape cultivar. Computers and Electronics in Agriculture, 162: 165-173. https://doi.org/10.1016/j.compag.2019.04.008 </span></p><br /> <p><span style="font-weight: 400;">Sinha, R., L. R. Khot, G.–A. Hoheisel, M. Grieshop and H. Y. Bahlol. 2019. Feasibility of a solid set canopy delivery system for efficient agrochemical delivery in vertical shoot positioning trained vineyards. Biosystems Engineering, 179: 59-70. https://doi.org/10.1016/j.biosystemseng.2018.12.011 </span></p><br /> <p><span style="font-weight: 400;">Sinha, R., R. Ranjan, G. Shi, G.-A. Hoheisel, M. Grieshop and L. R. Khot. 2019. Solid set canopy delivery system for efficient agrochemical delivery in modern architecture apple and grapevine canopies. Acta Horticulturae, Accepted, In Press.</span></p><br /> <p><span style="font-weight: 400;">Thompson, L.J., B.R. Krienke, R.B. Ferguson, and J.D. Luck. 360-degree Video for Immersive Learner Engagement. J. Extension [On-line], 53(4) Article 5TOT2. Available at: https://joe.org/joe/2018september/pdf/JOE_v56_5tt2.pdf (Contribution 20%)</span></p><br /> <p><span style="font-weight: 400;">Lv, G. Li, J.A. Benediktsson, Z. Zhang, and J. Yan, 2019. Training sample refining method using an adaptive neighbor to improve the classification performance of very high-spatial resolution remote sensing images. Journal of Applied Remote Sensing, vol. 13, no. 3, 2019.</span></p><br /> <p><span style="font-weight: 400;">Zhang, Q., M. Karkee, A. Tabb; The Use of Agricultural Robots in Orchard Management. In Robotics and Automation for a More Sustainable Agriculture (Editor: John Billingsley); rXiv preprint arXiv:1907.13114 (2019).</span></p><br /> <p><span style="font-weight: 400;">Zhang, Y. Jin, B. Chen, and P. Brown. California Almond Yield Prediction at the Orchard Level with a Machine Learning Approach. Frontiers in Plant Science, 10:809, 2019.</span></p><br /> <p><span style="font-weight: 400;">Zhao, R., Z. Shi, Z. Zou, and Z. Zhang. Ensemble-Based Cascaded Constrained Energy Minimization for Hyperspectral Target Detection. Remote Sensing, vol. 11, no. 11, 2019. </span></p>Impact Statements
- Image analysis work, at the University of Wisconsin-Madison, yielded a smart phone application that allows producers to assess Kernel Processing Score during harvest. Nearly 1,000 downloads of this app have shown its impact and results reporting to the research team also indicate its continued use.